Much maligned Google Flu Trends service gets an AI reboot — new AI-infused approach appears in research paper
AI hoped to bring big accuracy improvements compared to the prior collective intelligence model.
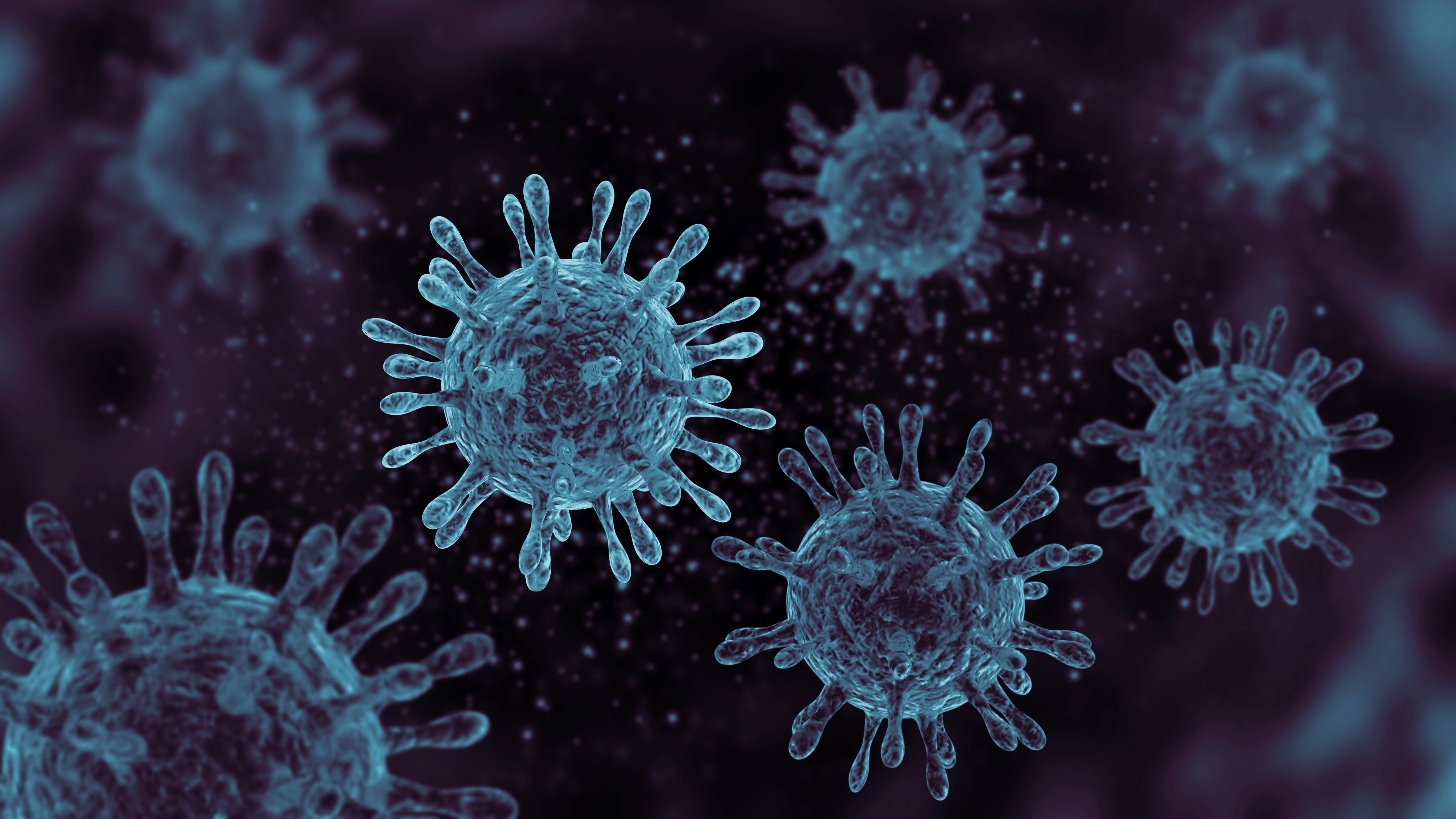
Google may be considering rebooting its much-maligned Google Flu Trends (GFT) service, which died of embarrassment in 2015. First launched in 2008, GFT overestimated, underestimated, and failed to predict several major flu-related events during its seven-year existence. However, Google researchers recently published a paper outlining a new and improved flu rate prediction model that uses modern artificial intelligence (AI) methodology.
