Generative AI Goes 'MAD' When Trained on AI-Created Data Over Five Times
Generative AI goes "MAD" after five training iterations on artificial outputs.
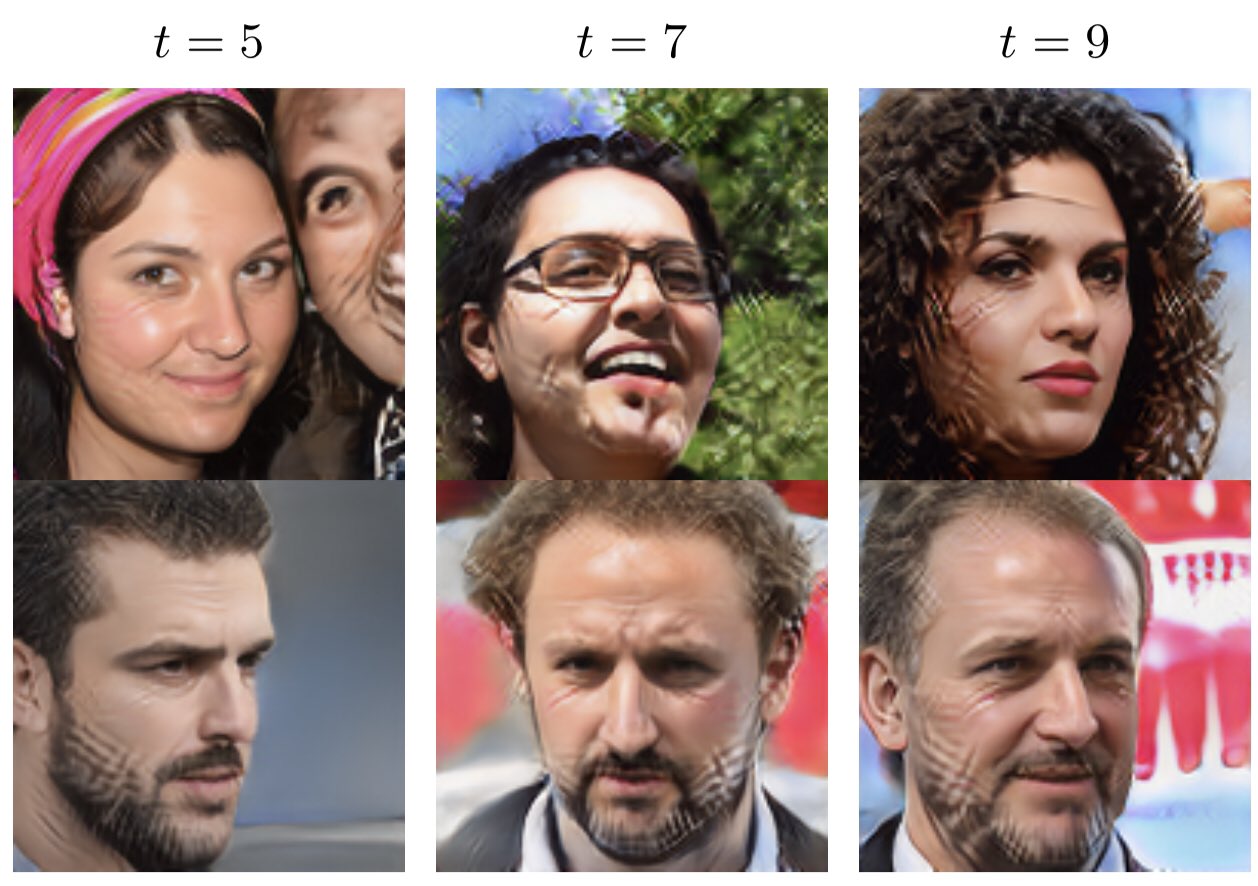
A new study on AI has found an inherent limitation on current-generation networks such as the ones employed by ChatGPT and Midjourney. It seems that AI networks trained on AI outputs (like the text created by ChatGPT or the image output created by a Stable Diffusion model) tend to go "MAD" after five training cycles with AI-generated data. As you can see in the above images, the result is oddly mutated outputs that aren't reflective of reality.
MAD - short for Model Autophagy Disorder — is the acronym used by the Rice and Stanford University researchers involved in the study to describe how AI models, and their output quality, collapses when repeatedly trained on AI-generated data. As the name implies, the model essentially "eats itself," not unlike the Ouroboros of myth. It loses information on the tails (the extremes) of the original data distribution, and starts outputting results that are more aligned with the mean representation of data, much like the snake devouring its own tail.
