Nvidia's H100 GPUs will consume more power than some countries — each GPU consumes 700W of power, 3.5 million are expected to be sold in the coming year
A lot of AI GPUs consume a lot of power.
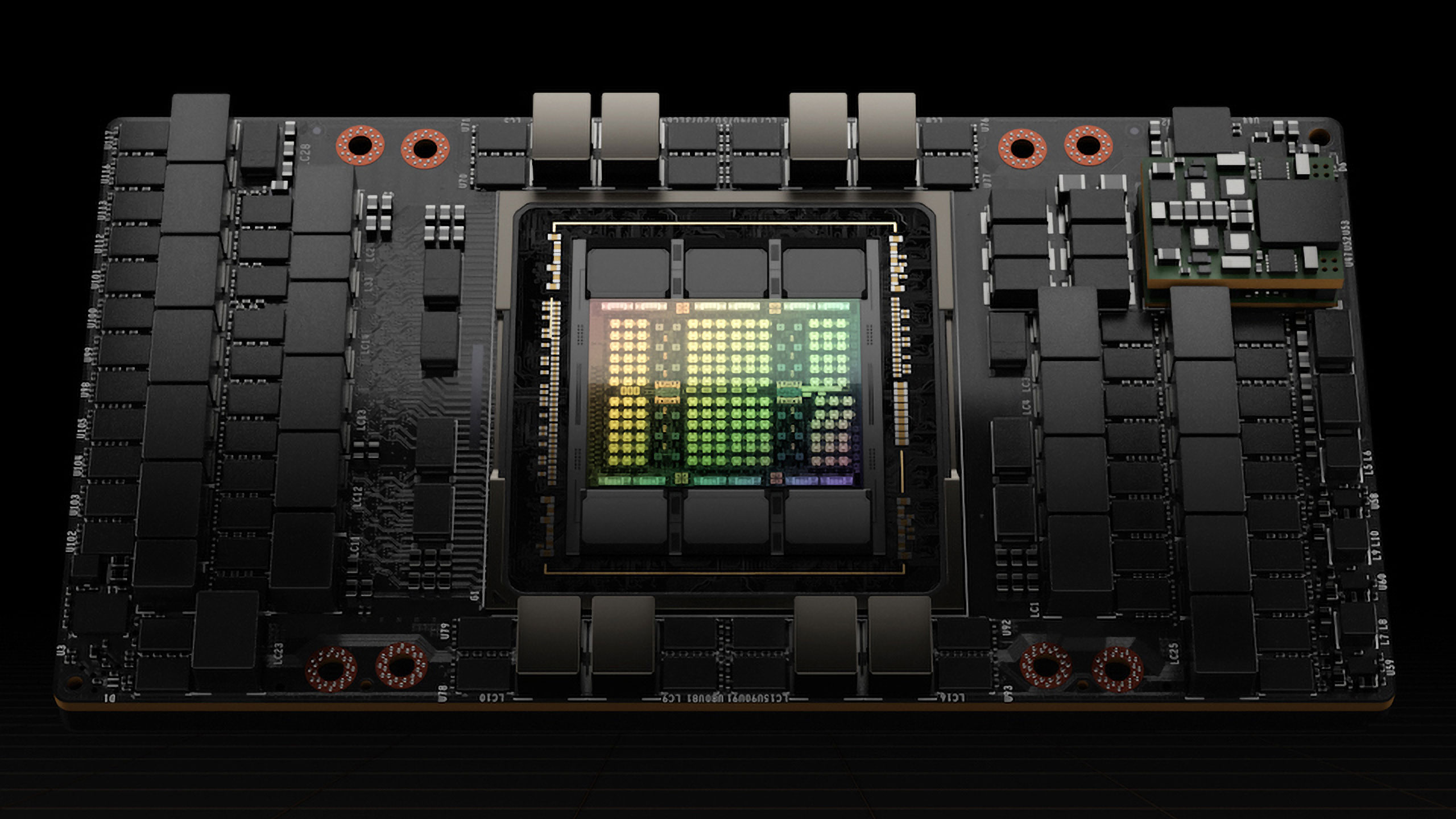
Nvidia literally sells tons of its H100 AI GPUs, and each consumes up to 700W of power, which is more than the average American household. Now that Nvidia is selling its new GPUs in high volumes for AI workloads, the aggregated power consumption of these GPUs is predicted to be as high as that of a major American city. Some quick back-of-the-envelope math also reveals that the GPUs will consume more power than in some small European countries.
