Nvidia announces Blackwell Ultra B300 —1.5X faster than B200 with 288GB HBM3e and 15 PFLOPS dense FP4
Even faster AI data center GPUs are incoming.
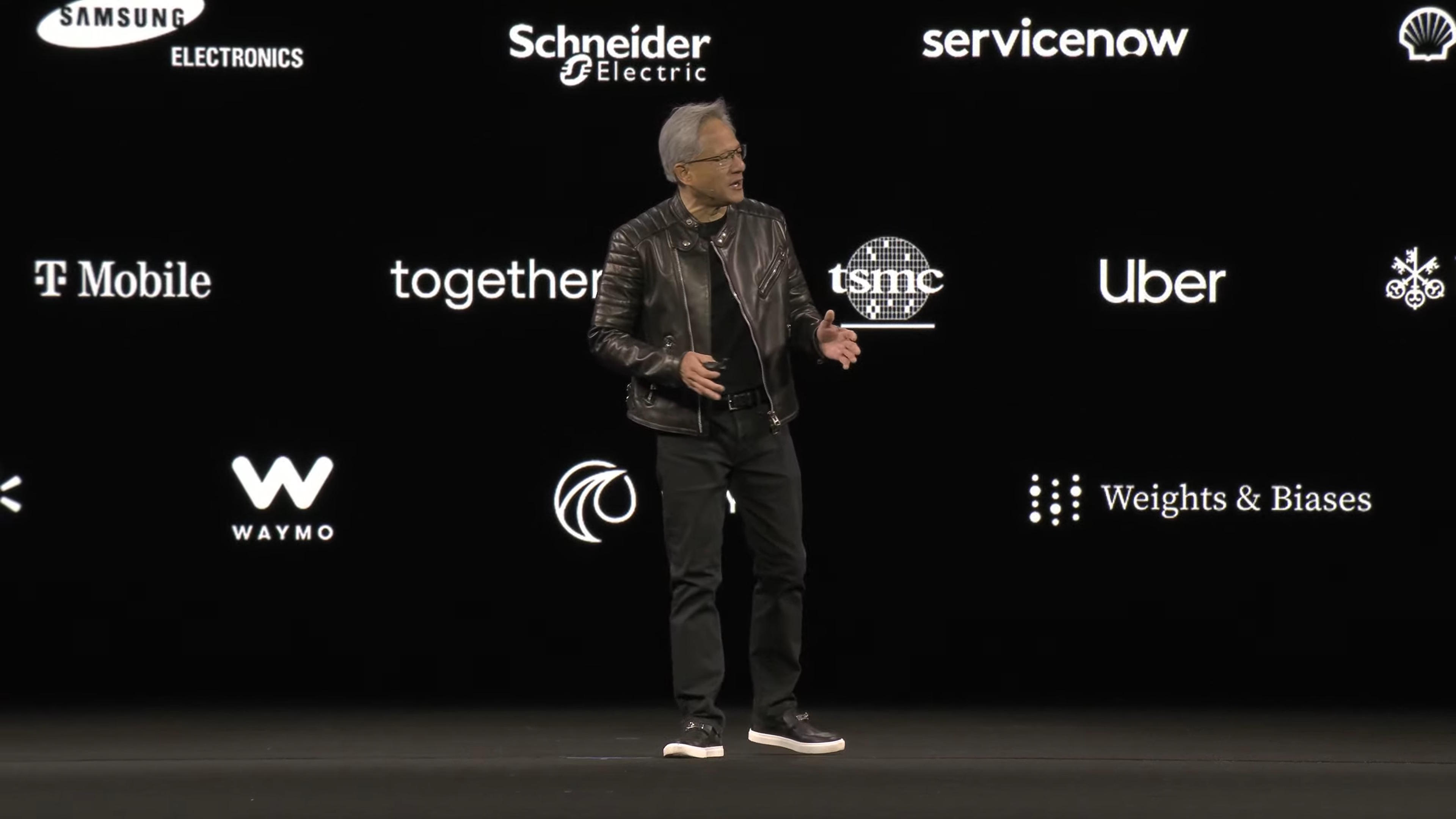
The Nvidia Blackwell Ultra B300 data center GPU was announced today during CEO Jensen Huang's keynote at GTC 2025 in San Jose, CA. Offering 50% more memory and FP4 compute than the existing B200 solution, it raises the stakes in the race to faster and more capable AI models yet again. Nvidia says it's "built for the age of reasoning," referencing more sophisticated AI LLMs like DeepSeek R1 that do more than just regurgitate previously digested information.
Naturally, Blackwell Ultra B300 isn't just about a single GPU. Along with the base B300 building block, there will be new B300 NVL16 server rack solutions, a GB300 DGX Station, and GB300 NV72L full rack solutions. Put eight NV72L racks together, and you get the full Blackwell Ultra DGX SuperPOD: 288 Grace CPUs, 576 Blackwell Utlra GPUs, 300TB of HBM3e memory, and 11.5 ExaFLOPS of FP4. These can be linked together in supercomputer solutions that Nvidia classifies as "AI factories."
While Nvidia says that Blackwell Ultra will have 1.5X more dense FP4 compute, what isn't clear is whether other compute have scaled similarly. We would expect that to be the case, but it's possible Nvidia has done more than simply enabling more SMs, boosting clocks, and increasing the capacity of the HBM3e stacks. Clocks may be slightly slower in FP8 or FP16 modes, for example. But here are the core specs that we have, with some inference of other data (indicated by question marks).
