Nvidia's defeatured H20 GPUs sell surprisingly well in China — 50% increase every quarter in sanctions-compliant GPUs for Chinese AI customers
Nvidia rakes it in from China business.
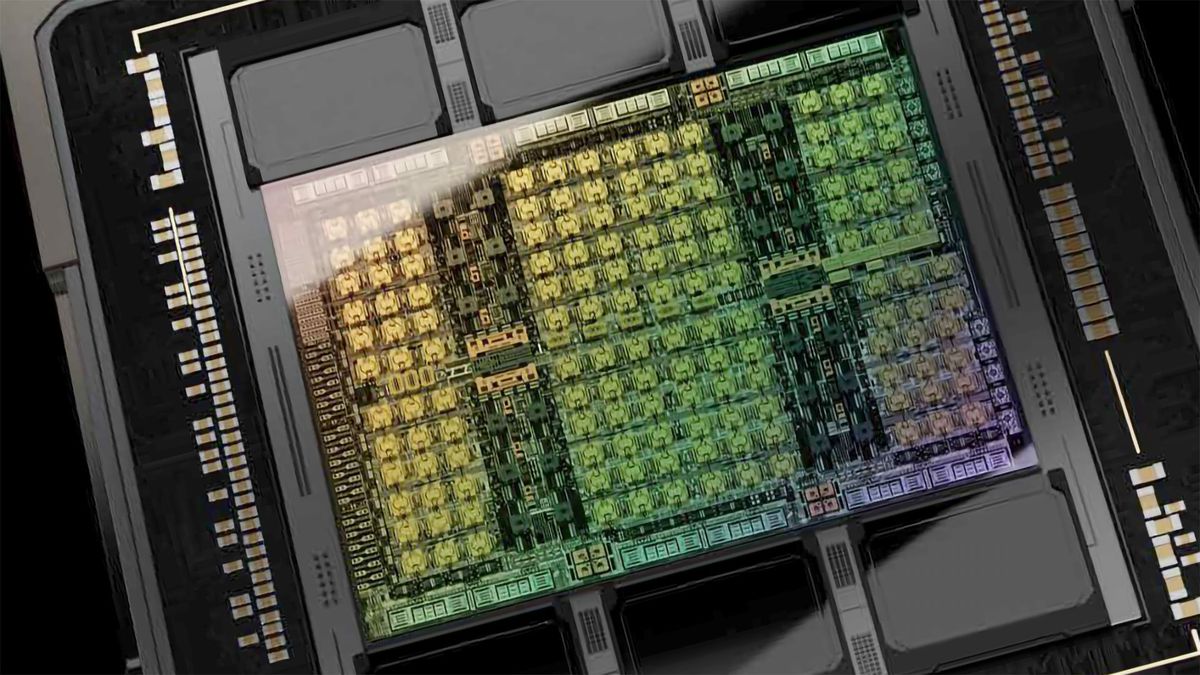
Nvidia's skyrocketing rise in 2023 and 2024 was fueled by the explosive demand for GPUs in the AI sector, mostly in the U.S., Middle-Eastern countries, and China. Since there are U.S. export restrictions and Nvidia cannot sell its highest-end Hopper H100, H200, and H800 processors to China without an export license from the government, it instead sells its cut-down HGX H20 GPUs to entities in China. However, while being cut down, the HGX H20 performs extraordinarily well in terms of sales, according to analyst Claus Aasholm. You can see the product's sales performance in the table embedded in the tweet below.
From @clausaasholm "the downgraded [Nvidia] H20 system that passes the embargo rules for China is doing incredibly well. With 50% growth, quarter over quarter.." pic.twitter.com/YdpvrRrvheDecember 27, 2024
"The downgraded H20 system that passes the embargo rules for China is doing incredibly well," wrote Aasholm. "With 50% growth, quarter over quarter, this is Nvidia's most successful product. The H100 business 'only' grew 25% QoQ."
Based on Claus Aasholm's findings, Nvidia earns tens of billions of dollars selling the HGX H20 GPU despite its seriously reduced performance compared to the fully-fledged H100. Artificial intelligence is indeed a megatrend that drives sales of pretty much all types of data center hardware, including Nvidia's Hopper GPUs, including the HGX H20.
The world's leading economies — the U.S. and China — are racing to gain maximum AI capabilities. For America, the growth is more or less natural: more money and more hardware equal higher capabilities, yet it is not enough. OpenAI alone earns billions, but it needs more to gain more hardware and, therefore, AI training and inference capabilities.