The total power consumption of the data centers used for AI applications today is comparable to that of the nation of Cyprus, french firm Schneider Electric estimated back in October. But what about the power consumption of the most popular AI processors — Nvidia's H100 and A100?
Paul Churnock, the Principal Electrical Engineer of Datacenter Technical Governance and Strategy at Microsoft, believes that Nvidia's H100 GPUs will consume more power than all of the households in Phoenix, Arizona, by the end of 2024 when millions of these GPUs are deployed. However, the total power consumption will be less than larger cities, like Houston, Texas.
"This is Nvidia's H100 GPU; it has a peak power consumption of 700W," Churnock wrote in a LinkedIn post. "At a 61% annual utilization, it is equivalent to the power consumption of the average American household occupant (based on 2.51 people/household). Nvidia's estimated sales of H100 GPUs is 1.5 – 2 million H100 GPUs in 2024. Compared to residential power consumption by city, Nvidia's H100 chips would rank as the 5th largest, just behind Houston, Texas, and ahead of Phoenix, Arizona."
Indeed, at 61% annual utilization, an H100 GPU would consume approximately 3,740 kilowatt-hours (kWh) of electricity annually. Assuming that Nvidia sells 1.5 million H100 GPUs in 2023 and two million H100 GPUs in 2024, there will be 3.5 million such processors deployed by late 2024. In total, they will consume a whopping 13,091,820,000 kilowatt-hours (kWh) of electricity per year, or 13,091.82 GWh.
To put the number into context, approximately 13,092 GWh is the annual power consumption of some countries, like Georgia, Lithuania, or Guatemala. While this amount of power consumption appears rather shocking, it should be noted that AI and HPC GPU efficiency is increasing. So, while Nvidia's Blackwell-based B100 will likely outpace the power consumption of H100, it will offer higher performance and, therefore, get more work done for each unit of power consumed.
Stay On the Cutting Edge: Get the Tom's Hardware Newsletter
Get Tom's Hardware's best news and in-depth reviews, straight to your inbox.
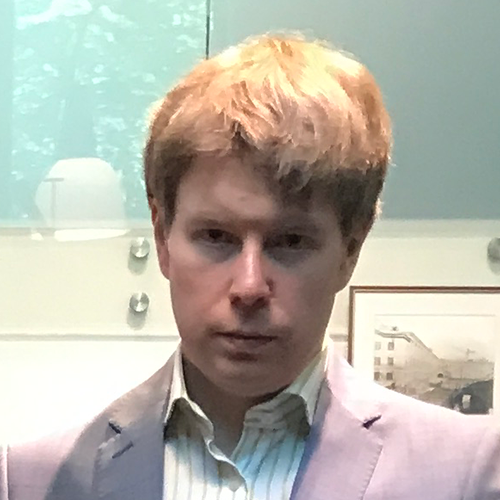
Anton Shilov is a contributing writer at Tom’s Hardware. Over the past couple of decades, he has covered everything from CPUs and GPUs to supercomputers and from modern process technologies and latest fab tools to high-tech industry trends.
-
Colif wow, couldn't that headline be made about almost any GPU sold in the last 5 years that uses over 700watts?Reply -
ElisDTrailz Once again another terribly wrong article from this place. Does Tom's ever check sources? The average American household uses over 30kWh which is WAY more than 700 watts.Reply -
DSzymborski ElisDTrailz said:Once again another terribly wrong article from this place. Does Tom's ever check sources? The average American household uses over 30kWh which is WAY more than 700 watts.