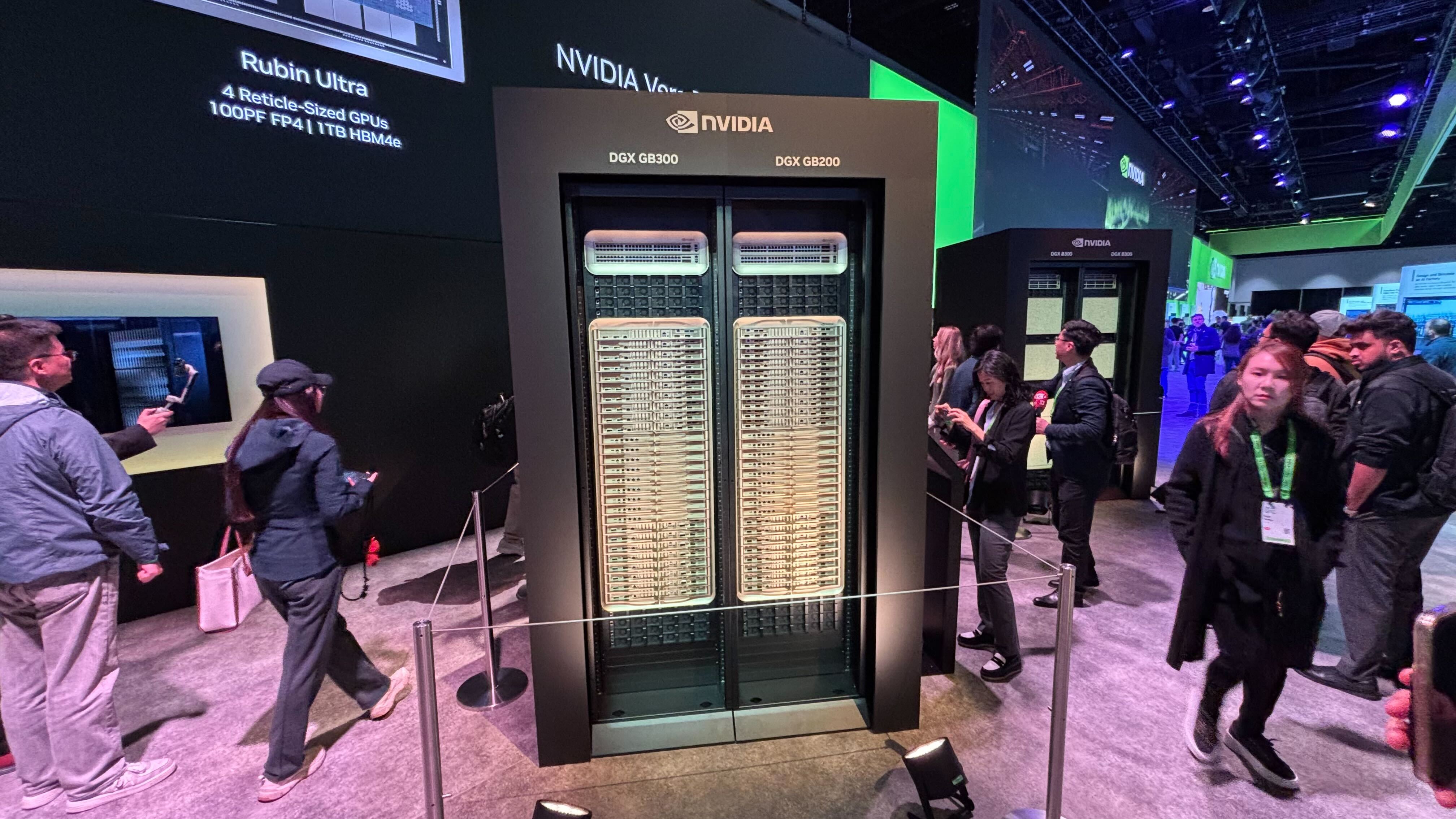
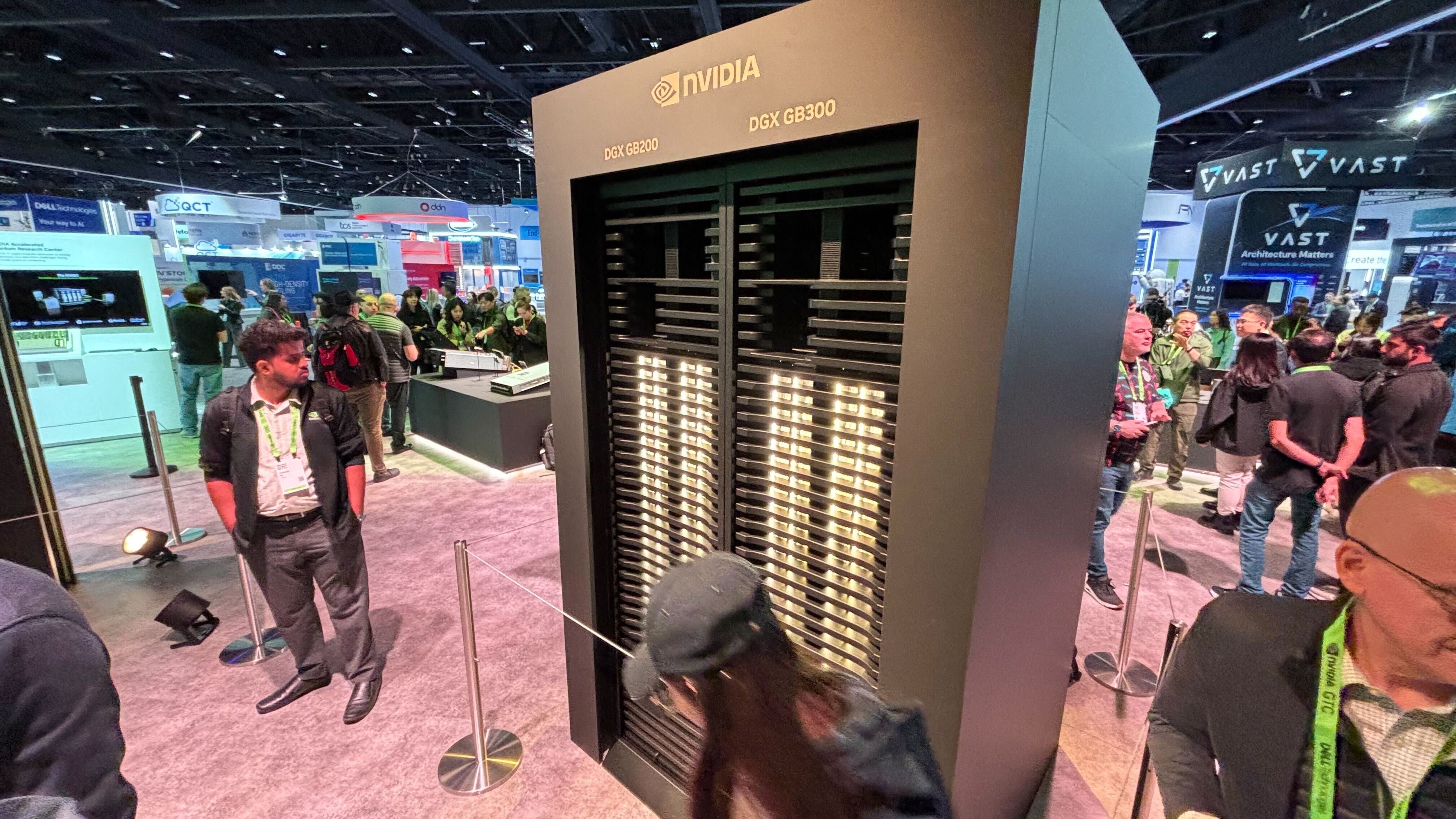
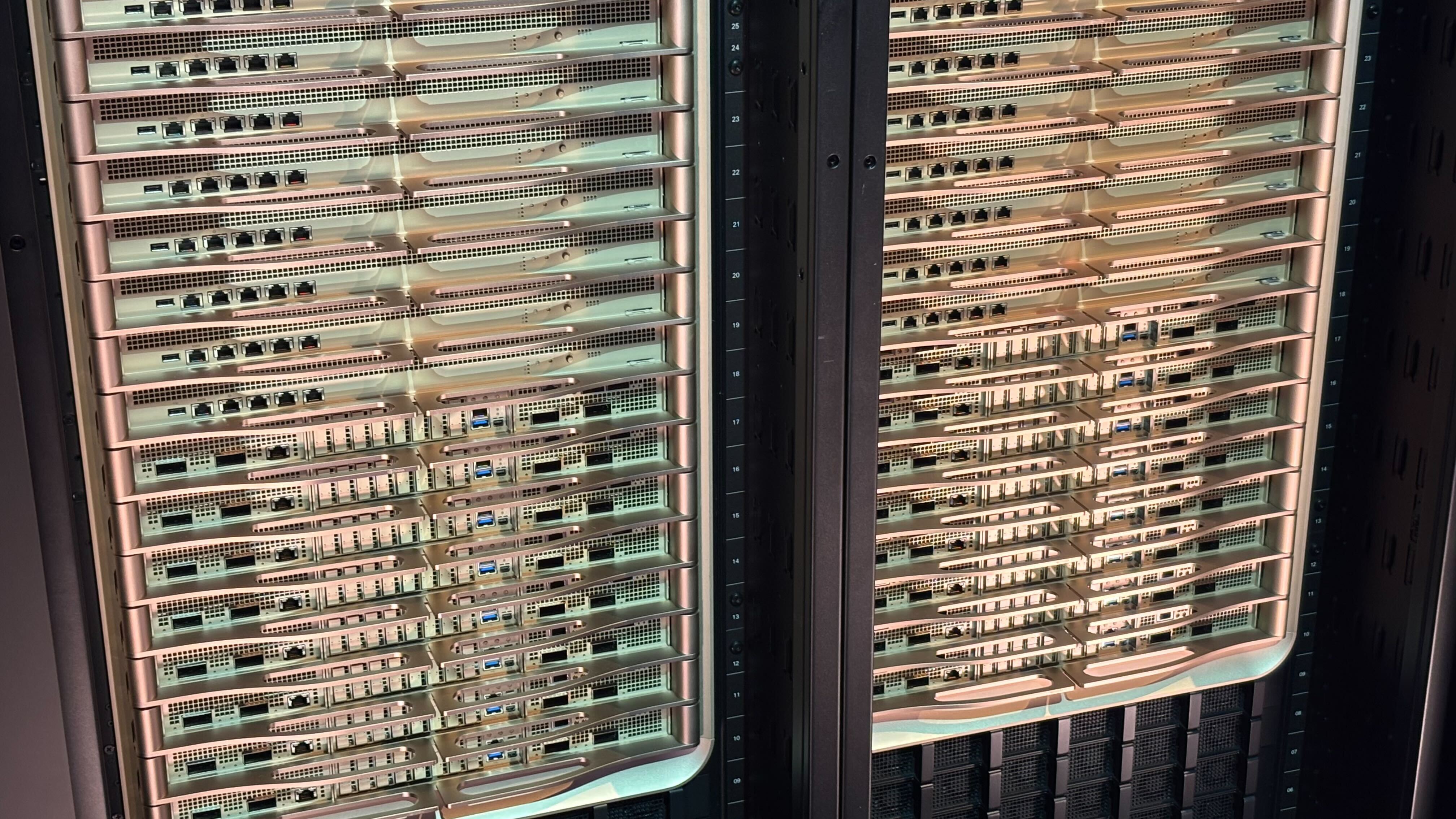
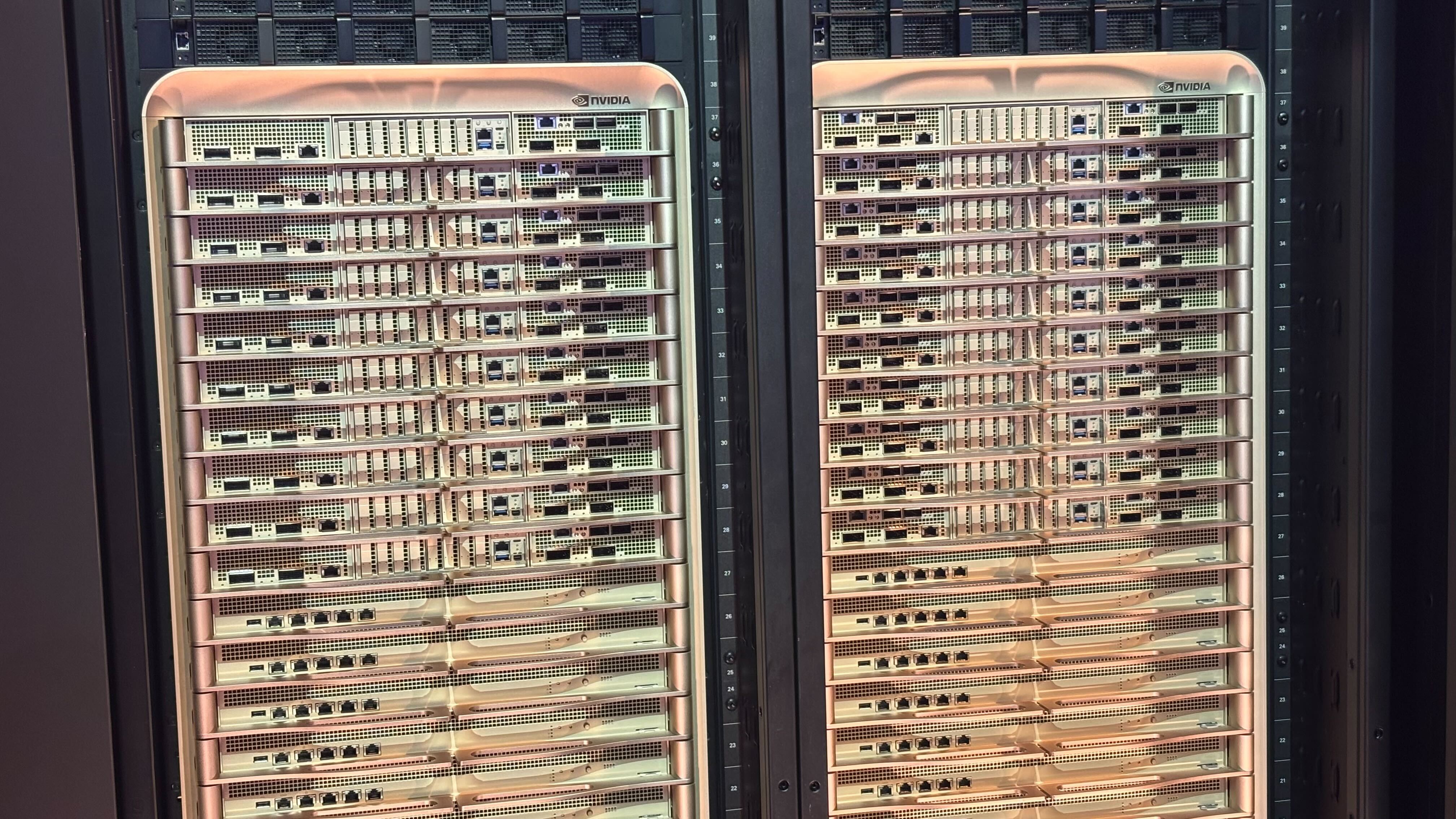
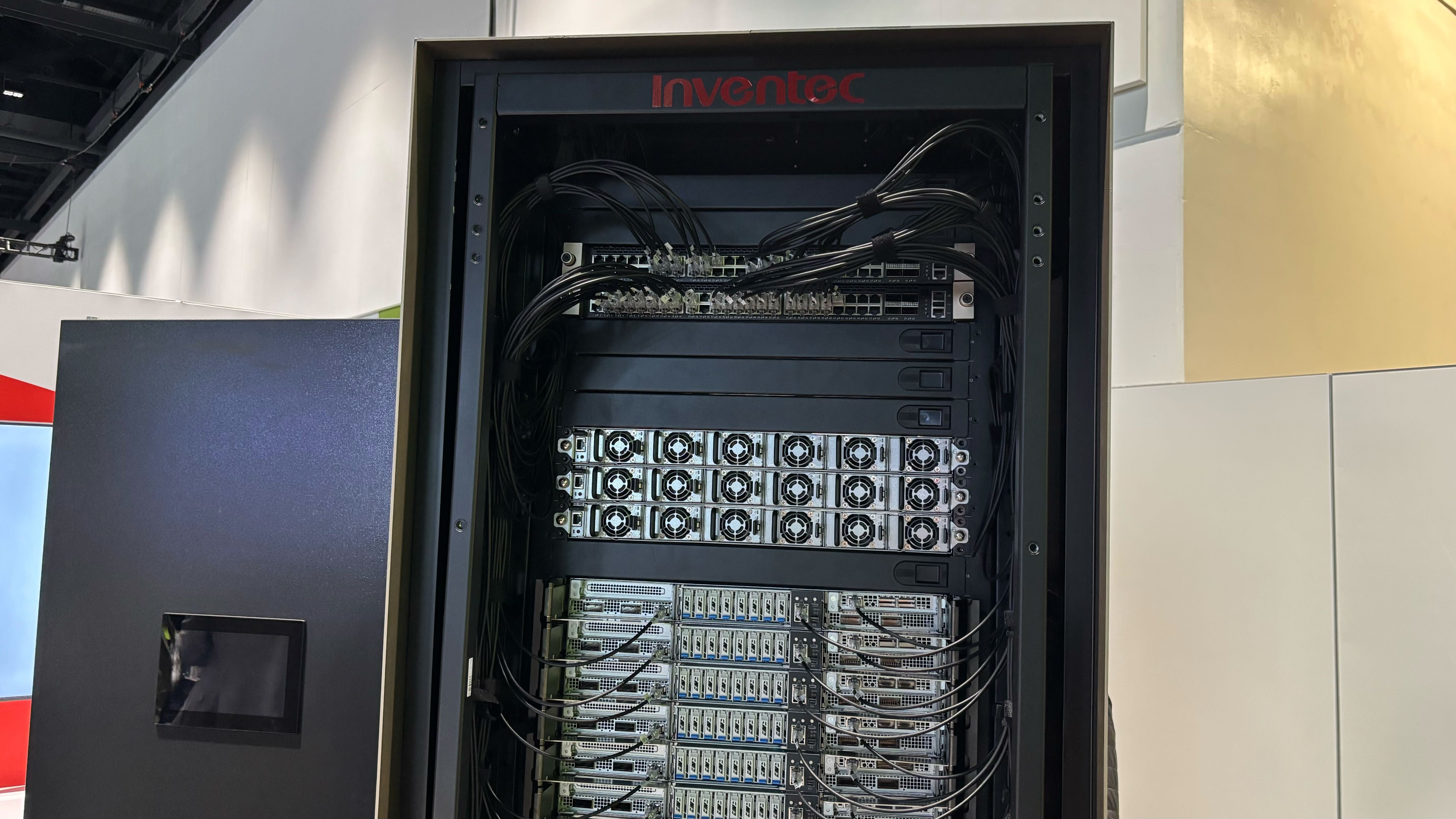
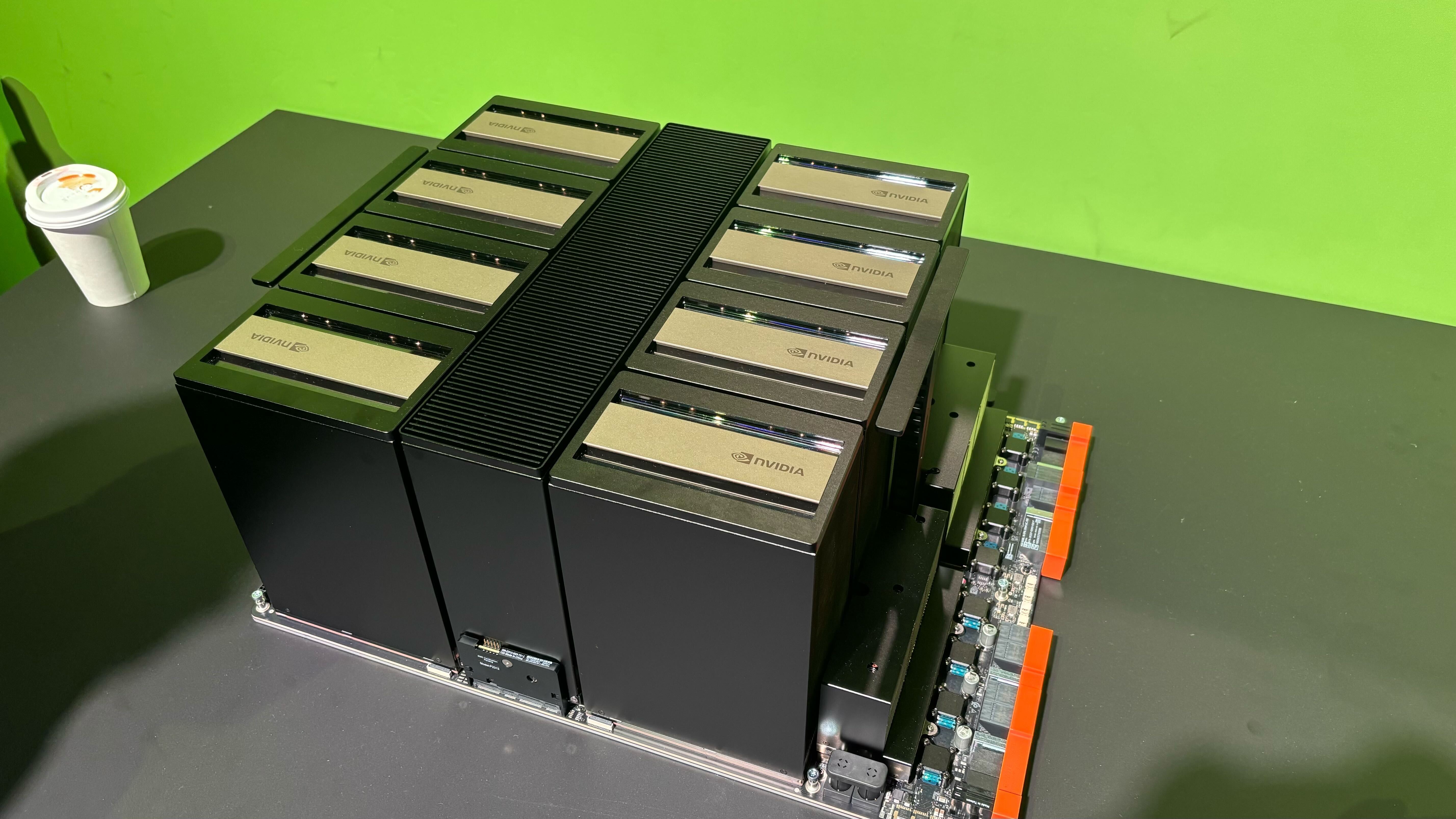
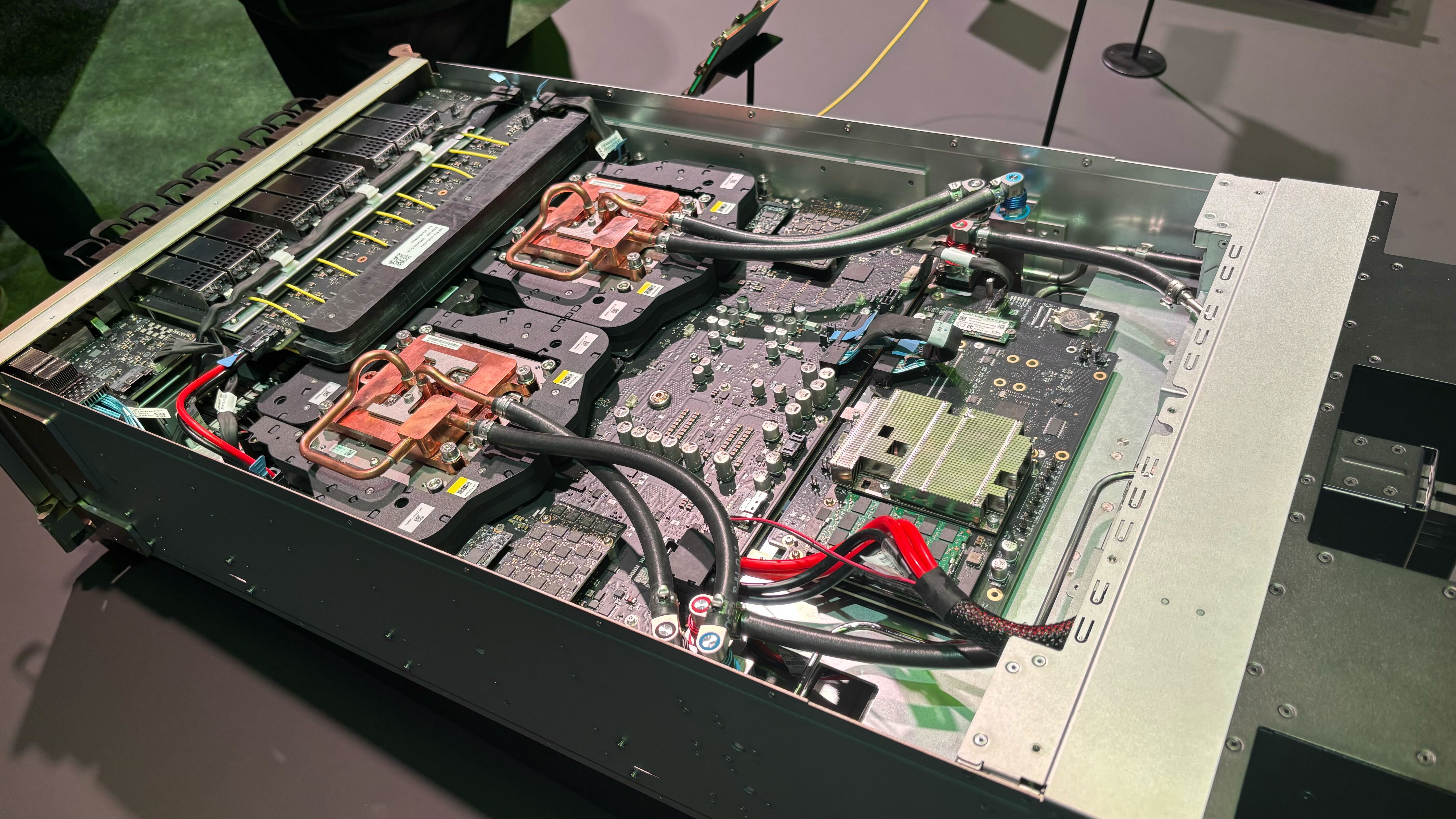
