Nvidia CEO Jensen Huang says $7 trillion isn't needed for AI — cites 1 million-fold improvement in AI performance in the last ten years
Nvidia expects to continue rapidly advancing the performance of its GPUs.
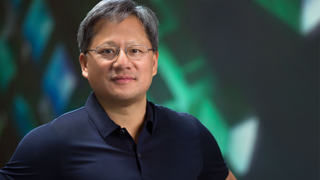
When we first heard that OpenAI's head, Sam Altman, was looking to build a chip venture, we were impressed but considered it as another standard case of a company adopting custom silicon instead of using off-the-shelf processors. However, his reported meetings with potential investors to reportedly raise $5 to $7 trillion to build a network of fabs for AI chips is extreme given that the entire world's semiconductor industry is estimated to be around $1 trillion per year. Nvidia's Jensen Huang doesn't believe that much investment is needed to build an alternative semiconductor supply chain just for AI. Instead, the industry needs to continue its GPU architecture innovations to continue improving performance — in fact, Huang claims that Nvidia has already increased AI performance 1 million fold over the last ten years.
"If you just assume that that computers never get any faster you might come to the conclusion [that] we need 14 different planets and three different galaxies and four more Suns to fuel all this," said Jensen Huang at the World Government Summit.
Investing trillions of dollars to build enough chips for AI data centers can certainly solve the problem of shortages in the course of the next three to five years. However, the head of Nvidia believes that creating an alternative semiconductor supply industry just for AI may not be exactly the best idea since, at some point, it may lead to an oversupply of chips and a major economic crisis. A shortage of AI processors will eventually be solved, partly by architectural innovations, and companies who want to use AI on their premises will not need to build a billion-dollar data center.
"Remember that the performance of the architecture is going to be improving at the same time so you cannot assume just that you will buy more computers," said Huang. "You have to also assume that the computers are going to become faster, and therefore, the total amount that you need is not going to be as much."
Indeed, Nvidia's GPUs evolve very fast when it comes to AI and high-performance computing (HPC) performance. The half-precision compute performance of Nvidia's V100 datacenter GPU was a mere 125 TFLOPS in 2018, but Nvidia's modern H200 provides 1,979 FP16 TFLOPS.
"One of the greatest contributions, and I really appreciate you mentioning that, is the rate of innovation," Huang said. "One of the greatest contributions we made was advancing computing and advancing AI by one million times in the last ten years, and so whatever demand that you think is going to power the world, you have to consider the fact that [computers] are also going to do it one million times faster [in the next ten years]."
Stay on the Cutting Edge
Join the experts who read Tom's Hardware for the inside track on enthusiast PC tech news — and have for over 25 years. We'll send breaking news and in-depth reviews of CPUs, GPUs, AI, maker hardware and more straight to your inbox.
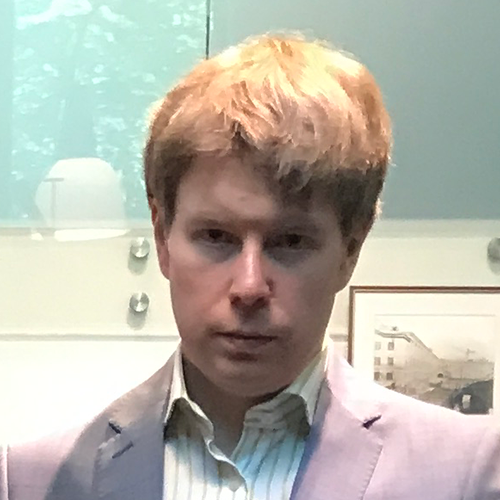
Anton Shilov is a Freelance News Writer at Tom’s Hardware US. Over the past couple of decades, he has covered everything from CPUs and GPUs to supercomputers and from modern process technologies and latest fab tools to high-tech industry trends.
-
digitalgriffin It's only logical Jensen would say this. If Altman's Custom AI Silicon becomes a reality, it's a competitor for NVIDIA which will eat into their profits.Reply
His statement boils down to: "Don't give our competitor money." which is a sign of worry.
Proper reply would have been: "Good luck keeping up with us." -
bit_user It sounds like he forgot that he's not just talking to a bunch of knuckle-dragging gamers, this time.Reply
"Remember that the performance of the architecture is going to be improving at the same time so you cannot assume just that you will buy more computers," said Huang. "You have to also assume that the computers are going to become faster, and therefore, the total amount that you need is not going to be as much."People know this, but they also know that future AI networks will also increase in size & sophistication. So far, the number of parameters has increased faster than the speed of GPUs. Whether that will continue I can't say, but it seems a safe assumption that it will increase and therefore you'll not be training something like a GPT-n model on a single GPU, at any point in the foreseeable future.
"One of the greatest contributions we made was advancing computing and advancing AI by one million times in the last ten years, and so whatever demand that you think is going to power the world, you have to consider the fact that are also going to do it one million times faster ."This is even more unhinged than the last claim! A lot of those multiples came from one-time optimizations, like switching from fp32 to fp8 arithmetic, introducing Tensor Cores, and supporting sparsity. That low-hanging fruit has largely been picked! There aren't a lot of other easy wins, like that.
Furthermore, density and efficiency scaling of new process nodes is slowing down, as are gains in transistors per $. What that means is that manufacturing-driven performance scaling is also mostly exhausted.
So, I don't see any reason to believe the insane pace of AI performance improvements will continue. There will still be gains, but more on the order of 10x or maybe 100x over the next 10 years. Not a million-fold. -
IronyTaken I have my fears of an impending market crash. It is way to top heavy with overspeculation and investors tend to become skittish at a moment's notice. The reality is that the infrastructure is just not there for the hype. The A.I. language models are still using the same neural network method that was being researched decades ago. It's just that we finally have the right pieces coming together to utilize it. The wide availability of data and hardware/software to utilize it has been at play for more than a decade and most of the utility remains the same since then just at a more precise level with less error rates.Reply
The utility hasn't changed much and it's rather limited given the hype and investments going in.
Just like with the dot com boom, the utility just wasn't mature enough at the time. About a decade later and so much changed with sites like YouTube and Facebook.
LLMs definitely have potential but I don't think it warrants this type of hype yet. The shovel sellers are making the majority of the profits. Companies like Nvidia or those who build data centers. Most companies have no idea how to properly utilize it and there is a severe lack of people employed who understand A.I. generative language models. -
bit_user
You're talking about how they're trained? The innovation is in their design & implementation, not the training method!IronyTaken said:The A.I. language models are still using the same neural network method that was being researched decades ago.
Also, kinda weird that you focus on LLMs. They're only one thing happening in AI, right now. OpenAI is rumored to have made significant strides in AGI, for one thing...
The utility requires reasonable low error rates, or the output is just useless rubbish.IronyTaken said:most of the utility remains the same since then just at a more precise level with less error rates. -
MatheusNRei
To be perfectly fair, that's just markets in general.IronyTaken said:It is way to top heavy with overspeculation and investors tend to become skittish at a moment's notice.
I don't believe we will face a market crash but I expect investments will taper off when the technology fails to advance as fast as expected as investors tend to expect a relatively quick ROI and don't tend to be much for long-term planning.