AI makes Doom in its own game engine — Google's GameNGen project uses Stable Diffusion to simulate gameplay
Google Research spawns AI game generation.
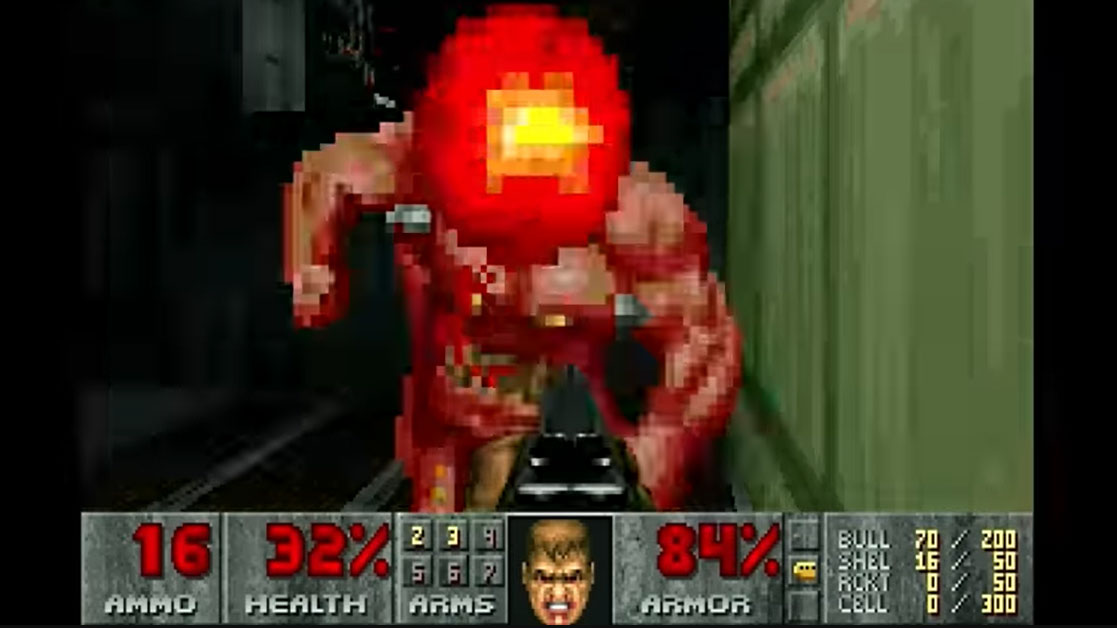
Google Research scientists have released their paper on GameNGen, an AI-based game engine that generates original Doom gameplay on a neural network. Using Stable Diffusion, scientists Dani Valevski, Yaniv Leviathan, Moab Arar, and Shlomi Fruchter designed GameNGen to process its previous frames and the current input from the player to generate new frames in the world with surprising visual fidelity and cohesion.
AI-generating a complete game engine with consistent logic is a unique achievement. GameNGen's Doom can be played like an actual video game, with turning and strafing, firing weapons, and accurate damage from enemies and environmental hazards. An actual level is built around you in real-time as you explore it. It even keeps a mostly precise tally of your pistol's ammo. According to the study, the game runs at 20 FPS and is difficult to distinguish in short clips from actual Doom gameplay.
To obtain all of the training data necessary for GameNGento to accurately model its own Doom levels, the Google team trained its agent AI to play Doom at all difficulties and simulate a range of player skill levels. Actions like collecting power-ups and completing levels were rewarded. At the same time, player damage or death was punished, creating agents that could play Doom and providing hundreds of hours of visual training data for the GameNGen model to reference and recreate.
A significant innovation in the study is how the scientists maintained cohesion between frames while using Stable Diffusion over long periods. Stable Diffusion is a ubiquitous generative AI model that generates images from image or text prompts and has been used for animated projects since its release in 2022.
Stable Diffusion's two most significant weaknesses for animation are its lack of cohesion from frame to frame and its eventual regression in visual fidelity over time. As seen in Corridor's Anime Rock Paper Scissors short film, Stable Diffusion can create convincing still images but suffers from flickering effects as the model outputs consecutive frames (notice how the shadows seem to jump all across the faces of the actors from frame to frame).
The flickering can be solved by feeding Stable Diffusion its output and training it using the image it created to ensure frames match one another. However, after several hundred frames, the image generation becomes less and less accurate, similar to the effect of photocopying a photocopy many times.
Google Research solved this problem by training new frames with a more extended sequence of user inputs and frames that preceded them—rather than just a single prompt image—and corrupting these context frames using Gaussian noise. Now, a separate but connected neural network fixes its context frames, ensuring a constantly self-correcting image and high levels of visual stability that remain for long periods.
Stay On the Cutting Edge: Get the Tom's Hardware Newsletter
Get Tom's Hardware's best news and in-depth reviews, straight to your inbox.
The examples of GameNGen seen so far are, admittedly, less than perfect. Blobs and blurs pop up on-screen at random times. Dead enemies become blurry mounds after death. Doomguy on the HUD is constantly flickering his eyebrows up and down like he's The Rock on Monday Night Raw. And, of course, the levels generated are inconsistent at best; the embedded YouTube video above ends in a poison pit where Doomguy suddenly stops taking damage at 4% and completely changes its layout after turning around 360 degrees inside it.
While the result is not a winnable video game, GameNGen produces an impressive simulacrum of the Doom we love. Somewhere between tech demos and thought experiments on the future of AI, Google's GameNGen will become a crucial part of future AI game development if the field continues. Paired with Caltech's research on using Minecraft to teach AI models consistent map generation, AI-baked video game engines could be coming to a computer near you sooner than we'd thought.