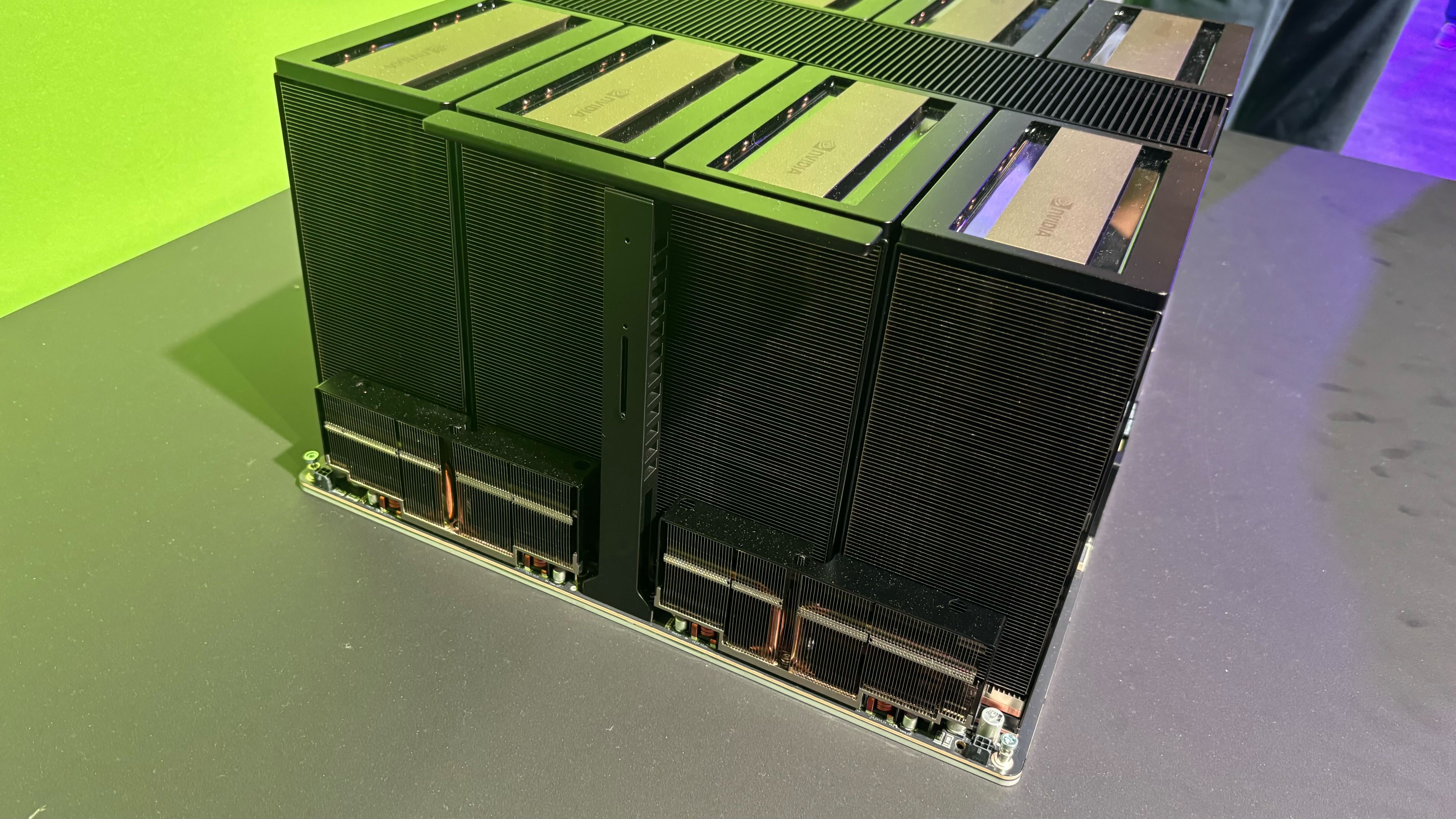
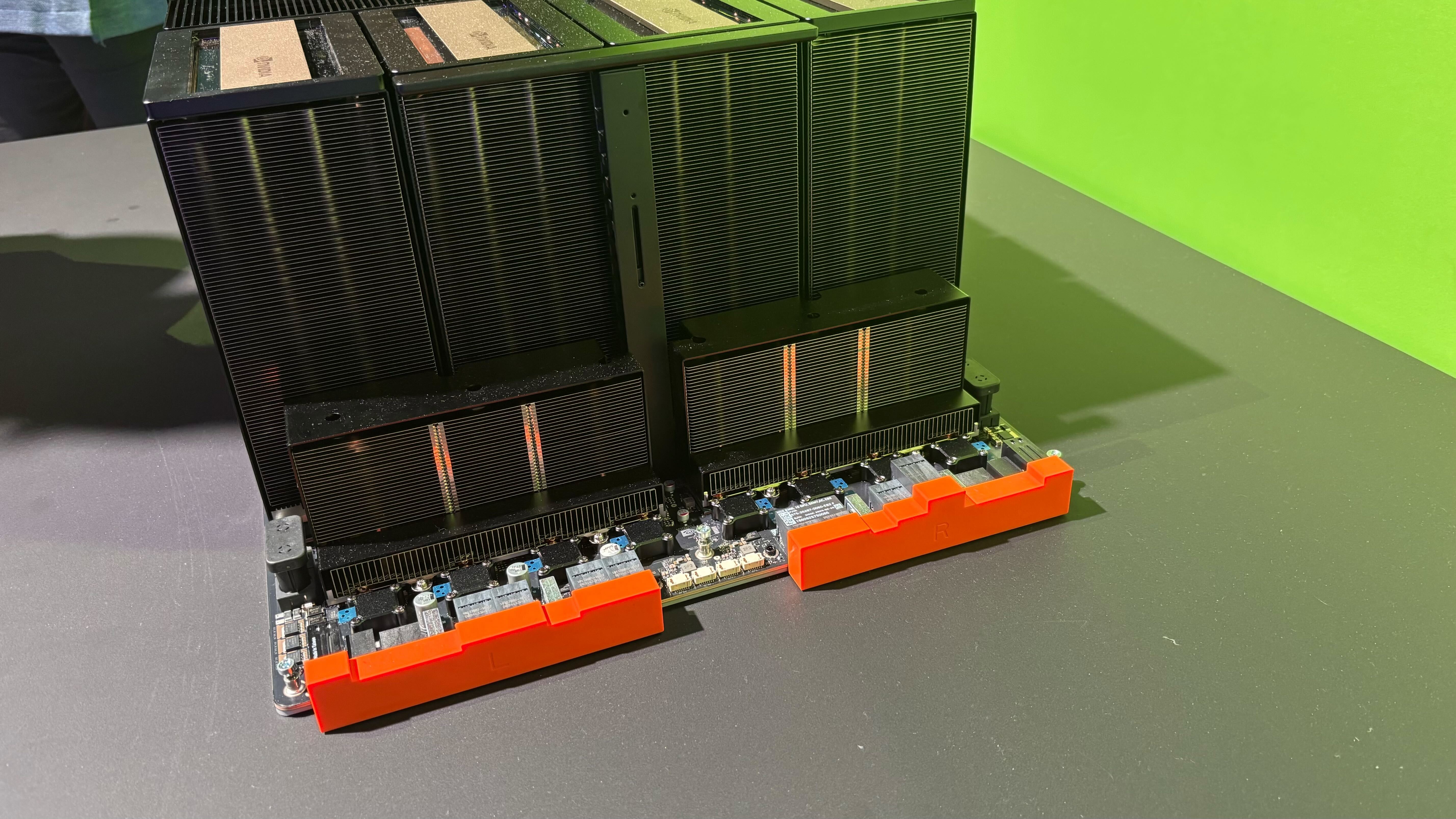
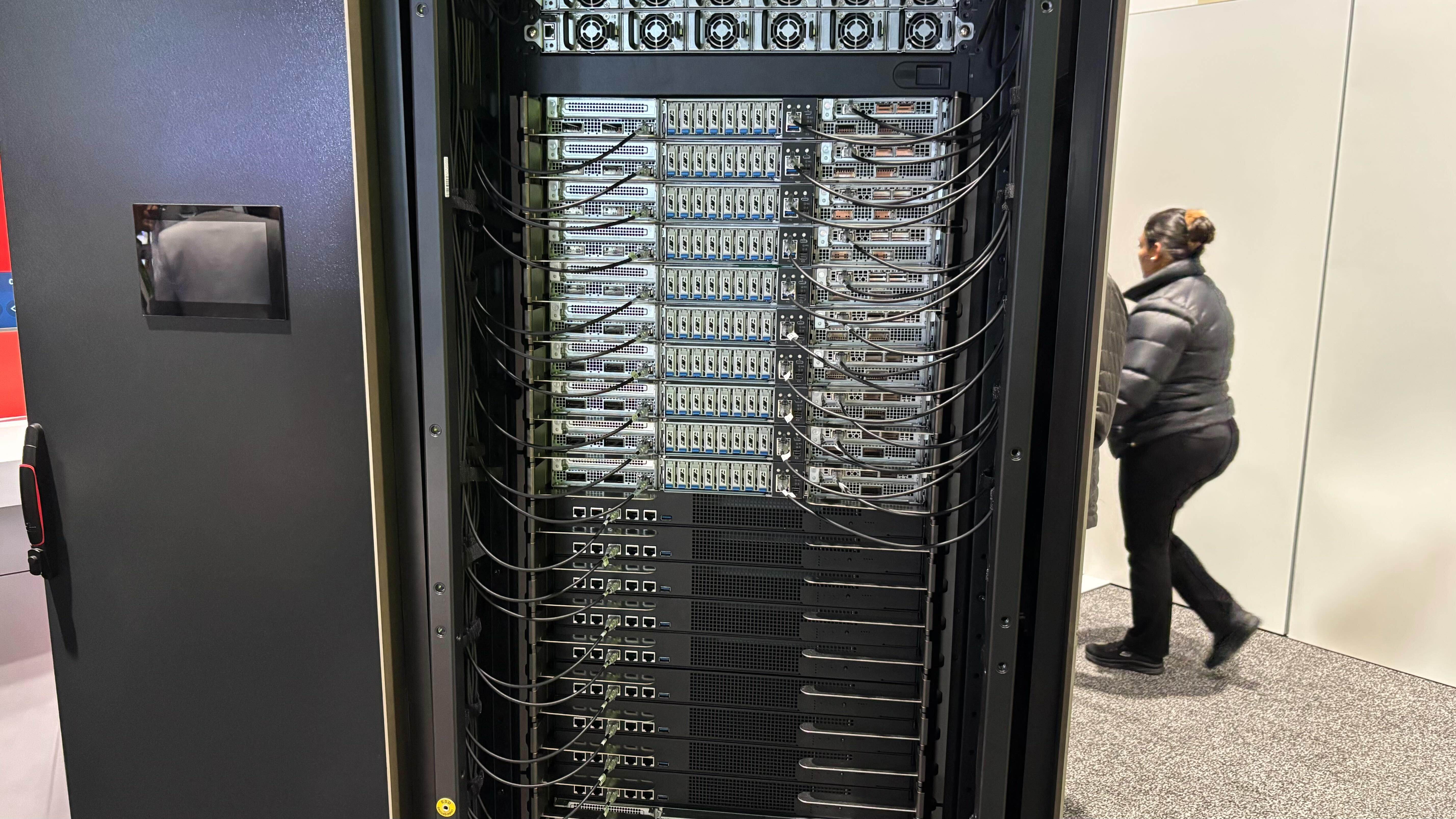
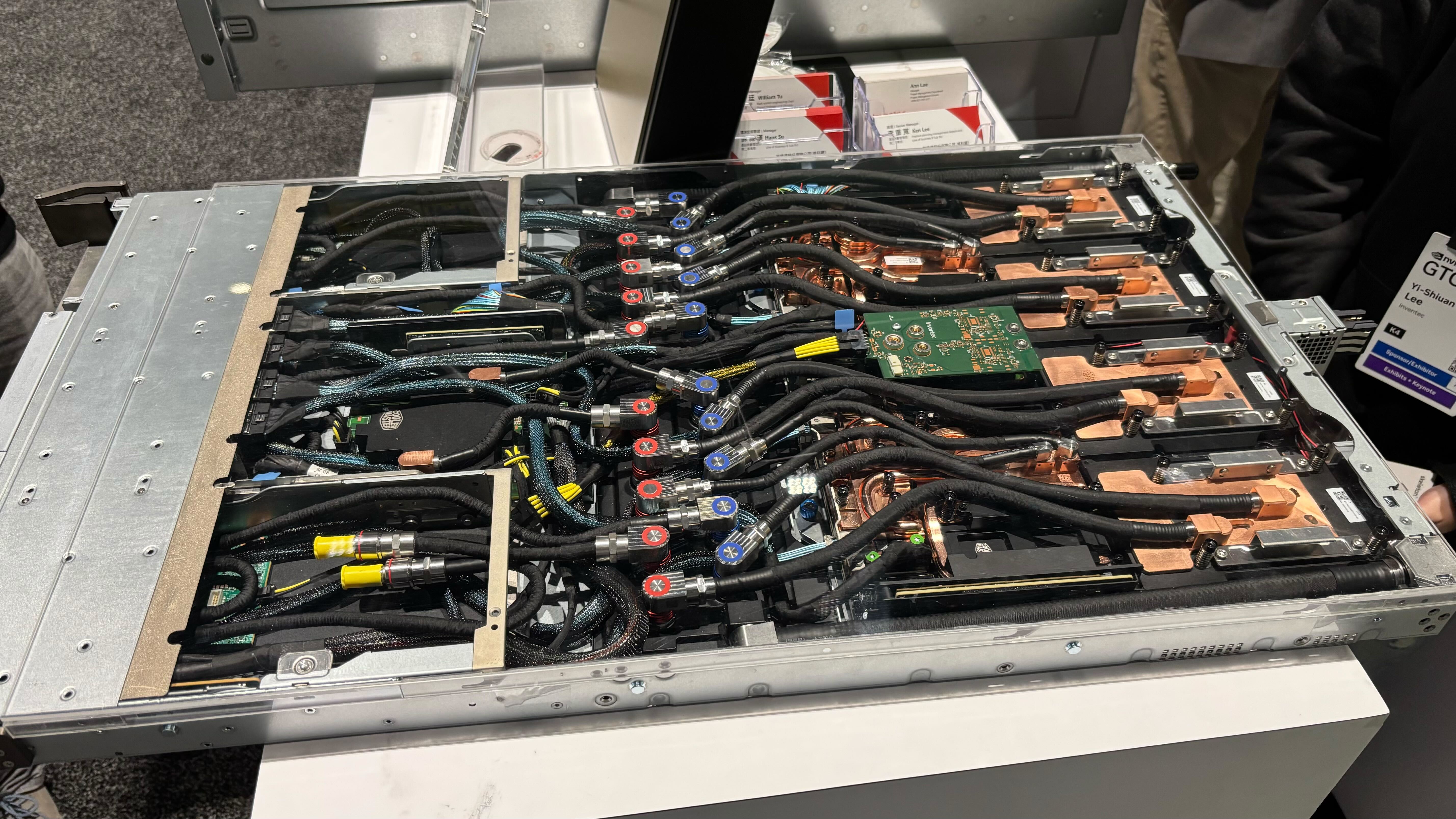
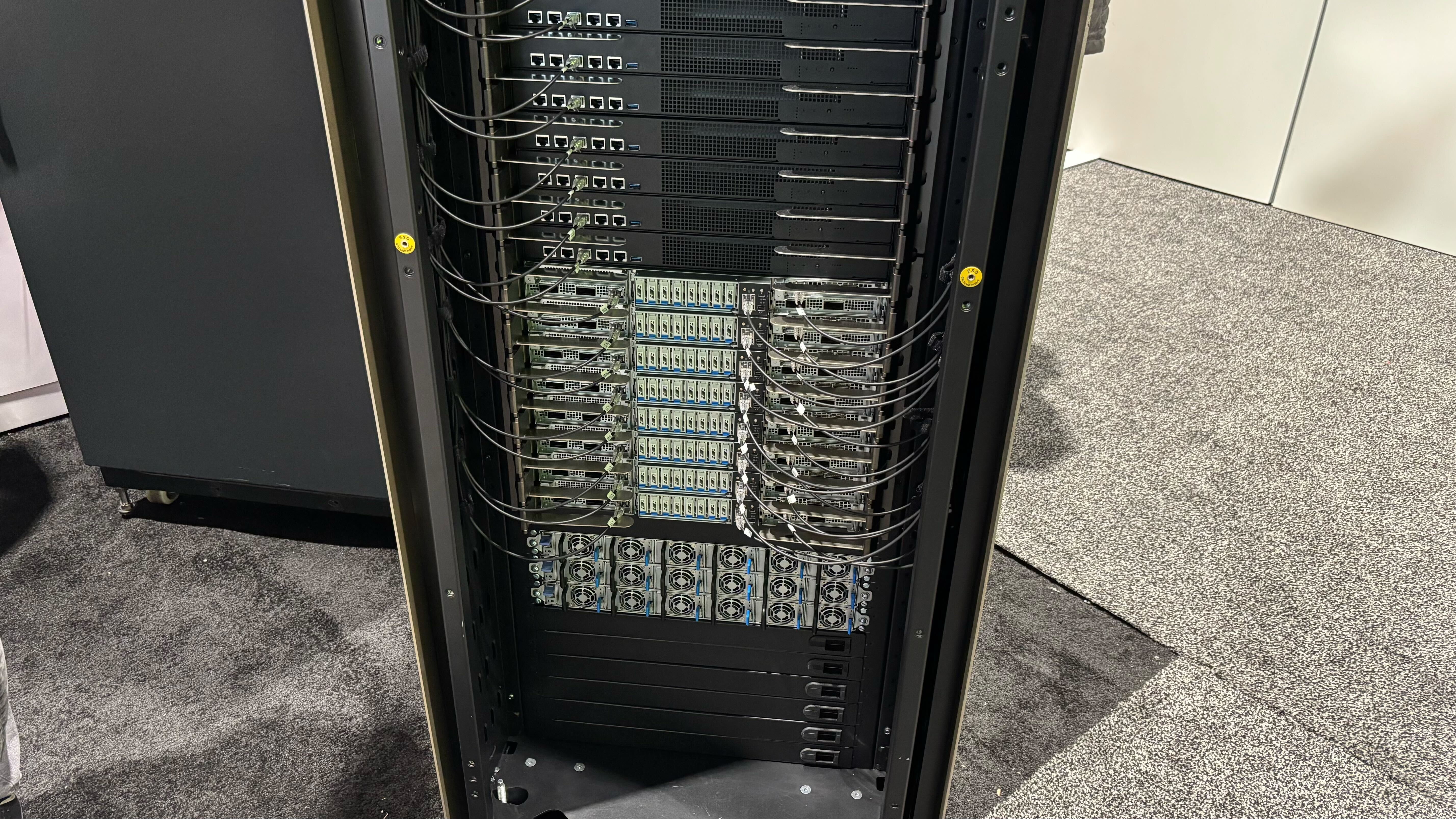
Platform | B300 | B200 | B100 |
---|---|---|---|
