Nvidia Uses GPU-Powered AI to Design Its Newest GPUs
Was AI instrumental to the advancements behind Ada Lovelace?
Nvidia's chief scientist recently talked about how his R&D teams are using GPUs to accelerate and improve the design of new GPUs. Four complex and traditionally slow processes have already been tuned by leveraging machine learning (ML) and artificial intelligence (AI) techniques. In one example, using AI/ML accelerated inference can speed a common iterative GPU design task from three hours to three seconds.
Bill Dally is chief scientist and SVP of research at Nvidia. HPC Wire has put together a condensed version of a talk Dally shared at the recent GTC conference, in which he discusses the development and use of AI tools to improve and speed up GPU design. Dally oversees approximately 300 people, and these clever folk generally work in the research groups set out below.
In his talk, Dally outlined four significant areas of GPU design where AI/ML can be leveraged to great effect: mapping voltage drop, predicting parasitics, place and routing challenges, and automating standard cell migration. Let's have a look at each process, and how AI tools are helping Nvidia R&D get on with the brain work instead of waiting around for computers to do their thing.
Mapping voltage drop shows designers where power is being used in new GPU designs. Using a conventional CAD tool will help you calculate these figures in about three hours, says Dally. However, once trained, Nvidia's AI tool can cut this process down to three seconds. Such a reduction in processing time helps a lot with a process like this, which is iterative in nature. The process, as it stands, offers 94% accuracy, which is the tradeoff for the huge iterative speed increase.
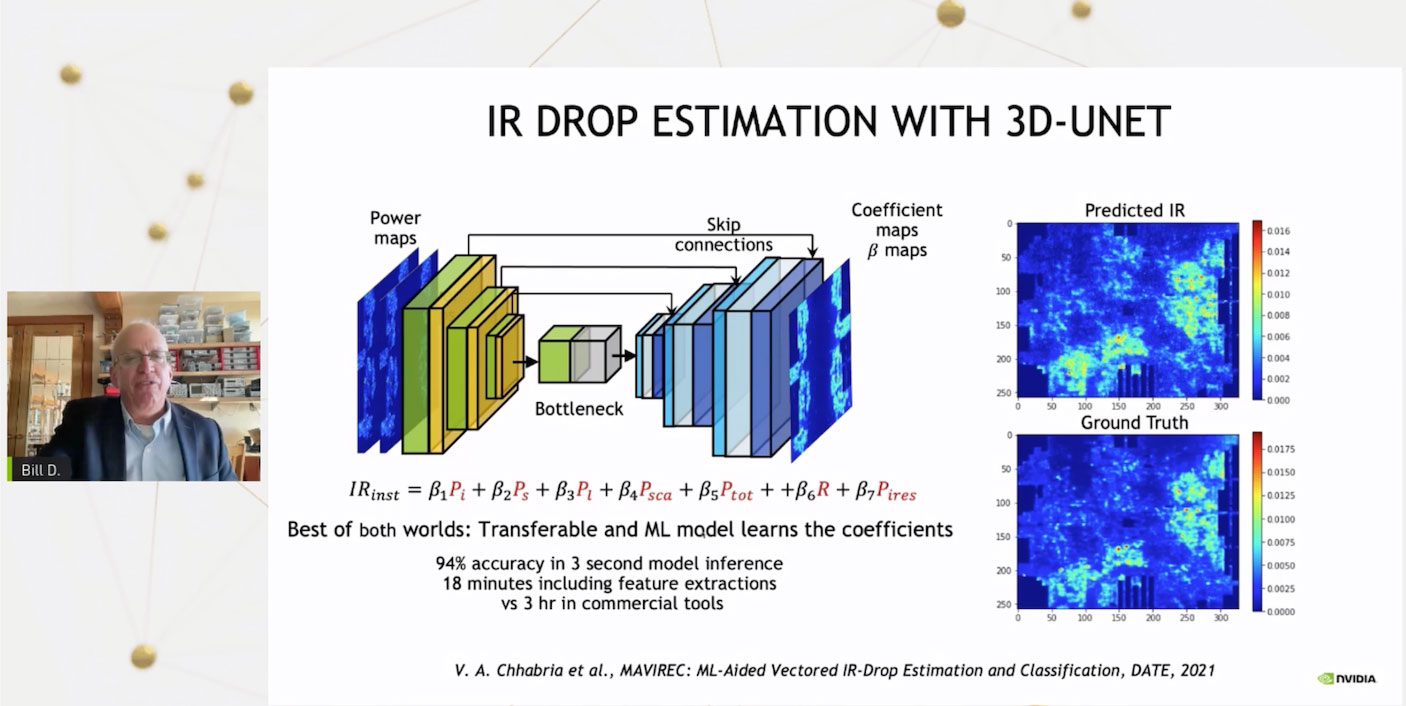
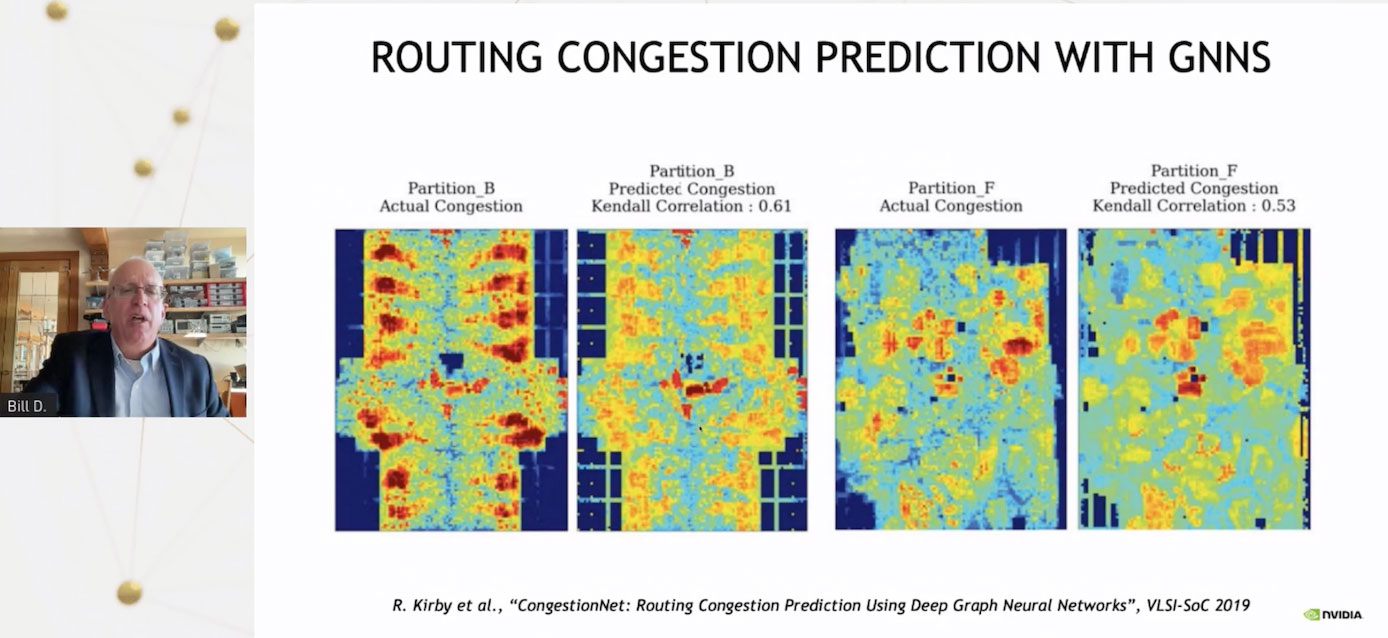
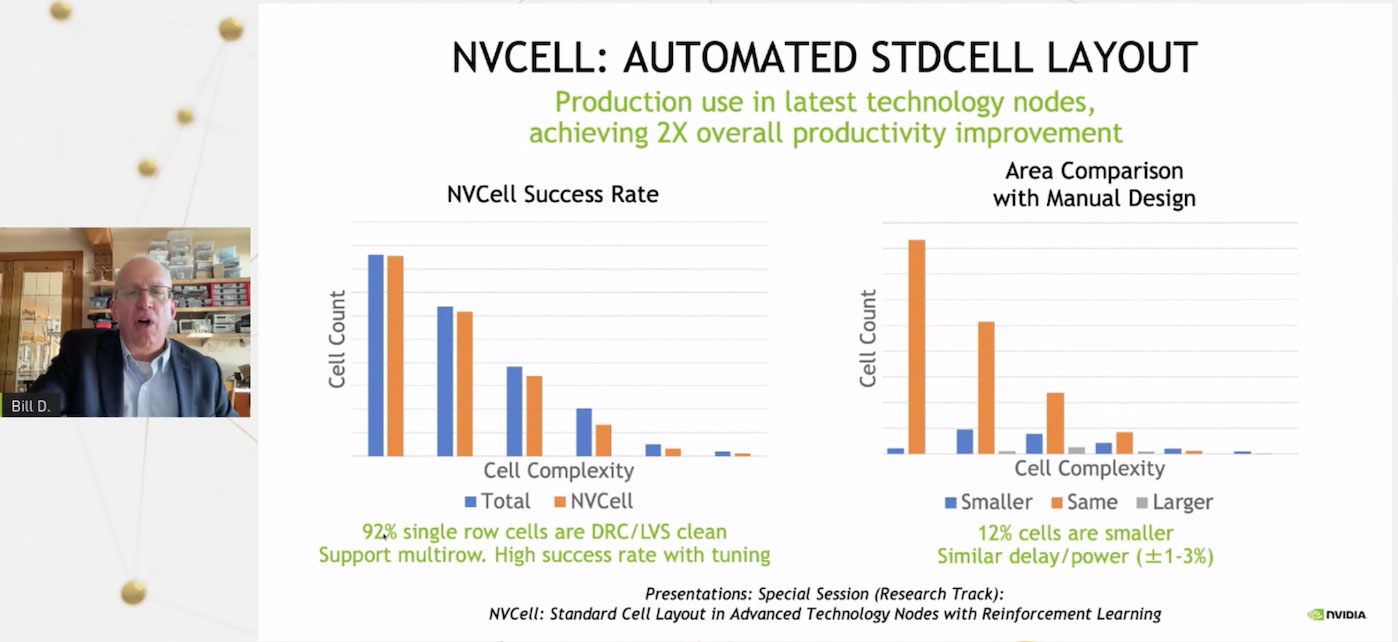
Predicting parasitics using AI is particularly pleasing for Dally. He says that he spent quite some time as a circuit designer and this new AI model cuts down a lengthy multi-personnel, multi-skilled process. Again the simulation error is reasonably low, at <10% in this case. Cutting down these traditionally lengthy iterative processes can free up a circuit designer to be more creative or adventurous.
Place and routing challenges are important to chip design as they are like planning roads through a busy conurbation. Getting this wrong will result in traffic (data) jams, requiring rerouting or replanning of layouts for efficiency. Using Graph Neural Networks (GNNs) to analyze this issue in chip design helps highlight areas of concern and act on issues intelligently.