To put it mildly, the original GFT service wasn’t a roaring success. Google’s own AI summation (image above) of GFT highlights several studies that found it inaccurate and thus unusable. It failed to predict the 2009 spring pandemic and also “consistently overestimated the relative incidence of flu” in both 2011 and 2013.
GFT launched in 2008 based upon quite a simple and logical premise – people search Google for flu symptoms when they get ill, and the trending flu symptom searches across regions could be used to pre-warn health agencies that a wave of flu infections was likely so precautions/preparations could be implemented. Thus, GFT relied on a kind of Collective Intelligence (CI), which was shoved into a linear model and would be tweaked across the lifetime of the service, but to little worthwhile effect. Hence, Google killed off GFT estimates in August 2015.
The new Google research paper outlines two key techniques that it is hoped will get better results from analyzing and modeling the huge swathes of user data that Google is privy to. These are outlined as follows:
- we introduce SLaM Compression, a way to quantify search terms using pre-trained language models and create a representation of search data that has low dimensionality, is memory efficient, and effectively acts as a summary of search, and
- we present CoSMo, a Constrained Search Model for estimating real-world events using only search data. We demonstrate the efficacy of our contributions by estimating with high accuracy U.S. automobile sales and U.S. flu rates using only Google Search data.
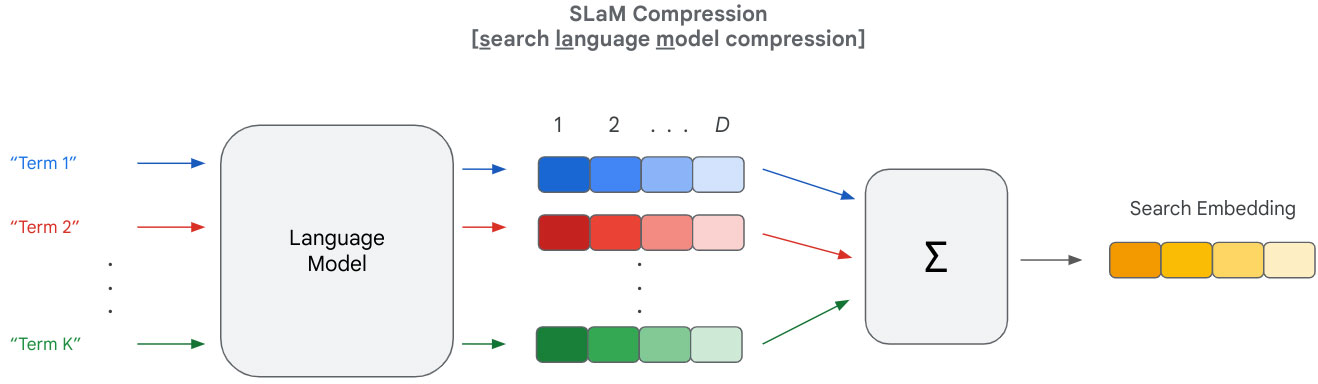
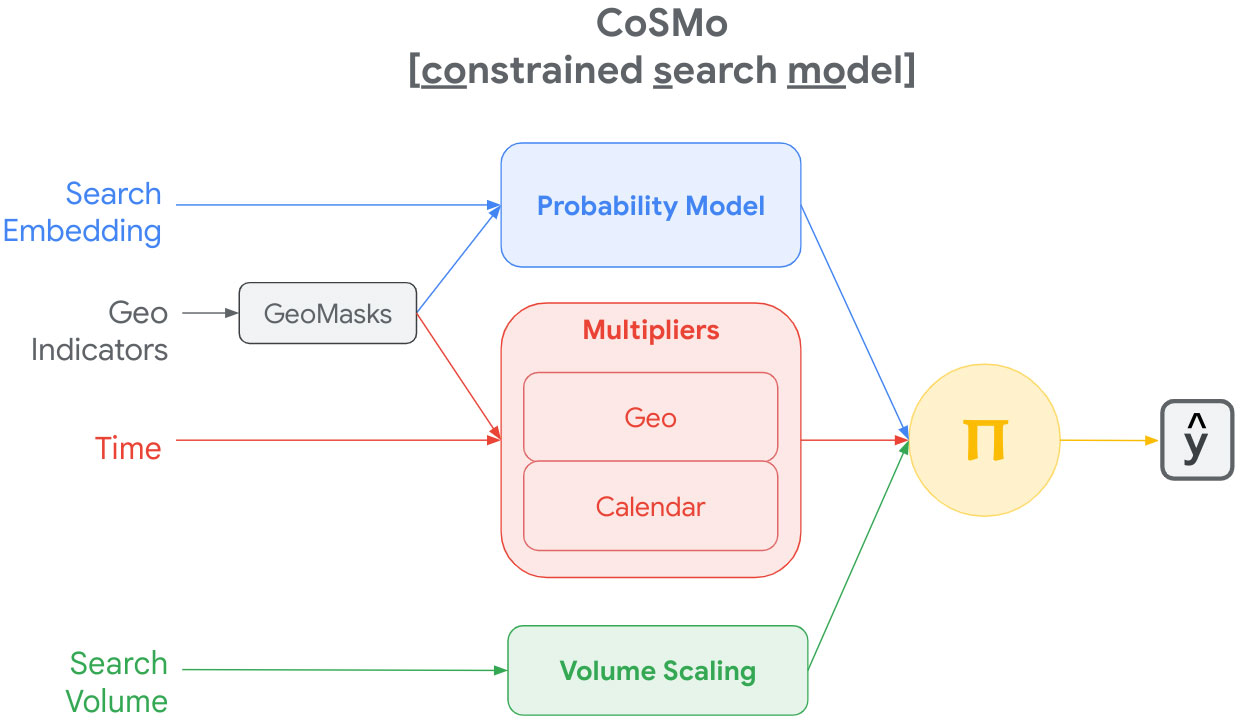
