Nvidia is firing back at AMD, claims Nvidia H100 Is 2X faster than AMD's MI300X
Twice as fast when properly optimized
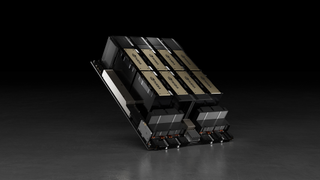
At its Instinct MI300X launch AMD asserted that its latest GPU for artificial intelligence (AI) and high-performance computing (HPC) is significantly faster than Nvidia's H100 GPU in inference workloads. Nvidia this week took time to show that the situation is quite the opposite: when properly optimized, it claims that its H100-based machines are faster than Instinct MI300X-powered servers.
Nvidia claims that AMD did not use optimized software for the DGX H100 machine, used to compare the performance to its Instinct MI300X-based server. Nvidia notes that high AI performance hinges on a robust parallel computing framework (which implies CUDA), a versatile suite of tools (which, again, implies CUDA), highly refined algorithms (which implies optimizations), and great hardware. Without any of the aforementioned ingredients, performance will be subpar, the company says.
According to Nvidia, its TensorRT-LLM features advanced kernel optimizations tailored for the Hopper architecture, a crucial performance enabler for its H100 and similar GPUs. This fine-tuning allows for models such as Llama 2 70B to run accelerated FP8 operations on H100 GPUs without compromising precision of the inferences.
To prove its point, Nvidia presented performance metrics for a single DGX H100 server equipped with eight H100 GPUs running the Llama 2 70B model. A DGX H100 machine is capable of completing a single inference task in just 1.7 seconds when set to a batch size of one, meaning it handles one request at a time, which is lower compared to AMD's eight-way MI300X machine's 2.5 seconds (based on the numbers published by AMD). This configuration provides the quickest response for model processing.
However, to balance response time and overall efficiency, cloud services often employ a standard response time for certain tasks (2.0 seconds, 2.3 seconds, 2.5 seconds on the graph). This approach allows them to handle several inference requests together in larger batches, thereby enhancing the server's total inferences per second. This method of performance measurement, which includes a set response time, is also a common standard in industry benchmarks like MLPerf.
Even minor compromises in response time can significantly boost the number of inferences a server can manage simultaneously. For instance, with a predetermined response time of 2.5 seconds, an eight-way DGX H100 server can perform over five Llama 2 70B inferences every second. This is a substantial increase compared to processing less than one inference per second under a batch-one setting. Meanwhile, Nvidia naturally did not have any numbers for AMD's Instinct MI300X when measuring performance in this setup.
Stay On the Cutting Edge: Get the Tom's Hardware Newsletter
Join the experts who read Tom's Hardware for the inside track on enthusiast PC tech news — and have for over 25 years. We'll send breaking news and in-depth reviews of CPUs, GPUs, AI, maker hardware and more straight to your inbox.
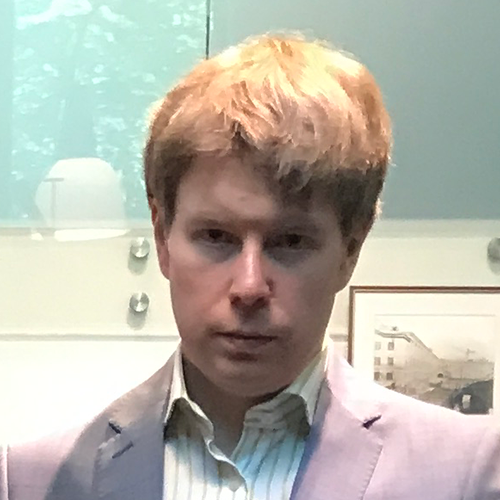
Anton Shilov is a Freelance News Writer at Tom’s Hardware US. Over the past couple of decades, he has covered everything from CPUs and GPUs to supercomputers and from modern process technologies and latest fab tools to high-tech industry trends.
-
-Fran- Isn't their FP8 and 16 special sauce, non-standard, types?Reply
--
EDIT: https://www.scaleway.com/en/docs/compute/gpu/reference-content/understanding-nvidia-fp8/
Looks like it was ARM, Intel and nVidia pushing the new FP8 type.