Lastly, automating standard cell migration using AI is another very useful tool in Nvidia's chip design toolbox. Dally talks about the great effort previously required to migrate a chip design from seven to five nanometer, for example. Using reinforcement learning AIs, "92% of the cell library was able to be done by this tool with no design rule or electrical rule errors," he says. This is welcome for its huge labor savings "and in many cases, we wind up with a better design as well," continues Dally.
Last year at GTC, Dally's talk stressed the importance of prioritizing AI and told of the five separate Nvidia labs indulging in AI research projects. We can't wait to see and hear about whether Nvidia's homegrown AI tools have been important to the design of Ada Lovelace GPUs and getting them ready for TSMC 5nm. Dally seems to hint that automating standard cell migration using AI was used in some 7nm to 5nm transition(s) recently.
Get Tom's Hardware's best news and in-depth reviews, straight to your inbox.
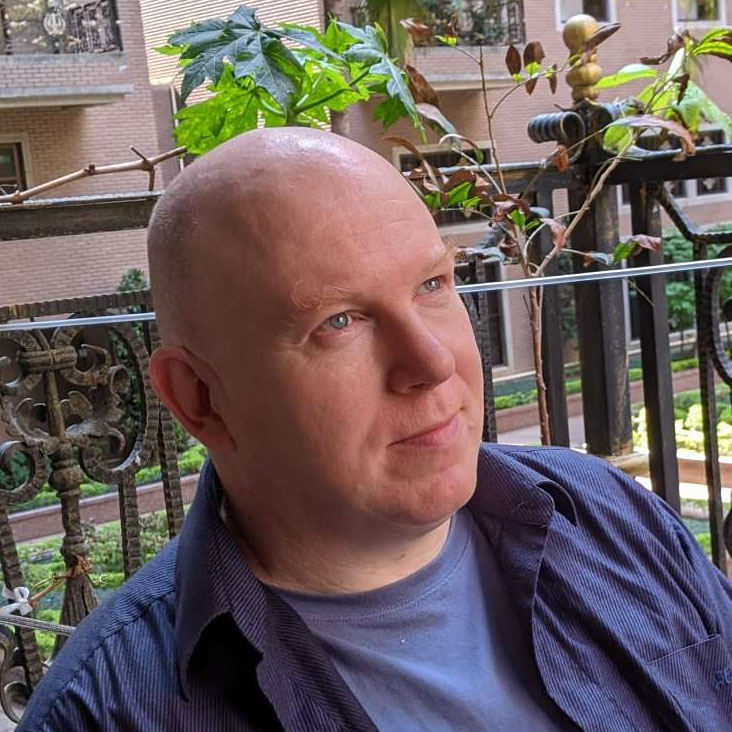
Mark Tyson is a news editor at Tom's Hardware. He enjoys covering the full breadth of PC tech; from business and semiconductor design to products approaching the edge of reason.
