AI engineers claim new algorithm reduces AI power consumption by 95% — replaces complex floating-point multiplication with integer addition
Addition is simpler than multiplication, after all.
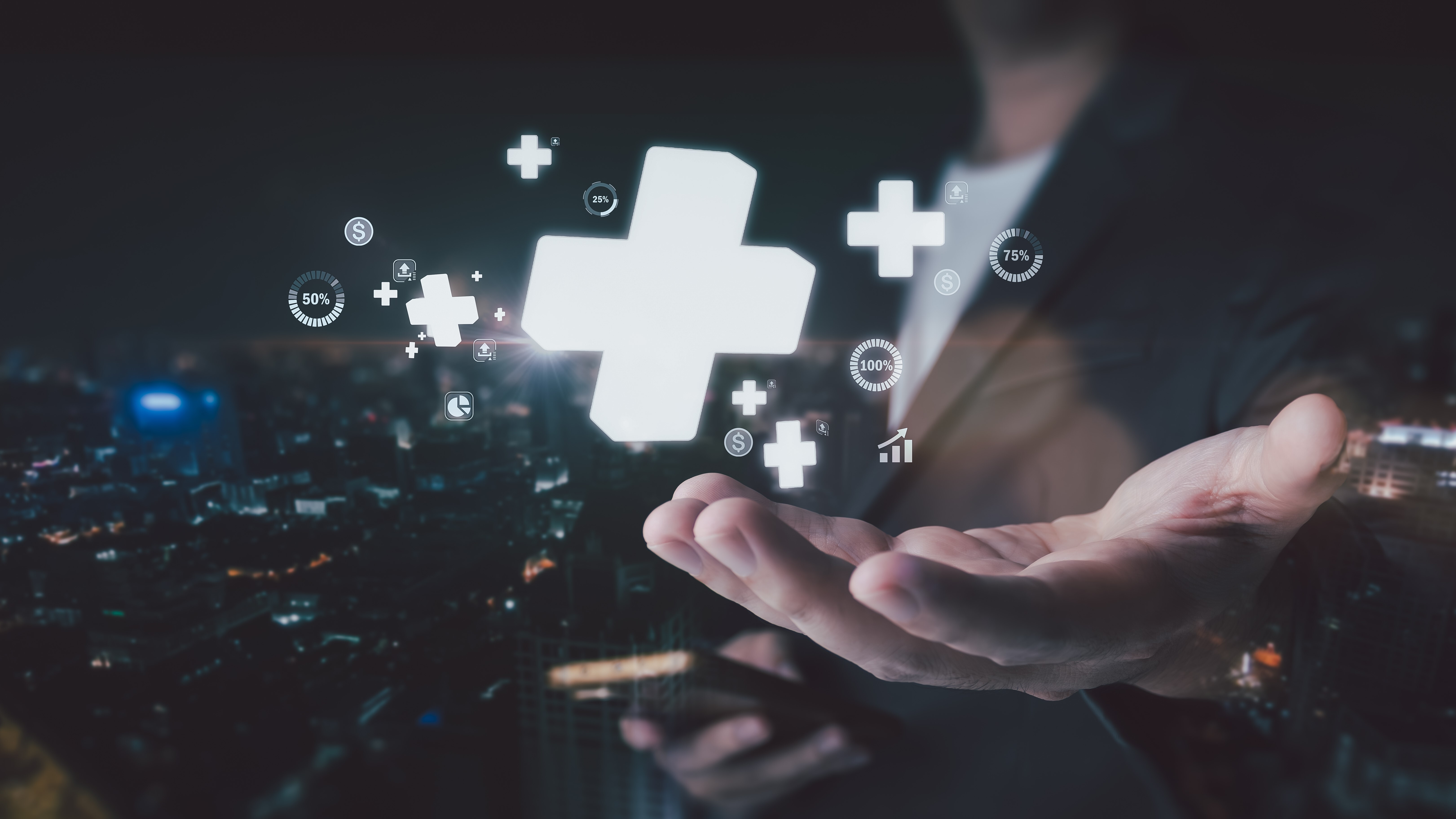
Engineers from BitEnergy AI, a firm specializing in AI inference technology, has developed a means of artificial intelligence processing that replaces floating-point multiplication (FPM) with integer addition.
The new method, called Linear-Complexity Multiplication (L-Mul), comes close to the results of FPM while using the simpler algorithm. But despite that, it’s still able to maintain the high accuracy and precision that FPM is known for. As TechXplore reports, this method reduces the power consumption of AI systems, potentially up to 95%, making it a crucial development for our AI future.
Since this is a new process, popular and readily available hardware on the market, like Nvidia’s upcoming Blackwell GPUs, aren't designed to handle this algorithm. So, even if BitEnergy AI’s algorithm is confirmed to perform at the same level as FPM, we still need systems that could handle it. This might give a few AI companies pause, especially after they just invested millions, or even billions, of dollars in AI hardware. Nevertheless, the massive 95% reduction in power consumption would probably make the biggest tech companies jump ship, especially if AI chip makers build application-specific integrated circuits (ASICs) that will take advantage of the algorithm.
Power is now the primary constraint on AI development, with all data center GPUs sold last year alone consuming more power than one million homes in a year. Even Google put its climate target in the backseat because of AI’s power demands, with its greenhouse gas emissions increasing by 48% from 2019, instead of declining year-on-year, as expected. The company’s former CEO even suggested opening the floodgates for power production by dropping climate goals and using more advanced AI to solve the global warming problem.