Despite all restrictions, China's AI capabilities — both in hardware and large-model development — are expanding. Just last week, it turned out that Chinese AI company Deepseek revealed in a paper that it had trained its 671-billion-parameter DeepSeek-V3 Mixture-of-Experts (MoE) language model on a cluster of 2,048 Nvidia H800 GPUs and that it took two months, a total of 2.8 million GPU hours. By comparison, Meta invested 11 times the compute resources (30.8 million GPU hours) to train Llama 3, which has 405 billion parameters, using 16,384 H100 GPUs over 54 days.
Over time, China's domestic accelerators from companies like Biren Technologies and Moore Threads might eat into what is now a near-monopoly for Nvidia in Chinese data centers. However, this simply cannot happen overnight.
Stay On the Cutting Edge: Get the Tom's Hardware Newsletter
Get Tom's Hardware's best news and in-depth reviews, straight to your inbox.
Anton Shilov is a contributing writer at Tom’s Hardware. Over the past couple of decades, he has covered everything from CPUs and GPUs to supercomputers and from modern process technologies and latest fab tools to high-tech industry trends.
-
jlake3
Specs are difficult to find, but it appears that H20 is a 78 SM configuration where the H100 comes in 114 SM and 132 SM versions. SMs have been fused off at the die level for many years and not unlockable via vBIOS flashing.Gu3sts said:Are these d"downgraded" at chip level, or at firmware level? -
bit_user One way to look at this news is that the sanctions are working to a significant degree. If it were a practical alternative for them to just buy H100 and H200's, I doubt Nvidia would be selling hardly any of these at all!Reply -
Pemalite In the end, you just add more GPU's to get the same performance.Reply
So the sanctions really aren't changing the landscape much. -
bit_user
Scaling isn't linear. There's a lot of communication involved in training AI models and the more compute nodes you need to exchange data with, the more overhead it adds.Pemalite said:In the end, you just add more GPU's to get the same performance.
So the sanctions really aren't changing the landscape much. -
ottonis Of course it sells well. Just today, there was an article here on tomshardware.com about some guys running a LLM on a Windows 98 machine, powered by a single core Pentium II.Reply
And with regards to training a model: it's much more important how the training data is selected and how the parameters are adjusted than the total time of processing. I don't care if the training lasts 58 days, 89 or 116 days - as long as it delivers great results. -
ottonis
Not sure about that. It all depends on the goal and aim of the sanctions. If the goal was to slightly slow down their efforts of developing large AI models, then yes, they were marginally successful. After all, with a slower/less capable compute unit, you can do everything that you can do with a more capable one, just slower. Heck, they could even pull a "Seti@Home"-like distributed computing approach and still develop everyting they want even without any nvidia card at all.bit_user said:One way to look at this news is that the sanctions are working to a significant degree. If it were a practical alternative for them to just buy H100 and H200's, I doubt Nvidia would be selling hardly any of these at all! -
bit_user
That has nothing to do with anything. First, that was inferencing, not training. The main reason you need lots of powerful GPUs is for training.ottonis said:Of course it sells well. Just today, there was an article here on tomshardware.com about some guys running a LLM on a Windows 98 machine, powered by a single core Pentium II.
Secondly, the quality of that model is too poor for literally anything, which made it just a transparently lame attempt to get some PR. It sort of worked, because they correctly predicted that a lot of people would just read the headline, but for the people who's attention actually could be relevant to their goals, they'll quickly lose interest upon seeing the details. -
bit_user
Everyone seems to focus on the exceptions where sanctioned Chinese users got H100/H200 GPUs anyway. But, with a mass-production item, you're always going to have some slipping through the cracks, so that doesn't necessarily concern me. What concerns me is the overall volume, and these clearly wouldn't be selling if they could really get H100/H200 GPUs very easily.ottonis said:Not sure about that.
No, you can't, for the reason I explained a few posts back. Training a large model requires way too much high-speed communication. An approach like that might take like a decade to train models like the one they did in 2 months.ottonis said:Heck, they could even pull a "Seti@Home"-like distributed computing approach and still develop everyting they want even without any nvidia card at all. -
bit_user
According to this, they did more than just disable SMs. They must've also significantly nerfed functional units within the SMs:jlake3 said:Specs are difficult to find, but it appears that H20 is a 78 SM configuration where the H100 comes in 114 SM and 132 SM versions. SMs have been fused off at the die level for many years and not unlockable via vBIOS flashing.
"When it comes to performance, HGX H20 offers 1 FP64 TFLOPS for HPC (vs. 34 TFLOPS on H100) and 148 FP16/BF16 TFLOPS (vs. 1,979 TFLOPS on H100)."
https://www.tomshardware.com/tech-industry/nvidia-readies-new-ai-and-hpc-gpus-for-china-market-report
Depending on whether the specs were referencing sparse or dense tensors, the half-precision tensor performance got cut to just 7.5% or 15% of a H100. The capability they left notably intact was NVLink.