Nvidia Hopper H200 breaks MLPerf benchmark record with TensorRT — no Blackwell submissions yet, sorry
Nvidia beat its previous MLPerf record with newly optimized software and hardware.
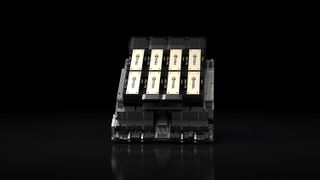
Nvidia reports that its new Hopper H200 AI GPU combined with its performance-enhancing TensorRT LLM has broken the record in the latest MLPerf performance benchmarks. The pairing together has boosted the H200's performance to a whopping 31,712 tokens a second in MLPerf's Llama 2 70B benchmark, a 45% improvement over Nvidia's previous generation H100 Hopper GPU.
Hopper H200 is basically the same silicon as H100, but the memory was upgraded to 24GB 12-Hi stacks of HBM3e. That results in 141GB of memory per GPU with 4.8 TB/s of bandwidth, where the H100 typically only had 80GB per GPU (94GB on certain models) with up to 3 TB/s of bandwidth.
This record will undoubtedly be broken later this year, or early next year, once the upcoming Blackwell B200 GPUs come to market. Nvidia likely has Blackwell in-house and undergoing testing, but it's not publicly available yet. It did claim performance up to 4X higher than H100 for training workloads, however.
Nvidia is the only AI hardware manufacturer in the market that has published full results since MLPerf's data center inference benchmarks became available in late 2020. The latest iteration of MLPerf's new benchmark utilizes Llama 2 70B, is a state-of-the-art language model leveraging 70 billion parameters. Llama 2 is more than 10x larger than GPT-J LLM that was used previously in MLPerf's benchmarks.
MLPerf benchmarks are a suite of benchmarks developed by ML Commons designed to provide unbiased evaluations of training and inference performance for software, hardware, and services. The entire suite of benchmarks consists of many AI neural network designs, including GPT-3, Stable Diffusion V2, and DLRM-DCNv2 to name a few.
Nvidia also highlighted how much it has managed to improve performance of the H100 GPU with its TensorRT software — an open-source suite of tools to help accelerate the processing efficiency of its GPUs. TensortRT is comprised of several elements, including tensor parallelism and in-flight batching. Tensor parallelism uses individual weight matrices to run an AI model efficiently across multiple GPUs and servers. In-flight batching evicts finished sequences of batch requests and begins executing new requests while others are still in flight.
The TensorRT enhancements when applied to the MLPerf GPT-J benchmark resulted in a 3X improvement in performance over the past six months — for the same hardware.
Nvidia also highlighted its performance in MLPerf Llama 2 70B when compared with Intel's Gaudi2 NPU solution. According to Nvidia's charts, the H200 achieved its world record 31,712 scores in server mode with TensorRT-LLM enhancements. In offline mode, the chip scored 29,526 points. The H200's new scores are around 45% faster than what H100 could accomplish, thanks in a large part to having more memory bandwidth and capacity. In the same benchmark, using TensorRT as well, the H100 scored 21,806, and 20,556 in server and offline modes, respectively. Intel's Gaudi2 results by comparison were only 6,287 and 8,035 in server and offline modes.
Beyond TensorRT, Nvidia has integrated several other optimizations into its GPUs. Structured sparsity reportedly adds 33% speedups on inference with Llama 2 by reducing calculations on the GPU. Pruning is another optimization that simplifies an AI model or LLM to increase inference throughput. DeepCache reduces the math required for inference with Stable Diffusion XL models, accelerating performance by 74%.
Below is the full slide deck from Nvidia's MLPerf announcement. You can also read more about some of the TensorRT enhancements going on in Stable Diffusion.
Stay on the Cutting Edge
Join the experts who read Tom's Hardware for the inside track on enthusiast PC tech news — and have for over 25 years. We'll send breaking news and in-depth reviews of CPUs, GPUs, AI, maker hardware and more straight to your inbox.
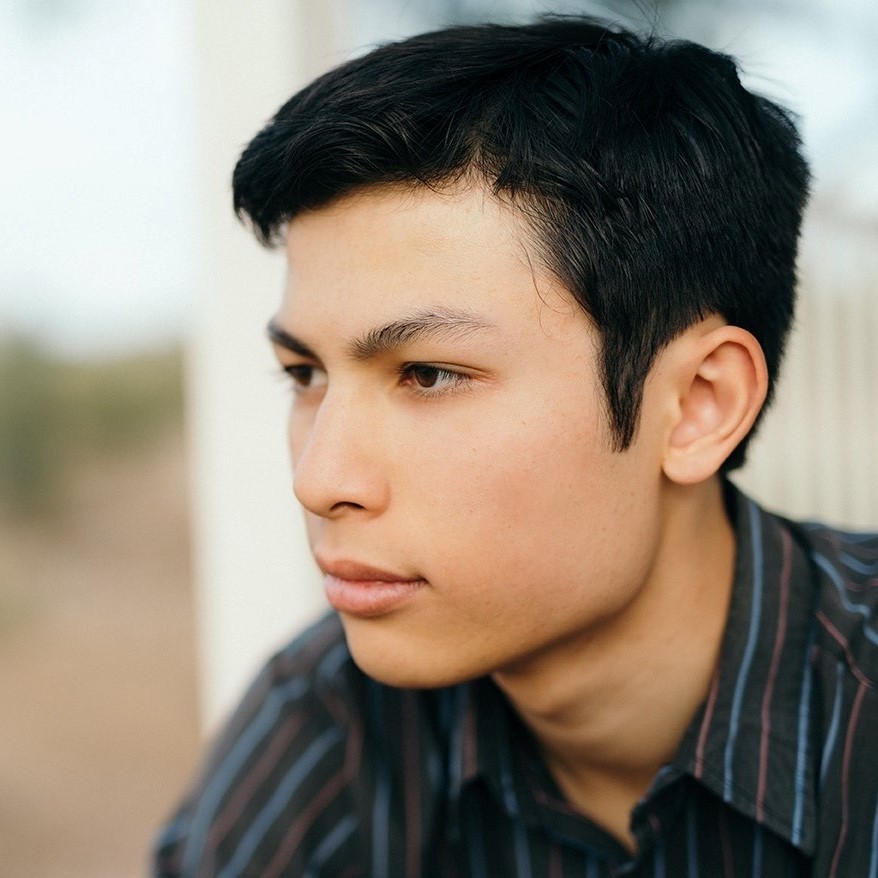
Aaron Klotz is a freelance writer for Tom’s Hardware US, covering news topics related to computer hardware such as CPUs, and graphics cards.