Arm Announces Machine Learning Processors For ‘Every Market Segment’
The company also announced new GPU IP for mid-range devices and new display processor IP targeting lower-end devices.
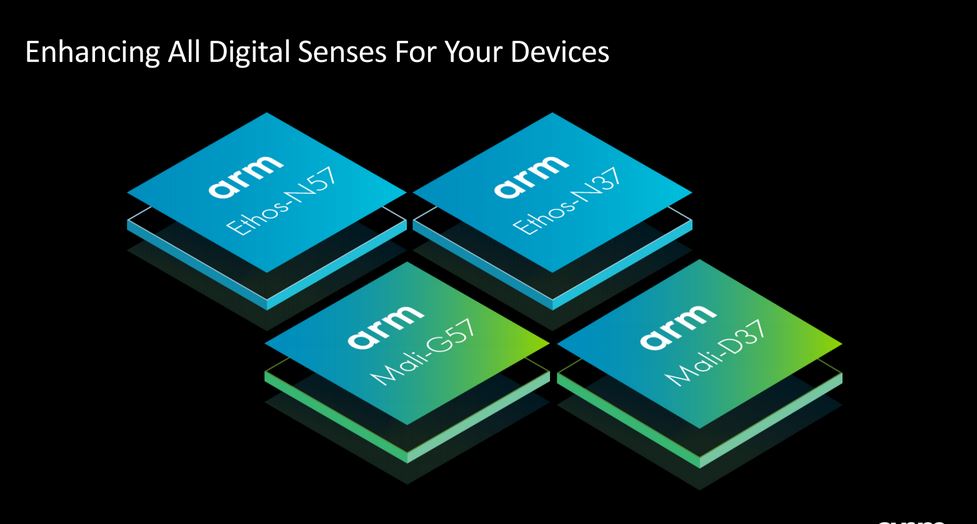
Arm announced the release of new IP for machine learning inference processors that should now cover all market segments from low-budget to the high-end. The company also announced a new high-end GPU IP design based on the Valhall architecture, and a new highly-efficient display processor for smaller devices.
Arm’s new mass market GPU IP, Mali-G57, comes with a 30% performance improvement compared to its predecessor, Mali-G52. The chip is also 30% more efficient, assuming Mali-G52 levels of performance are used. The GPU also supports foveated rendering for VR and 60% better on-device machine learning performance for more complex xR workloads.
The new Mali-D37 is Arm’s most area efficient display processor. It can be configured in an area smaller than 1mm2 using a 16nm process. The processor is optimized for Full HD and 2k resolutions.
Using the Mali-D37 instead of a GPU to drive the display can result in up to 30% system power savings, according to Arm. The new display processor also brings key features from the more premium Mali-D71 display processor, such as mixed HDR and SDR composition when combined with Assertive Display 5 (Arm’s solution for managing lighting on displays).
Arm’s Ethos-N57 and Ethos-N37 neural processing units (NPUs) are dedicated machine learning inference processors meant for mid-range and budget devices. They follow the release of the more premium and higher performance Arm “ML processor,” which has now been rebranded to Ethos-N77.
While the Ethos-N77 ML processor was designed to have a performance level of 4 trillion operations per second (TOPS) and above, the Ethos-N57 will cover the 2 TOPS range, while Ethos-N37 is optimized for the 1 TOPS range.
According to Arm, the Ethos-N57 NPU is meant to provide a balance of performance and power consumption, while the Ethos-N37 NPU is meant to minimize chip die footprint (<1mm2). The performance of these processors can be pushed slightly upwards or downwards, depending on the Arm customers’ needs, as well as the heat dissipation and power consumption capabilities of a particular device.
Get Tom's Hardware's best news and in-depth reviews, straight to your inbox.
The new ML processors are optimized for INT16 and INT8 datatypes and can gain a 200% performance uplift compared to other NPUs through the use of the Winograd algorithm, which can significantly reduce the number of floating point multiplications compared to more conventional techniques.
Over the past few years we’ve seen rapid adoption of machine learning inference processors in smartphones. Compared to CPUs or GPUs, these offer some significant improvements in performance and energy efficiency for certain tasks.
These tasks include: computational photography, voice analysis, smart personalization of certain aspects of your device (like pausing or killing apps that haven’t been used for sometime to save battery life, etc), and anything else that would otherwise have to be analyzed in someone's data center in order to provide you with "intelligent recommendations."
The advantage of having such a chip in your device is that you don’t need to rely on a third-party to analyze the data for you, nor do you need a good internet connection to receive back the results. Your data should also remain private, on your device, as you no longer have to share it for analysis.
As your device doesn’t have to send data back and forth, you can also have a better experience and maintain a good battery life for your device when using an on-device inference chip as opposed to having the data analyzed in the cloud.
Arm now wants to take advantage of this trend by releasing machine learning processor IP for all market segments, so that each device can ship with its own inference processor.