--
This feels like nVidia is casually telling people to go out of their way to optimize for a closed technology that will tie them down to nVidia forever... I don't know if this is the underlying message, but it does feel like it for sure.
Oh wait, they have been doing this with CUDA forver. Nevermind.
Regards. -
hotaru251 rule #1: never trust the results of tests stated by the maker of product....they will ALWAYS cherry pick results.Reply -
JarredWaltonGPU
Rule #2: Never trust the results from a competitor for a product, as they will ALWAYS (rotten?) cherry pick results.hotaru251 said:rule #1: never trust the results of tests stated by the maker of product....they will ALWAYS cherry pick results.
This is why independent third-party testing is critical for reviews. But I will say, in the case of AI workloads, I would expect properly implemented algorithms to trend toward being highly optimized. Companies are spending millions on the hardware, and they should put forth a real effort to get the software at least reasonably optimized. -
LastStanding
You do understand that this report exposes AMD's evil trickery just to give the ILLUSION, at whatever cost, to gain false momentum? And its not about nothing NVIDIA is doing wrong, huh?-Fran- said:Isn't their FP8 and 16 special sauce, non-standard, types?
--
EDIT: https://www.scaleway.com/en/docs/compute/gpu/reference-content/understanding-nvidia-fp8/
Looks like it was ARM, Intel and nVidia pushing the new FP8 type.
--
This feels like nVidia is casually telling people to go out of their way to optimize for a closed technology that will tie them down to nVidia forever... I don't know if this is the underlying message, but it does feel like it for sure.
Oh wait, they have been doing this with CUDA forver. Nevermind.
Regards. -
-Fran-
Of course I do.LastStanding said:You do understand that this report exposes AMD's evil trickery just to give the ILLUSION, at whatever cost, to gain false momentum? And its not about nothing NVIDIA is doing wrong, huh?
Like mentioned above, you never trust 1st party numbers anyway.
Regards. -
jeremyj_83
The biggest companies are always pushing their proprietary stuff just to make sure you can never leave. The smaller companies tend to use open standards since they don't have the resources to do proprietary stuff. Just look at G-Sync vs Free-Sync. If you have a G-Sync only monitor then you can only use an nVidia GPU to have the variable refresh rate. However, if you have a Free-Sync monitor you can use AMD, nVidia, or Intel GPUs because of the open standard AND the fact that variable refresh rate isn't anything "special." I'm sure that nVidia's FP8 isn't anything special either but they are going to do something with their libraries to lock it to CUDA only.-Fran- said:This feels like nVidia is casually telling people to go out of their way to optimize for a closed technology that will tie them down to nVidia forever... I don't know if this is the underlying message, but it does feel like it for sure. -
DSzymborski Twice as fast is one time faster, not two times faster. Two times faster would be three times as fast.Reply
(Which is one of the reasons why style guides frequently forbid use of the term as it's confusing even when used properly). -
NeoMorpheus
You forgot the media's favorite lock in tool, DLSS.-Fran- said:Isn't their FP8 and 16 special sauce, non-standard, types?
--
EDIT: https://www.scaleway.com/en/docs/compute/gpu/reference-content/understanding-nvidia-fp8/
Looks like it was ARM, Intel and nVidia pushing the new FP8 type.
--
This feels like nVidia is casually telling people to go out of their way to optimize for a closed technology that will tie them down to nVidia forever... I don't know if this is the underlying message, but it does feel like it for sure.
Oh wait, they have been doing this with CUDA forver. Nevermind.
Regards.
That said, You know that Nvidia feels threatened when it responds to competition 😂
Good job AMD -
JarredWaltonGPU
No, that's bad math on your part. 200% faster is three times as fast, but look at what you just said: "Two times faster would be three times as fast." That's basically saying that 2 equals 3. Or to put it another way:DSzymborski said:Twice as fast is one time faster, not two times faster. Two times faster would be three times as fast.
(Which is one of the reasons why style guides frequently forbid use of the term as it's confusing even when used properly).
What is anything times (multiplied by) two? Double the value, twice as fast, 100% faster.