Configuration | Blackwell GPU | Blackwell GPU | Blackwell GPU |
FP4 Tensor Dense/Sparse | 15/30 petaflops | 10/20 petaflops | 7/14 petaflops |
FP6/FP8 Tensor Dense/Sparse | 7.5/15 petaflops ? | 5/10 petaflops | 3.5/7 petaflops |
INT8 Tensor Dense/Sparse | 7.5/15 petaops ? | 5/10 petaops | 3.5/7 petaops |
FP16/BF16 Tensor Dense/Sparse | 3.75/7.5 petaflops ? | 2.5/5 petaflops | 1.8/3.5 petaflops |
TF32 Tensor Dense/Sparse | 1.88/3.75 petaflops ? | 1.25/2.5 petaflops | 0.9/1.8 petaflops |
FP64 Tensor Dense | 68 teraflops ? | 45 teraflops | 30 teraflops |
Memory | 288GB (8x36GB) | 192GB (8x24GB) | 192GB (8x24GB) |
Bandwidth | 8 TB/s ? | 8 TB/s | 8 TB/s |
Power | ? | 1300W | 700W |
We asked for some clarification on the performance and details for Blackwell Ultra B300 and were told: "Blackwell Ultra GPUs (in GB300 and B300) are different chips than Blackwell GPUs (GB200 and B200). Blackwell Ultra GPUs are designed to meet the demand for test-time scaling inference with a 1.5X increase in the FP4 compute." Does that mean B300 is a physically larger chip to fit more tensor cores into the package? That seems to be the case, but we're awaiting further details.
