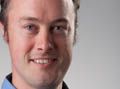
Meet Nvidia's Ian Buck
Thanks for taking the time to chat today. Let's start with some basics. Why don't you tell our readers a little bit about yourself and what you currently do at Nvidia?
I’m the Software Director for GPU Computing here at Nvidia. My main focus is to build and evolve a complete GPU computing platform, which includes system software, developer tools, language and compiler direction, libraries, and targeted applications and algorithms. With the help of a great team, we develop both the end-user software as well as set the direction for GPU computing within Nvidia.
Why don't we start from the beginning? I imagine that your interest in GPGPU didn't start when you were 5 years old--what were the events going on at Princeton or Stanford that really led you to discover an interest in GPGPU?
I did dabble in GPU computing in my Princeton days, experimenting with thermal convection and fluid simulation on graphics hardware, which, at the time was the SGI O2. Though things were so very constrained, it was hard to make a case for it.
I seriously started looking at GPU computing during my PhD research at Stanford. At Stanford, I, along with others in the research community realized that the natural progression of programmable graphics was the evolution of the GPU into a more general purpose processor. We wrote one of the first SIGGRAPH papers on ray tracing with DX9-class GPU hardware to help prove the point. What was so motivating about the work was that this commodity processor, which was available in everyone’s PC, was following a Moore’s law cubed performance growth rate, way faster than the CPU. This begged the question: what could a PC do if it had multiple orders magnitude more computing horsepower than today? A total game-changer for the computational sciences as well as computer vision, AI, data mining, and graphics.
What was your role with Brook?
After working on the ability to ray trace on the GPU, my research focus at Stanford switched to understanding the right programming model for GPU computing. At the time, many others had shown that the GPU was good at a variety of different applications, but there wasn’t a good framework or programming model on how one should think about the GPU as a compute device. At the time, it required a PhD in computer graphics to be able to port an application to the GPU. So I started the Brook project with the goal of defining a programming language for GPU computing, which abstracted the graphics-isms of the GPU into more general programming concepts. Brook’s fundamental programming concept was the “stream,” which was a collection of data elements requiring similar work. Brook eventually became my PhD thesis at Stanford.
Stay on the Cutting Edge
Join the experts who read Tom's Hardware for the inside track on enthusiast PC tech news — and have for over 25 years. We'll send breaking news and in-depth reviews of CPUs, GPUs, AI, maker hardware and more straight to your inbox.
Your work started with Merrimac, the Stanford Streaming Super Computer. How is this different from something like a Tesla?
Brook’s programming model concepts were applicable to more than just GPUs. At Stanford we worked on two different implementations of the Brook programming model: one for GPUs, the other for Merrimac which was a research architecture developed at Stanford. Many of the ideas pioneered as part of Merrimac did influence how GPUs could be improved for general purpose computing. It should also be noted that Bill Dally who was the principle investigator of Merrimac at Stanford, is now the Chief Scientist at Nvidia.
Did CUDA have any roots in Gelato? What was the first academic exploration of GPGPU? What about the first commercialized use?
I started CUDA while completing my research at Stanford. Nvidia was already very supportive of my research and clearly saw the potential to better enable GPU computing on the hardware side of things. I joined Nvidia to start the CUDA project in 2005. At the time, it was just myself and one other engineer. We’ve now grown the project into the organization it is today, and a central component to Nvidia’s GPUs today.
www.gpgpu.org provides a nice history of GPU computing, dating back to 2002.
Currently, AMD pushes Brook as the programing language of choice for GPGPU, whereas Nvidia has C with CUDA extensions. How would you compare the strengths/weaknesses of both?
Starting at Nvidia, we had an opportunity to revisit some of the fundamental design decisions of Brook, which were largely based on what DX9-class hardware could achieve. One of key limitations was the constraints of the memory model, which required the programmer to map their algorithm around a fairly limited memory access pattern. With our C with CUDA extensions, we relaxed those constraints. Fundamentally, the programmer was simply given a massive pool of threads and could access memory any way he or she wished. This improvement, as well as a few others, allowed us to implement full C language semantics on the GPU.