In work led by @iliaishacked we ask what happens as we train new generative models on data that is in part generated by previous models.We show that generative models lose information about the true distribution, with the model collapsing to the mean representation of data pic.twitter.com/OFJDZ4QofZJune 1, 2023
In essence, training an LLM on its own (or anothers') outputs creates a convergence effect on the data that composes the LLM itself. This can be easily seen in the graph above, shared by scientists and research team member Nicolas Papernot on Twitter, where successive training iterations on LLM-generated data leads the model to gradually (yet dramatically) lose access to the data contained at the extremities of the Bell curve - the outliers, the less common elements.
The data at the edges of the spectrum (that which has fewer variations and is less represented) essentially disappears. Because of that, the data that remains in the model is now less varied and regresses towards the mean. According to the results, it takes around five of these rounds until the tails of the original distribution disappear - that's the moment MAD sets in.
Cool paper from my friends at Rice. They look at what happens when you train generative models on their own outputs…over and over again. Image models survive 5 iterations before weird stuff happens.https://t.co/JWPyRwhW8oCredit: @SinaAlmd, @imtiazprio, @richbaraniuk pic.twitter.com/KPliZCABd4July 7, 2023
Model Autophagy Disorder isn't confirmed to affect all AI models, but the researchers did verify it against autoencoders, Gaussian mixture models, and large language models.
It just so happens that all of these types of models that can "go MAD" have been widespread and operating for a while now: autoencoders can handle things such as popularity prediction (in things such as a social media app's algorithm), image compression, image denoising, and image generation; and gaussian mixture models are used for density estimation, clustering, and image segmentation purposes, which makes them particularly useful for statistical and data sciences.
As for the large language models at the core of today's popular chatbot applications (of which OpenAI's ChatGPT and Anthropic's friendly AI Claude are mere examples), they, too, are prone to go MAD when trained on their own outputs. With that, it's perhaps worth stressing how important these AI systems are in our lives; algorithmic AI models are employed both in the corporate and public spheres.
We faced a similar issue while bootstrapping generative models for Sokoban level generation using https://t.co/ONWUSMnBTQOne solution was to cluster levels based on their characteristics and to change the batch sampling process to emphasize levels with rarer characteristics.June 6, 2023
This research provides a way to peer into the black box of AI development. And it shreds any hope that we'd found an endless fountain of data from making a hamster wheel out of certain AI models: feeding it data, and then feeding its own data back into it, in order to generate more data that's then fed back again.
This could be an issue for currently-existing models and applications of these models: if a model that's achieved commercial use has, in fact, been trained on its own outputs, then that model has likely regressed toward its mean (remember it takes around five cycles of input-output for that to manifest). And if that model has regressed towards its mean, then it's been biased in some way, shape, or form, as it doesn't consider the data that would naturally be in the minority. Algorithmic bigotry, if you will.
Get Tom's Hardware's best news and in-depth reviews, straight to your inbox.
Another important point pushed forward by the results is the concern of data provenance: it now becomes even more important to be able to separate "original" data from "artificial" data. If you can't identify what data was created by an LLM or a generative image application, you might accidentally include it in training data for your next-generation product.
Unfortunately, that ship has already likely sailed: there's been a non-zero amount of unlabeled data that's been already produced by these types of networks and been incorporated into other systems. Even if we had a snapshot of the entire Internet before the popularity explosion for ChatGPT or Midjourney, there's long been AI-produced data poured daily onto the world wide web. And that's saying nothing of the immense amounts of data they've in the meantime produced.
But even if that ship has sailed, at least now we know. Knowing means that the search for a watermark that identifies AI-generated content (and that's infallible) has now become a much more important - and lucrative - endeavor, and that the responsibility for labeling AI-generated data has now become a much more serious requirement.
Apart from that, though, there are other ways to compensate for these biases. One of the ways is to simply change the model's weightings: if you increase how relevant or how frequent the results at the tails of the distribution are, they will naturally move along the bell curve, closer to the mean. It follows that they would then be much less prone to "pruning" from the self-generative training: the model still loses the data at the edges of the curve, but that data is no longer only there.
But then, how is the weighting decided? In what measure should the weightings be moved? The frequency increased? There's also a responsibility here to understand the effects of model fine-tuning and how those impact the output as well.
For each question that's answered, there are a number of others that jump to the foreground: questions relating to the truth behind the model's answers (where deviations are known as hallucinations); whether or not the model is biased, and where does this bias come from (if from the training data itself or from the weighting process used to create the network, and now we know from the MAD process as well); and of course, what happens when models are trained on their own data. And as we've seen, the results aren't virtuous.