What's clear is that the new B300 GPUs will offer significantly more computational throughput than the B200. Having 50% more on-package memory will enable even larger AI models with more parameters, and the accompanying compute will certainly help.
Nvidia gave some examples of the potential performance, though these were compared to Hopper, so that muddies the waters. We'd like to see comparisons between B200 and B300 in similar configurations — with the same number of GPUs, specifically. But that's not what we have.
By leveraging FP4 instructions, using B300 alongside its new Dynamo software library to help with serving reasoning models like DeepSeek, Nvidia says an NV72L rack can deliver 30X more inference performance than a similar Hopper configuration. That figure naturally derives from improvements to multiple areas of the product stack, so the faster NVLink, increased memory, added compute, and FP4 all factor into the equation.
In a related example, Blackwell Ultra can deliver up to 1,000 tokens/second with the DeepSeek R1-671B model, and it can do so faster. Hopper, meanwhile, only offers up to 100 tokens/second. So, there's a 10X increase in throughput, cutting the time to service a larger query from 1.5 minutes down to 10 seconds.
The B300 products should begin shipping before the end of the year, sometime in the second half of the year. Presumably, there won't be any packaging snafus this time, and things won't be delayed, though Nvidia does note that it made $11 billion in revenue from Blackwell B200/B100 last fiscal year. It's a safe bet to say it expects to dramatically increase that figure for the coming year.
Stay On the Cutting Edge: Get the Tom's Hardware Newsletter
Get Tom's Hardware's best news and in-depth reviews, straight to your inbox.
Jarred Walton is a senior editor at Tom's Hardware focusing on everything GPU. He has been working as a tech journalist since 2004, writing for AnandTech, Maximum PC, and PC Gamer. From the first S3 Virge '3D decelerators' to today's GPUs, Jarred keeps up with all the latest graphics trends and is the one to ask about game performance.
-
DS426 Gotta have those ultra halo products to firmly seat oneself as "#1".Reply
I just have to say no. More marketing, more "WOW!" numbers, but it's really a distortion of the compute market and synthesizing Moore's Law into a reality that works if you ignore cost and efficiency scaling. Just not impressed when -- as far as "AI" has come -- a quarter of the earth's population still doesn't have access to fresh drinking water, and I don't see that needle changing rapidly.
The technologists and industrialists have horded wealth and IP to unimaginable levels. Kind of strange post for me, but I stand my ground that the more successful you are individually and as a organization, the more you contribute to society. This just screams of excess to me...and is only getting worse. Look at the gaming dGPU situation today, with both AMD and nVidia claiming that they had decent supply and are selling in record numbers.
If you don't have deep pockets, you're out. You - are - out. That's our "bright" future. -
bit_user
It's not multiplicative. The only way you get to multiply together any improvements is like if you manage to improve the overlap between compute and communication, so that computation isn't blocking on it, as much. That multiple could then be applied to the improvements gained from going to fp4, and the product would be the final speedup. Algorithmic improvements should also factor in, which I suspect a good chunk of that improvement is from. And the 30x number is almost certainly an outlier, rather than typical. Blackwell just isn't that much faster than Hopper.The article said:By leveraging FP4 instructions, using B300 alongside its new Dynamo software library to help with serving reasoning models like DeepSeek, Nvidia says an NV72L rack can deliver 30X more inference performance than a similar Hopper configuration. That figure naturally derives from improvements to multiple areas of the product stack, so the faster NVLink, increased memory, added compute, and FP4 all factor into the equation.
Improvements in things like HBM bandwidth should only be enough to (hopefully) keep pace with the compute improvements. Same with NVLink. I doubt it will be less blocked on HBM or NVLink than Hopper was.
It could also be something like the increased HBM capacity enabling local storage of weights that previously had to be fetch from an attached GPU or Grace node. -
JarredWaltonGPU
Yes, which is precisely what I'm saying. We haven't tested this. Nvidia isn't giving exact details. It's just saying it's 30X faster. Now, I personally think "increased memory" is a big part of the increased performance. It could also be the faster NVLink, but I don't know that it's as pertinent. And there's MIG and other stuff in play where perhaps the software is better able to distribute work across the GPUs. Someone else will have to test that; this is just what Nvidia has claimed.bit_user said:It's not multiplicative. The only way you get to multiply together any improvements is like if you manage to improve the overlap between compute and communication, so that computation isn't blocking on it, as much. That multiple could then be applied to the improvements gained from going to fp4, and the product would be the final speedup. Algorithmic improvements should also factor in, which I suspect a good chunk of that improvement is from. And the 30x number is almost certainly an outlier, rather than typical. Blackwell just isn't that much faster than Hopper.
Improvements in things like HBM bandwidth should only be enough to (hopefully) keep pace with the compute improvements. Same with NVLink. I doubt it will be less blocked on HBM or NVLink than Hopper was.
It could also be something like the increased HBM capacity enabling local storage of weights that previously had to be fetch from an attached GPU or Grace node. -
jp7189 30x only if you can squint hard enough to make an orange look like an apple... in the same vain as the 5070 being faster than a 4090... different compute process, different precision, etc. It's the reason to see hopper in the comparison... H100 doesn't support the same data types as B200 and B300.Reply
As much as I hate that from a technical stand point, it's perfect for marketing because their target market for upgrades is H100 not B200.