-
How about some GPU acceleration for linux! I'd love blue-ray and HD content to be gpu accelerated by VlC or Totem. Nvidia?Reply
-
matt87_50 I ported my simple sphere and plane raytracer to the gpu (dx9) using Render Monkey, it was soo simple and easy, only took a few hours, nearly EXACTLY the same code. (using hlsl, which is basically c) and it was so beautiful, what took seconds on the cpu was now running at over 30fps at 1680x1050.Reply
a monumental speed increase with hardly any effort (even without a gpgpu language)
its going to be nothing short of a revolution when gpgpu goes mainstream (hopefully soon, with dx11)
computer down on power? don't replace the cpu, just dump another gpu in one of the many spare pci16x slots and away you go, no fussing around with sli or crossfire and the compadibillity issues they bring. it will just be seen as another pile of cores that can be used!
even for tasks that can't be done easily on the gpu architecture, most will still probably run faster than they would on the cpu, because the brute power the gpu has over the cpu is so immense, and as he kinda said, most of the tasks that aren't suited to gpgpu don't need speeding up anyway. -
shuffman37: Nvidia does have gpu accelerated video on linux. Link to wikipedia http://en.wikipedia.org/wiki/VDPAU about VDPAU. Its gaining support by a lot of different media players.Reply
-
NVIDIA, saying that "spreadsheet is already fast enough" may be misleading. Business users have the money. Spreadsheets are already installed (huge existing user base). Many financial spreadsheets are very complicated 24 layers, 4,000 lines, with built in Monte Carlo simulations.Reply
Making all these users instantly benefit from faster computing may be the road for success for NVIDIA.
Dr. Drey
Bloomington, IN -
raptor550 Although I appreciate his work... I had to AdBlock his face. Sorry, its just creepy.Reply -
techpops While I can't get enough of GPGPU articles, it really saddens me that Nvidia is completely ignoring Linux and not because I'm a Linux user. Ignoring Linux stops the GPU from being the main source for rendering in 3D software that also is available under Linux. So in my case, where I use Cinema 4D under Windows, I'll never see the massive speedups possible because Maxon would never develop for a Windows and Mac only platform.Reply
It's worth pointing out here that I saw video of Cuda accelerated global illumination from a single Nvidia graphics card, going up against an 8 core CPU beast. Beautiful globally illuminated images were taking 2-3 minutes to render, just for a single image on the 8 core PC. The Cuda one, rendering to the same quality was rendering at up to 10 frames per second! That speed up is astonishing and really makes an upgrade to a massive 8 core PC system seem pathetic in the face of that kind of performance.
One can only imagine what would be possible with multiple graphics cards.
I also think the killer app for the GPU is not ultimately going to be graphics at all, while in the early days it will be, further down the line, I think it will be augmented reality that takes over for the main GPU use. Right now, it's pretty shoddy using a smart phone for augmented reality applications, everything is dependent on GPS, and that's totally unreliable and will remain so. What's needed for silky smooth AR apps is a lot of processing power to recognize shapes and interpret all that visual data you get through a camera to work with the GPS. So if you're standing in front of a building, an arrow can point on the floor leading into the buildings entrance because the GPS has located the building and the gpu has worked out where the windows and doors are and made overlaid graphics that are motion locked to the video.
I think AR is going to change everything with portable computers, but only when enough compute power is in a device to make it a smooth experience, rather than the jerky unreliable experimental toy it is on today's smart phones. -
pinkzeppelin97 zipzoomflyhighIf my forehead was that big due to a retreating hairline, I would shave my head.Reply
amen to that -
tubers cpu and gpu combined? will that bring more profit to each of their respective companies? hmmReply -
jibbo shuffman37How about some GPU acceleration for linux! I'd love blue-ray and HD content to be gpu accelerated by VlC or Totem. Nvidia?Reply
There is GPU acceleration for Linux. I believe NVIDIA's provided a CUDA driver, compiler, toolkit, etc for Linux since day 1.