That's 30,000 watt-hours per day. The comparison with the GPU would be the theoretical of 16,800 watt-hours per day. The article exaggerated, but this also compared apples to hand grenades. -
Conor Stewart
It seems you don't know what you are talking about either. Did you really say 30 kWh is more than 700 W? The units aren't even the same, you can't compare them. kWh is a measure of how much energy is used, W is a measure of power.ElisDTrailz said:Once again another terribly wrong article from this place. Does Tom's ever check sources? The average American household uses over 30kWh which is WAY more than 700 watts.
This is the actual quote in the article, "At a 61% annual utilization, it is equivalent to the power consumption of the average American household occupant (based on 2.51 people/household).", so it isn't comparing it to full households, but to individual people and how much energy they use.
30 kWh divided by 2.51 people is 11.95 kWh and that figure is per day anyway. 700 W at 61 % utilisation for a day uses 10.24 kWh or at full utilisation uses 16.8 kWh.
So whilst a few statements in the article may be wrong the quotes they use and the general idea are correct. -
jp7189
Not to be nit picky, but the article states 61% utilization, so that's more like 10kwh per day per H100 or 1/3 that of the average American household. That's a far cry from the assertion that H100 uses more than the average American household.DSzymborski said:That's 30,000 watt-hours per day. The comparison with the GPU would be the theoretical of 16,800 watt-hours per day. The article exaggerated, but this also compared apples to hand grenades. -
jp7189
The article source (which you're quoting) is accurate. Tom's summary is not. That's the point trying to be made. Here a direct quote from the very first line: "...each consumes up to 700W of power, which is more than the average American household."Conor Stewart said:It seems you don't know what you are talking about either. Did you really say 30 kWh is more than 700 W? The units aren't even the same, you can't compare them. kWh is a measure of how much energy is used, W is a measure of power.
This is the actual quote in the article, "At a 61% annual utilization, it is equivalent to the power consumption of the average American household occupant (based on 2.51 people/household).", so it isn't comparing it to full households, but to individual people and how much energy they use.
30 kWh divided by 2.51 people is 11.95 kWh and that figure is per day anyway. 700 W at 61 % utilisation for a day uses 10.24 kWh or at full utilisation uses 16.8 kWh.
So whilst a few statements in the article may be wrong the quotes they use and the general idea are correct.
Tom's left out "per person". -
DSzymborski jp7189 said:Not to be nit picky, but the article states 61% utilization, so that's more like 10kwh per day per H100 or 1/3 that of the average American household. That's a far cry from the assertion that H100 uses more than the average American household.
Yeah, it's off. I was pointing out that the objection made things even worse, not better. -
H4UnT3R Well... now just count consumption of banks... and compare with this one... or BTC ;-) Love these nonsense articles by some Greta lovers (and Greta supports terroristm).Reply -
HansSchulze Peak power is needed during very intense operations that are compute bound. It is hard to achieve full peak power consumption in most apps unless they are hand tuned. Some workloads like inference are more memory bound, so GPU isn't running as hard, and memory power is a small fraction of the GPU power. Likewise 60% utilization is pulling a rabbit out of a hat, server centers run multiple workloads which could put them up against the wall for 24/7 (efficient) or bursty web traffic (GPT?) which would reduce lifetime due to temperature fluctuations. If the 60% number was what the fraction of peak power, I would believe that - easy to misread or misquote sources.Reply
Server centers like AWS, Azure are designed to find 24/7 workloads for all their expensive GPUs. But the GPUs aren't the most expensive part, as the article correctly alludes to. The larger part of the operating budget is electricity, so the primary goal is to use the most recent chips with the highest perf per watt. So imagine using V100 or K180 class supercomputers instead of H100, it would take similar power and take 10x or 50x longer.
Then, ask yourself what the goal is. Do we need to train 59 different AI models? No. Do we need a way to compile models incrementally? Yes. Pancake more layers of knowledge into them without starting over each time? Yes. Do we need to find ways to simplify their representation? Yes.
Source: I am NV alumni.