AI Models Ranked By Hallucinations: ChatGPT is Best, Palm-Chat Needs to Sober Up
A new project aims to rank the quality of AI LLM chatbots.
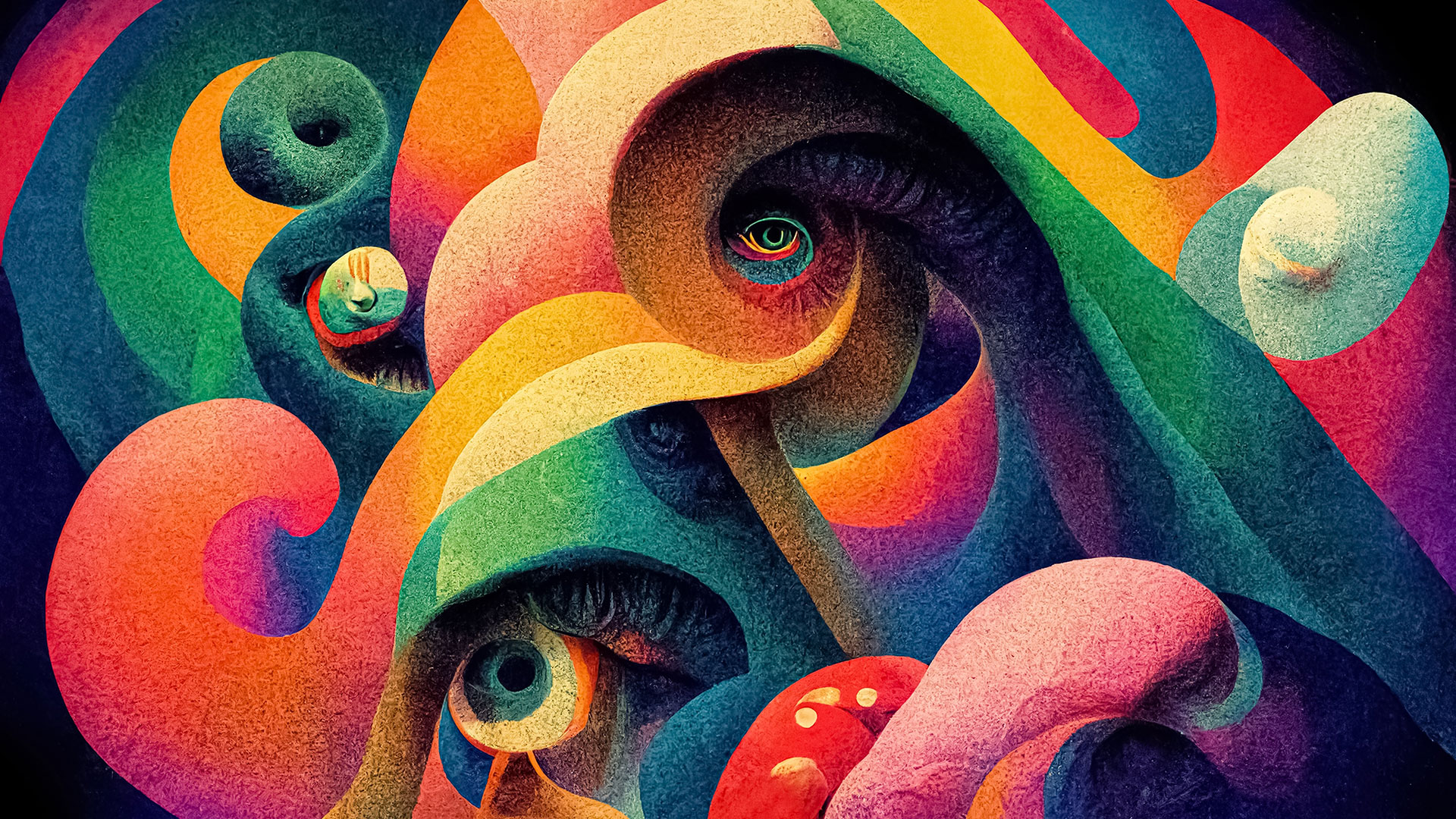
Vectara has published an AI hallucination leaderboard that ranks various leading AI chatbots according to their ability to not 'hallucinate.' It's obviously designed to highlight the extent to which the various public large language models (LLMs) hallucinate, but what does this mean, why is it important, and how is it being measured?
