Elon Musk and Larry Ellison begged Nvidia CEO Jensen Huang for AI GPUs at dinner
Shut up and take my money.
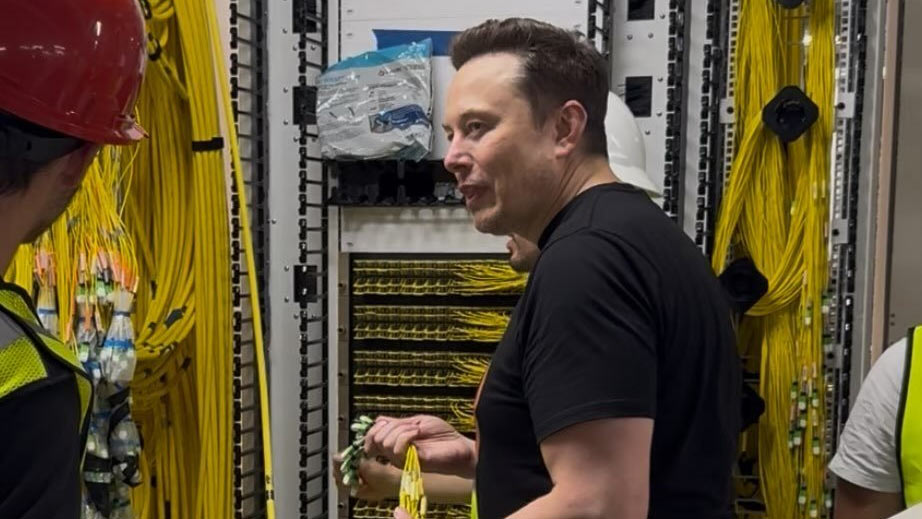
During the company's latest earnings call, Oracle founder Larry Ellison admitted (via Barron’s) that he had to beg Nvidia CEO Jensen Huang to supply his company with its latest GPUs. “In Nobu Palo Alto, I went to dinner with Elon Musk, Jensen Huang, and I would describe the dinner as me and Elon begging Jensen for GPUs. Please take our money; no, take more of it. You’re not taking enough of it; we need you to take more of our money, please,” Ellison said during the call. “It went okay; it worked.”
$ORCL CTO Larry Ellison: "I went to dinner with @elonmusk, Jensen Huang..& I would describe the dinner as Oracle & me & Elon begging Jensen for GPUs. Please take our money....No, no, take more of it. You're not taking out enough. We need you to take more of our money, please" pic.twitter.com/atBUJaULCSSeptember 14, 2024
It looks like this dinner was very productive indeed and that it was money well spent for Ellison and Oracle. The company recently announced that it will create a Zettascale AI supercluster composed of 131,072 Nvidia GB200 NVL72 Blackwell GPUs that delivers 2.4 ZettaFLOPS of AI performance—more powerful than Musk and xAI’s Memphis Supercluster, which currently has 100,000 Nvidia H100 AI GPUs.
Oracle’s AI plans demand an incredible amount of power, which is also why the company has already secured permits to build three modular nuclear reactors to deliver the electrical needs of its facilities. However, since deploying nuclear reactors to data centers will likely take several years, the company could, in the meantime, follow Musk’s lead and use massive mobile generators to boost local power supply if needed.
Despite being smaller than other big tech companies that offer data center services, like Amazon Web Services, Microsoft Azure, and Google Cloud, Oracle Cloud Infrastructure (OCI) has a distinct advantage over these giants. According to Barron’s report, OCI provides better flexibility and can meet specific requirements that some customers demand. It can even provide offline servers that run on its own networking infrastructure to maximize security for the most demanding clients.
But despite its size, Oracle is pushing its investments in AI. Ellison says that frontier AI models coming in the next three years will cost $100 billion to train, echoing Anthropic CEO Dario Amodei’s thoughts on the matter. And it seems that OCI wants to become one of the leaders in AI processing. “Someone’s going to be better at this than anybody else, and multiple people are trying, and there is a race,” said Ellison during the call. He then added later, “Getting there first is a big deal.”
Get Tom's Hardware's best news and in-depth reviews, straight to your inbox.
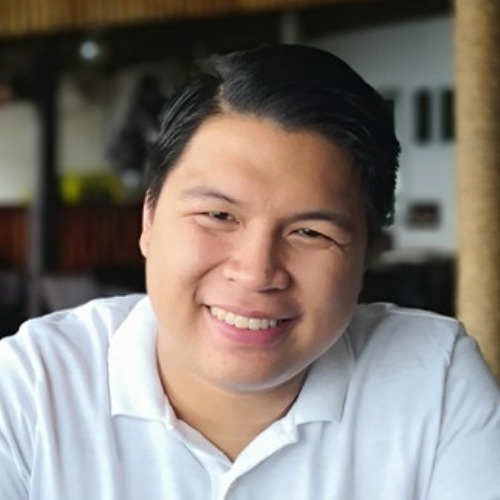
Jowi Morales is a tech enthusiast with years of experience working in the industry. He’s been writing with several tech publications since 2021, where he’s been interested in tech hardware and consumer electronics.