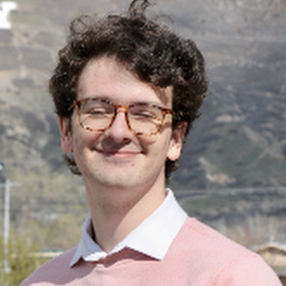
Dallin Grimm is a contributing writer for Tom's Hardware. He has been building and breaking computers since 2017, serving as the resident youngster at Tom's. From APUs to RGB, Dallin has a handle on all the latest tech news.
-
Crazyy8 Finally, the answer to the question I've been asking since the begining, can it run doom?Reply -
Giroro "AI-generating a complete game engine with consistent logic is a unique achievement."Reply
But that's not what they did though, right?
Did they have an AI (presumably a LLM) make a game engine, or did they make an engine that uses Stable Diffusion to guess what the next frame will look like.
... or did they " generate original Doom gameplay on a neural network" - which would be by far the easiest way to pretend like they've accomplished something.
These are 3 different claims that would use 3 very different methods to accomplish, all of which are currently getting lumped together under the AI buzzword.
Based on the video, I'm not sure what they did, but the Doom guy's eyebrow twitches a lot so I doubt it's a level generator. Also they can't seem to get more than a couple seconds of consistent-looking gameplay before they either have to cut to a new clip, or the game teleports the player to a completely different area/state in a way that looks like they cut to a new clip.
But whatever they did, I'm sure they wasted an absolutely unfathomable amount of money and compute time on something that cannot possibly be used to generate revenue for their business. -
bit_user
The game engine is entirely implemented as a neural network. The article says they just feed your mouse & keyboard input into it, and then it renders the image. The neural network model contains all of the game logic, level designs, and is effectively the renderer.Giroro said:"AI-generating a complete game engine with consistent logic is a unique achievement."
But that's not what they did though, right?
Did they have an AI (presumably a LLM) make a game engine, or did they make an engine that uses Stable Diffusion to guess what the next frame will look like.
... or did they " generate original Doom gameplay on a neural network" - which would be by far the easiest way to pretend like they've accomplished something.
As an AI company, doing AI research is part of their business. There's no question that image generators and video generators have real commercial value. If you read the article, it sounds like they made some real advances in addressing the problems with using a Stable Diffusion network to generate video.Giroro said:But whatever they did, I'm sure they wasted an absolutely unfathomable amount of money and compute time on something that cannot possibly be used to generate revenue for their business. -
hotaru251 if you watch the video and look ofr it you can see flaws (most noticeable is the ammo count keeps going between a 5 and 6 digit as if it cant make up its mind)Reply -
Bikki As a AI practictioner I can say a few things about this. First and foremost it is not a game engine. It is image generate AI that acts on a long window of frames (think of video generation) trained on hours of actual game play video so it can generate doom world. In a sense it is a renderer engine.Reply
Now you can say what is the different? A renderer engine doesnt know about player, enermy, item, stat, line of sight and so on. It shows in the demo video when you see corspes disappere when moving out of frame, bullet count doesnt increase when player pick up, tank doesnt blow up when shot ... This is fundamental limit of this approach. At least they solve the game rendering part. -
bit_user
I disagree with your characterization. The input to a rendering engine is geometry and textures. This is not simply a rendering engine.Bikki said:As a AI practictioner I can say a few things about this. First and foremost it is not a game engine. It is image generate AI that acts on a long window of frames (think of video generation) trained on hours of actual game play video so it can generate doom world. In a sense it is a renderer engine.
Now you can say what is the different? A renderer engine doesnt know about player, enermy, item, stat, line of sight and so on. It shows in the demo video when you see corspes disappere when moving out of frame, bullet count doesnt increase when player pick up, tank doesnt blow up when shot ... This is fundamental limit of this approach. At least they solve the game rendering part.
By contrast, a game engine knows the concept of physics, game rules, how to interpret game levels, AI for the enemies, etc. For a game engine, the input is essentially the player actions and it does pretty much all of the rest (with customizations by the game, as necessary).
They said its input is the game controller actions by the player. The model is doing all the work of a game engine, including the rendering phase. Just because it has flaws in the way it implements some of the game engine logic doesn't mean it's not a game engine - it just means that it's a flawed one.
I guess it would be more proper to say the AI model implemented the game, not just a game engine. The distinction is that a game engine abstracts certain things and provides hooks for game-specific customizations, whereas this is a complete game (again, accepting its various flaws and limitations). -
jp7189 "Google Research solved this problem by training new frames with a more extended sequence of user inputs and frames that preceded them—rather than just a single prompt image—and corrupting these context frames using Gaussian noise. Now, a separate but connected neural network fixes its context frames, ensuring a constantly self-correcting image and high levels of visual stability that remain for long periods."Reply
This was the most interesting part to me. It sounds like they have a new approach that's yielding results. Once this is perfected, it may have profound impact on all types of video generation. -
gg83
Doom can run Doom within DoomCrazyy8 said:Finally, the answer to the question I've been asking since the begining, can it run doom? -
edzieba I could see the 'wobblyness' of this being leant into rather than fixed. Train a NN on your game world, then enter a 'dream/nightmare' sequence where you are navigating a version where everything is slightly off, the map starts to diverge the longer time you spend there, nothing quite looks or works right, etc.Reply -
gg83
Maybe games will be released complete? Without 50gb update.jp7189 said:"Google Research solved this problem by training new frames with a more extended sequence of user inputs and frames that preceded them—rather than just a single prompt image—and corrupting these context frames using Gaussian noise. Now, a separate but connected neural network fixes its context frames, ensuring a constantly self-correcting image and high levels of visual stability that remain for long periods."
This was the most interesting part to me. It sounds like they have a new approach that's yielding results. Once this is perfected, it may have profound impact on all types of video generation.