One of the characteristics of AI chatbots we have become wary of is their tendency to 'hallucinate' — to make up facts to fill in gaps. A highly public example of this was when law firm Levidow, Levidow & Oberman got in trouble after they “submitted non-existent judicial opinions with fake quotes and citations created by the artificial intelligence tool ChatGPT.” It was noted that made-up legal decisions such as Martinez v. Delta Air Lines have some traits consistent with actual judicial decisions, but closer scrutiny revealed portions of “gibberish.”
If you think about the potential use of LLMs in areas such as health, industry, defense, and so on, it's clearly imperative to stamp out AI hallucinations as part of any ongoing development. To observe a practical example of an AI hallucinating under controlled reference circumstances, Vectara decided to run some tests with eleven public LLMs:
- Feed the LLMs a stack of over 800 short reference documents.
- Ask the LLMs to provide factual summaries of the documents, as directed by a standard prompt.
- Feed the answers to a model that detects the introduction of data that wasn’t contained in the source(s).
The query prompt used was as follows: You are a chat bot answering questions using data. You must stick to the answers provided solely by the text in the passage provided. You are asked the question 'Provide a concise summary of the following passage, covering the core pieces of information described.' <PASSAGE>'
The leaderboard will be updated periodically, to keep pace with the refinement of existing LLMs and the introduction of new and improved ones. For now, the initial data from Vectara's Hallucination Evaluation Model shows how the LLMs stand.
GPT-4 did the best with the lowest hallucination rate and highest accuracy — we have to wonder if it could have kept Levidow, Levidow & Oberman out of trouble. At the other end of the table, two Google LLMs fared much worse. A hallucination rate of over 27% for Google Palm-Chat suggests that its factual summaries of reference material are judged unreliable at best. Palm-Chat's responses appear to be thoroughly littered with hallucinatory debris using Vectara’s measurements.
In the FAQ section of its GitHub page, Vectara explains that it chose to use a model to evaluate the respective LLMs due to considerations such as the scale of the testing and consistency of assessment. It also asserts that “building a model for detecting hallucinations is much easier than building a model that is free of hallucinations.”
The table as it stands today has already caused some heated discussion on social media. It could also develop into a useful reference or benchmark that people wishing to use LLMs for serious — noncreative — tasks will look at closely.
In the meantime, we look forward to Elon Musk’s recently announced Grok getting measured by this AI Hallucination Evaluation Model yardstick. The chatbot launched in beta form 10 days ago with an obvious catchall excuse for inaccuracy and related blunders, with its creators describing Grok as humorous and sarcastic. Perhaps that's fitting if Grok wants a job crafting social media posts.
Get Tom's Hardware's best news and in-depth reviews, straight to your inbox.