-
hotaru.hino
And AI like this has been used for over 20 years in various things. My first encounter with such was a presentation on Blondie24, which was a checkers neural net AI that managed to get within the top 1% of players on Yahoo Games. And supposedly it did very well against the best checkers AI at the time. NASA also used AI at one point to have it design an optimized antenna.DRagor said:No no no please don't do that. The last thing we need now is SkyNet.
Also if anything else, if there's one thing the military has yet to do: it's give robots the actual trigger. I mean sure, AI may be used in the information dissemination process, but an actual human still has to pull the trigger. -
jkflipflop98 hotaru.hino said:I mean sure, AI may be used in the information dissemination process, but an actual human still has to pull the trigger.
Yeah. Until the AI that designs the circuitry in the chip decides that's a weakness and puts in a backdoor for itself. -
edzieba
Oh, AI have had trigger authority for decades. CIWS when armed will engage and fire automatically, but there are also cruise missiles. Whilst those are given engagement areas and sometimes routes/waypoints, once in those areas target identification, classification, prioritisation and engagement are autonomous. The sole difference between a cruise missile operating in this manner and a "killer drone" is there is a more than 0% change to get your drone back again. 'Killer suicide drones' in that sense have been used in anger for decades. The recent sinking of the Moskva with Luch's Neptun is an example: the Neptun will be given a search area within which to find targets (and possibly waypoints to follow beforehand if local air defence is known), but the decision to engage or abort is taken on-board the missile. If there are no targets detected (either due to ECM, or just due to there being nothing there) that's performed without a human in the loop. Likewise, if there are multiple targets (e.g. civilian vessels and a warship) the missile will be performing identification and discrimination without a human in the loop. The venerable Harpoon is rather dumber in this regard, as it's area discrimination is limited to "first thing it sees", but almost any other AShM is more capable.hotaru.hino said:Also if anything else, if there's one thing the military has yet to do: it's give robots the actual trigger. I mean sure, AI may be used in the information dissemination process, but an actual human still has to pull the trigger. -
hotaru.hino
If I wanted to get really technical, someone still has to activate the system. The system doesn't just suddenly activate on its own. Nor is the system powered by AI in the sense that it's using neural network based software, at least as far as I'm aware of.edzieba said:Oh, AI have had trigger authority for decades. CIWS when armed will engage and fire automatically, but there are also cruise missiles. Whilst those are given engagement areas and sometimes routes/waypoints, once in those areas target identification, classification, prioritisation and engagement are autonomous. The sole difference between a cruise missile operating in this manner and a "killer drone" is there is a more than 0% change to get your drone back again. 'Killer suicide drones' in that sense have been used in anger for decades. The recent sinking of the Moskva with Luch's Neptun is an example: the Neptun will be given a search area within which to find targets (and possibly waypoints to follow beforehand if local air defence is known), but the decision to engage or abort is taken on-board the missile. If there are no targets detected (either due to ECM, or just due to there being nothing there) that's performed without a human in the loop. Likewise, if there are multiple targets (e.g. civilian vessels and a warship) the missile will be performing identification and discrimination without a human in the loop. The venerable Harpoon is rather dumber in this regard, as it's area discrimination is limited to "first thing it sees", but almost any other AShM is more capable.
The trigger need not necessarily mean setting off the actual munition. -
edzieba
"Real AI" is a continuous moving target based mostly on what is in common usage and what is new and trendy. "Fuzzy logic" was AI decades ago, but then became not AI because Learning Classifier Systems were the hot new AI thing and Fuzzy Logic was no longer AI but now merely flowcharts with wide tolerances (can't go saying your washing machine used AI with a straight face, so clearly it couldn't be AI anymore). Now, 'Deep Learning' (SLNNs run massively parallel for training because compute is cheap now) is AI, and Learning Classifier Systems are not AI and are merely common-or-garden recursive algorithms. 'Deep Learning' is becoming pretty ubiquitous, so we're due for the next round of a new architecture being 'real' AI, and Deep Learning being relegated to just that boring old non-AI stuff everyone's phones do.hotaru.hino said:If I wanted to get really technical, someone still has to activate the system. The system doesn't just suddenly activate on its own. Nor is the system powered by AI in the sense that it's using neural network based software, at least as far as I'm aware of.
The trigger need not necessarily mean setting off the actual munition.