-
hotaru251 if two rich guys are begging another guy to take their $ they should be paying more in taxes.Reply -
JRStern The customer is always right, ROFLMAO.Reply
Ellison says that frontier AI models coming in the next three years will cost $100 billion to train, echoing Anthropic CEO Dario Amodei’s thoughts on the matter.
Yeah, and Sam Altman who wants to raise $1t so he can be a player (that means he takes 10% off the top).
But they're wrong.
OpenAI's talk about their new o1 model that "thinks before it answers", does more work at inference time rather than putting all the load on pre-training. D'yall see what that means?
First it means needing more GPUs for inference time, OK, but maybe not that many. Actually GPU may not even be the right architecture to optimize this kind of inference, but that's complicated.
But it means a LOT less GPUs for training.
It even suggests that trillion parameter models will be decomposed saving exponential amounts of training work.
IOW I think it's the right path.
And also that it will cut even the big boys' demand for mass quantities of GPUs by a lot, by far more than are incrementally needed for inference.
Have a nice day. -
bit_user
He should enjoy it, while it lasts. Such a power imbalance never lasts forever.Elusive Ruse said:Jensen be eating good! More power to him, ...
This is funny, but it's actually their businesses that want the GPUs from his business.hotaru251 said:if two rich guys are begging another guy to take their $ they should be paying more in taxes.
Now, say what you will about real corporate taxes rates...
(if it weren't off-topic, that is. So, actually please don't.)
It definitely leaves a bad taste, when backroom deals can gain someone such an important advantage in what's supposed to be an open market. I'll bet Jensen would rather Larry not have posted about it, but at least it shed a glimpse of transparency.Tonet666 said:Why this sound like a scene from the move The Godfather 1 :ROFLMAO::ROFLMAO: -
jp7189
I'd actually say the future is inferencing moving outwards towards low power end user devices. A few large training datacenters and disturbed inferencing on very efficient modelsJRStern said:The customer is always right, ROFLMAO.
Yeah, and Sam Altman who wants to raise $1t so he can be a player (that means he takes 10% off the top).
But they're wrong.
OpenAI's talk about their new o1 model that "thinks before it answers", does more work at inference time rather than putting all the load on pre-training. D'yall see what that means?
First it means needing more GPUs for inference time, OK, but maybe not that many. Actually GPU may not even be the right architecture to optimize this kind of inference, but that's complicated.
But it means a LOT less GPUs for training.
It even suggests that trillion parameter models will be decomposed saving exponential amounts of training work.
IOW I think it's the right path.
And also that it will cut even the big boys' demand for mass quantities of GPUs by a lot, by far more than are incrementally needed for inference.
Have a nice day. -
bit_user
I think it depends a lot on how big the models are. You're not going to be using something like GPT 4 on a cell phone any time soon, just due to its sheer size. Not only does it chew up lots of storage, but also download bandwidth. Then, there's the issue of battery power, if you're inferencing huge models very much, like with some kind of Alexa/Siri assistant.jp7189 said:I'd actually say the future is inferencing moving outwards towards low power end user devices. A few large training datacenters and disturbed inferencing on very efficient models
There would also be IP concerns about letting models run on edge devices, for those which aren't already open source. All someone needs to do is find one device with a known exploit to bypass memory encryption and now your model leaks out into the world. -
jp7189
I'm thinking of the pruning and distillation work e.g. mistral et al are doing. The focus is on maintaining accuracy while greatly reducing size and processing power. The minitron 8b runs on fairly low power.bit_user said:I think it depends a lot on how big the models are. You're not going to be using something like GPT 4 on a cell phone any time soon, just due to its sheer size. Not only does it chew up lots of storage, but also download bandwidth. Then, there's the issue of battery power, if you're inferencing huge models very much, like with some kind of Alexa/Siri assistant.
There would also be IP concerns about letting models run on edge devices, for those which aren't already open source. All someone needs to do is find one device with a known exploit to bypass memory encryption and now your model leaks out into the world. -
JRStern
If there were value in it I suppose a terabyte model could be run on a phone, you just download it once a year or so, perhaps from some local genius bar where you pay $29 for the privilege (or purchase it on ROM and just buy an upgrade and plug it in as available). I think 1tb would cover GPT4, and if not I'll bet it could be compressed some just for edge distribution, with little or no impact on performance.bit_user said:I think it depends a lot on how big the models are. You're not going to be using something like GPT 4 on a cell phone any time soon, just due to its sheer size. Not only does it chew up lots of storage, but also download bandwidth.
Now the problem is that this new form of inference may need more horsepower than one typically finds in a phone. The old form probably is OK, the new form I'm going to guess not so much. But again, going to the other side, *your* phone may very well learn *your* patterns of interference and be able to cache the most important parts specifically to perform YOUR inferences. That's the real promise of edge computing, that it can be as individual as you are, assuming that's a good thing, LOL. -
bit_user
It's not like LLMs are renown for their accuracy. Yeah, a pruned version will still be useful for certain things, but current LLMs have more issues than just their resource requirements.jp7189 said:I'm thinking of the pruning and distillation work e.g. mistral et al are doing. The focus is on maintaining accuracy while greatly reducing size and processing power.