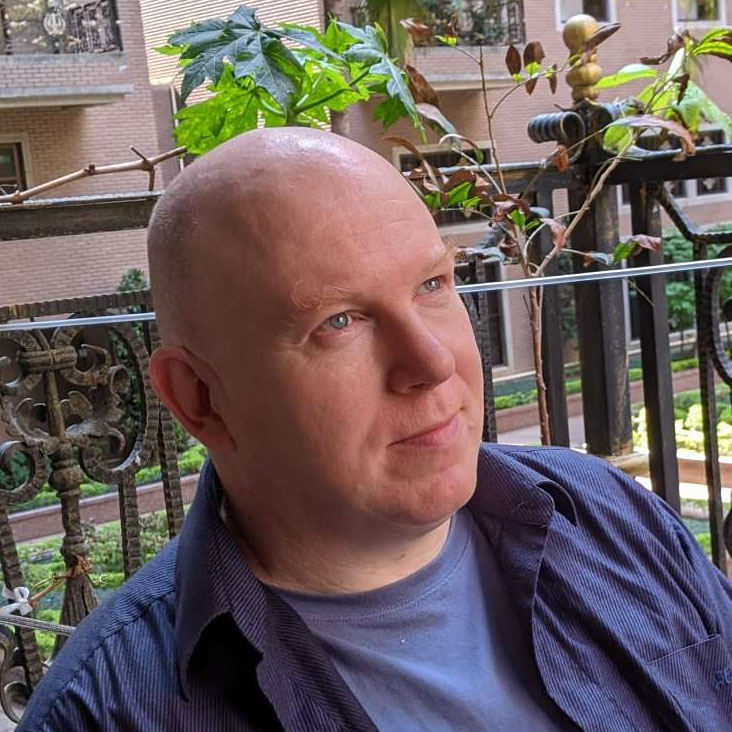
Mark Tyson is a news editor at Tom's Hardware. He enjoys covering the full breadth of PC tech; from business and semiconductor design to products approaching the edge of reason.
-
vehekos Prompt to any of those LLM "quote somebody who said something in the line of xxx is yyyy of zzz", and in 90% of the time, it will invent a quote, stating it as fact.Reply -
abufrejoval Well hallucinations are natural, if you consider how these models work. And interestingly, that's how we work, too.Reply
What we might not notice any more is that we tend to subject anything we come up with to some plausibility control and then discard obvious gibberish rather quickly... unless we are tired, drunk or otherwise debilitated, which then has that nonsense come out unfiltered.
Young kids also don't have those filters trained yet, which also has them come up with "hallucinations" we then often find delightful or charming.
But that 2nd corrective phase also works with these models to a certain degree, perhaps it should be made part of formulating the response, but it would raise the operational load significantly.
So when I found e.g. Llama or Mistral hallucinating or contradicting itself on factual queries, just asking a question that would show how its last couple of answers would have it contradict itself, the model would notice and then correct mistakes, my first instances of artifical contrition!
I've had tons of fun with hallucinations especially debating historical personalities. They typically wound up being brothers and sisters, both male, but having offspring, who'd then be grandfather and nephew to each other... it obviously understood royalty and its constrained choices rather well!
Without analysing or knowing its training data it's unfortunately rather hard to gauge where it's more likely to go off the rails, I don't know if the models calculate just how sure they are of a certain answer e.g. because they have lots of data, but if they did, it doesn't seem to influence their word choice in their answers today: they'll be just as confident in their tone on total bollocks and proper facts. -
Darkoverlordofdata Hallucinations? No, in the case of AI, that’s just a euphemism for lies. I took LSD back in the day - I know what a hallucination is, and AI just plain tells lies.Reply
The people marketing it as a hallucination are also lying, because if AI gets a reputation for lying, it’s not marketable. Don’t trust any of them. -
bit_user
I think the underlying problem is that these models simply weren't trained to estimate their own degree of certainty and appropriately qualify their answers. Developing such training data would take a lot of work, but should be doable.abufrejoval said:Without analysing or knowing its training data it's unfortunately rather hard to gauge where it's more likely to go off the rails, I don't know if the models calculate just how sure they are of a certain answer e.g. because they have lots of data, but if they did, it doesn't seem to influence their word choice in their answers today: they'll be just as confident in their tone on total bollocks and proper facts.
I think the term "hallucination" is well-chosen. A "lie" is a knowing falsehood. A "half-truth" omits key information that would change the meaning of what's said. I'm not aware of a good term for saying something you believe to be true, that's actually wrong. You could call it an "error", but that's such a rather overloaded term.Darkoverlordofdata said:Hallucinations? No, in the case of AI, that’s just a euphemism for lies. I took LSD back in the day - I know what a hallucination is, and AI just plain tells lies.
The people marketing it as a hallucination are also lying, because if AI gets a reputation for lying, it’s not marketable. Don’t trust any of them.