But if AI processing can be more power efficient, then it seems that we can still get advanced AI technologies without sacrificing the planet. Aside from that, this 95% drop in energy use would also reduce the burden that these massive data centers put on the national grid, reducing the need to build more energy plants to power our future quickly.
While most of us are amazed by the additional power that new AI chips bring every generation, true advancement only comes when these processors are more powerful and more efficient. So, if L-Mul works as advertised, then humanity could have its AI cake and eat it, too.
Get Tom's Hardware's best news and in-depth reviews, straight to your inbox.
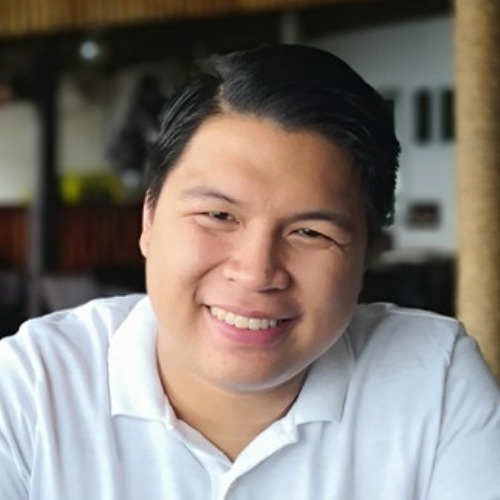
Jowi Morales is a tech enthusiast with years of experience working in the industry. He’s been writing with several tech publications since 2021, where he’s been interested in tech hardware and consumer electronics.
-
yahrightthere Seeing is believing, where's the white paper on this? As I could not find it.Reply
As for the load on the grid, have seen many reports of data center's inking deals to get this that & the other nuclear sites back up & running on line, as well as adding new nuclear sites & including small modular reactors, which would add to the infrastructure of the grid & reduce the load.
It's understood that all this will take time, money & efforts from all facets to accomplish this. -
ekio If that can apply to ClosedAI, Meta, Google and co, that would be a game changer but without proof, no beliefs.Reply -
nitrium "potentially up to 95%". I mean that corporate speak, for anywhere from 0% to 95%. The "Up to" number is not something anyone cares about. What's the average saving for typical AI workloads?Reply -
Mama Changa They don't say at what level of precision. Is it like fp4, fp16 etc. Also, have they never heard of fixed point math?Reply -
Li Ken-un “Work smarter not harder.” 🙂Reply
The operating cost to feed the power-hungry algorithms should convince them if the 95% reduction is true.
What’s the relatively fixed cost of investment into the hardware and nuclear power plants compared to the ongoing cost of feeding the less efficient algorithms? -
JTWrenn Not sure if promising or just a flare for the hope of capital investment. The hedged wording and apparently no fully working product screams "please invest in us" to me.Reply -
AkroZ Here is the paper: https://arxiv.org/html/2410.00907v2Reply
I have read it, it's interesting but it is listing only the advantages and not the downfalls, basically this is a paper to ask for investments.
They demonstrate higher precision than FP8 with theorically less costly operations but their implementation is a FP32 meaning that it use 4 times more memory and they do not calculate the potential energy drain of those memory operations.
This is not considered for inference but only for the execution of models (as memory is the main limiting factor), notably for AI processor unit. -
bit_user
Thanks for this! @yahrightthere take note!AkroZ said:Here is the paper: https://arxiv.org/html/2410.00907v2
They do list its limitations.AkroZ said:I have read it, it's interesting but it is listing only the advantages and not the downfalls, basically this is a paper to ask for investments.
They merely prototyped it on existing hardware. Nvidia GPUs, to be precise. Nvidia doesn't support general arithmetic on lower-precision data types than that.AkroZ said:They demonstrate higher precision than FP8 with theorically less costly operations but their implementation is a FP32 meaning that it use 4 times more memory and they do not calculate the potential energy drain of those memory operations.
From briefly skimming the paper, I think they're actually proposing to implement it at 16 bit, but they also work out the implementation cost at 8-bit.
"inference" is the term used for what I think you mean by "execution of models". Here's what the abstract says:AkroZ said:This is not considered for inference but only for the execution of models
"We further show that replacing all floating point multiplications with 3-bit mantissa ℒ-Mul in a transformer model achieves equivalent precision as using float8_e4m3 as accumulation precision in both fine-tuning and inference."
So, they claim that it's applicable to both inference and a subset of training work (i.e. fine-tuning). -
bit_user
The paper mostly focuses on comparing it against different fp8 number formats.Mama Changa said:They don't say at what level of precision.
What good would that do? The problem with fp multiplication is in the mantissa, which is actually cheaper than multiplying fixed-point, since it's fewer bits.Mama Changa said:Also, have they never heard of fixed point math? -
ex_bubblehead
Billions of $$ and decades to implement. I'm not holding my breath.yahrightthere said:Seeing is believing, where's the white paper on this? As I could not find it.
As for the load on the grid, have seen many reports of data center's inking deals to get this that & the other nuclear sites back up & running on line, as well as adding new nuclear sites & including small modular reactors, which would add to the infrastructure of the grid & reduce the load.
It's understood that all this will take time, money & efforts from all facets to accomplish this.