And they couldn't be: people with no access to new experiences wither too, so they become echo chambers of what has come before. And that's exactly the same as saying something along the lines of "when the model is trained on its own outputs, it collapses."
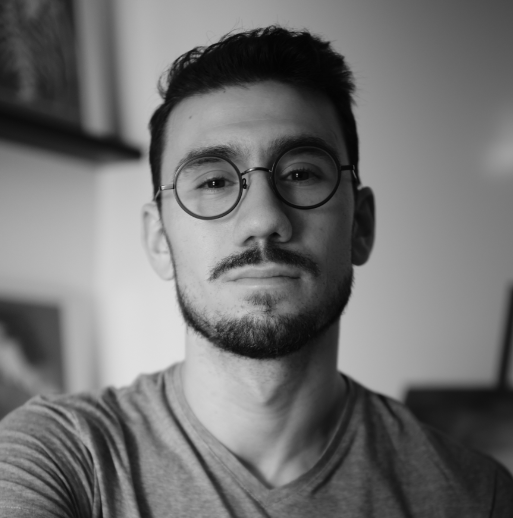
Francisco Pires is a freelance news writer for Tom's Hardware with a soft side for quantum computing.
-
The Historical Fidelity Idk if this is inappropriate for comments so if it is please let me know.Reply
But the first thing that came to mind is that we found out that AI to AI intimate contact can lead to contracting Artificial Syphilis (Syphilis is the STD that makes humans go mad in the brain if left untreated) -
sepuko That means you can poison internet content with image previews and data that appears has the traits of AI generated one so AIs get MAD when crawling. So now AI owners will need to start validating the data the AI trains on. What worries me is that we hold them to no standard when it comes to the selection they feed the AI when training it and that trustworthiness of the output is very dubious, image and code generation aside.Reply -
HonkingAntelope This is by far the number one issue all future LLMs will run into. And that is the lack of "clean" data for training. More so, when you have an arms race, it's very possible for an opponent to introduce subtle poison into the data in order to manipulate the training outcome. Especially if an LLM is being trained essentially by just crawling the internet on a 10Gbps linkReply -
Giroro " ...starts outputting results that are more aligned with the mean representation of data, much like the snake devouring its own tail."Reply
... I never knew that the ouroboros was known for outputting results that align with the mean representation of data. I thought it represented infiinity. -
Giroro The Historical Fidelity said:Idk if this is inappropriate for comments so if it is please let me know.
But the first thing that came to mind is that we found out that AI to AI intimate contact can lead to contracting Artificial Syphilis (Syphilis is the STD that makes humans go mad in the brain if left untreated)
More like inbreeding.
And we are already on *at least* the second generation of AI training AI. The overwhelming majority of online text content has been AI generated for at least a few years. Pretty much since content farms realized the only way to SEO for google listings was to grab "common knowledge" information (which is always unsourced and often incorrect) then repeatedly restate and repackage those bullet points into different ways people might ask full questions into a smart speaker or digital assistant. This destroys the nuance of the information, to the point it quickly loses meaning and eventually becomes wrong and contradictory.
It's why you can't find the info you're looking for on google anymore. It's also why Bard is essentially unusable, and already showing signs of getting worse. -
domih Duh, the scientists at Hollywood predicted the issue back in 1996: https://en.wikipedia.org/wiki/Multiplicity_(film).Reply -
bit_user It's not surprising, really. Your model is only as good as your data.Reply
I do find it interesting that, in the sample images, it seems to have latched onto JPEG-style image compression artifacts and treated them as if they're part of the underlying object.
But, one thing about the article @Francisco Alexandre Pires : the primary focus seems to be on image generators, and yet you seem to conflate them with LLMs:
"In essence, training an LLM on its own (or anothers') outputs creates a convergence effect on the data that composes the LLM itself. "
Yes, the phenomenon was found to apply to LLMs, but let's be clear: image generators aren't LLMs. For instance, Stable Diffusion is a latent diffusion model, as its name implies. The paper actually used an image generator called StyleGAN-2, which is a generative adversarial network.
Please take care not to treat LLM as a short-hand for advanced neural networks, except when it actually applies. -
bit_user
Mad Cow Disease is caused by a specific defective protein (AKA prion), which interferes with protein synthesis in a way that causes yet more of them to be produced. It got propagated through the cattle industry feeding slaughterhouse scraps to other cows. Even cooking the scraps isn't enough to breakdown enough of the prions.Kamen Rider Blade said:So would this be a case of "Mad AI" Disease?
So, in a way, it's not completely off the mark. However, mere cow cannibalism isn't enough. You need to introduce the defective protein into the cycle, at some point.