You might have heard of similar tech before, as SLaM (Search Language Model Compression) is used for machine learning tasks and has been especially useful in automotive AI. Meanwhile, CoSMo is a new language model (LM) approach that uses around 512 dimensions to predict real-world events.
We must note that Google also seemed quite confident in its science/methods when it originally launched GFT back in 2008. However, this time, we have new AI-related science and even tighter correlations between what the new model would have predicted and what actually happened in history.
Google seems to have found its new approach to be successful, noting, "We also introduce CoSMo, a constrained search model, which has inductive biases that greatly improve the accuracy of our models built on search data. For estimating the flu rates, we show our simple approach is on par or better than the existing complex ensemble methods. [...] Finally, we demonstrate that our models, despite being highly non-linear neural networks, offer interpretability that explains what terms are related to the variables of interest."
Get Tom's Hardware's best news and in-depth reviews, straight to your inbox.
Whether the heralded new flu rate modeling research leads to Google resurrecting GFT remains to be seen. However, it demonstrates that Google is still interested in perfecting its protection tech, which could be applied to a wide range of potential uses that would eventually make the company yet more money.
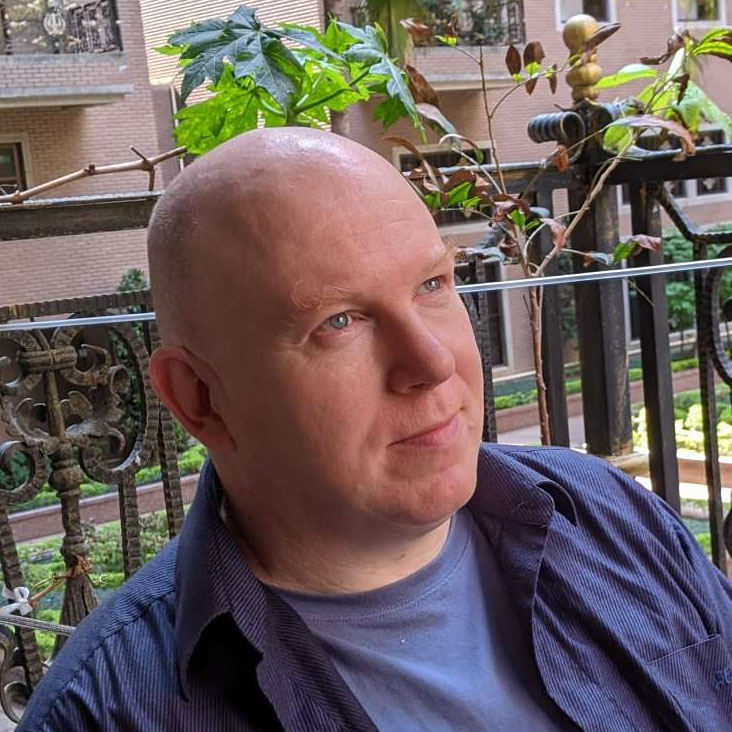
Mark Tyson is a news editor at Tom's Hardware. He enjoys covering the full breadth of PC tech; from business and semiconductor design to products approaching the edge of reason.