AMD's Future CPUs Could Feature a 3D-Stacked ML Accelerator
Genoa-AI? Bergamo-AI? Turin-AI?
AMD has patented a processor featuring a machine learning (ML) accelerator that is stacked on top of its I/O die (IOD). The patent indicates that AMD may be planning to build special-purpose or datacenter system-on-chips (SoCs) with integrated FPGA or GPU-based machine learning accelerators.
Just like AMD can now add cache to its CPUs, it might add an FPGA or GPU on top of its processor I/O die. But, more importantly, the technology allows the company to add other types of accelerators to future CPU SoCs. As with any patented work, the patent doesn't guarantee that we'll see designs with the tech come to market. However, it gives us a view into what direction the company is moving with its R&D, and there is a chance we could see products based on this tech, or a close derivative, come to market.
Stacking AI/ML Accelerator on Top of an I/O Die
AMD's patent titled 'Direct-connected machine learning accelerator' rather openly describes how AMD might add an ML-accelerator to its CPUs with an IOD using its stacking technologies. Apparently, AMD's technology allows it to add a field-programmable processing array (FPGA) or a compute GPU for machine learning workloads on top of an I/O die with a special accelerator port.
AMD describes several means of adding an accelerator: one involves an accelerator with its own local memory, another implies that such an accelerator uses memory that's connected to an IOD, while in the third scenario, an accelerator could possibly use system memory, and in this case, it does not even have to be stacked on top an IOD.
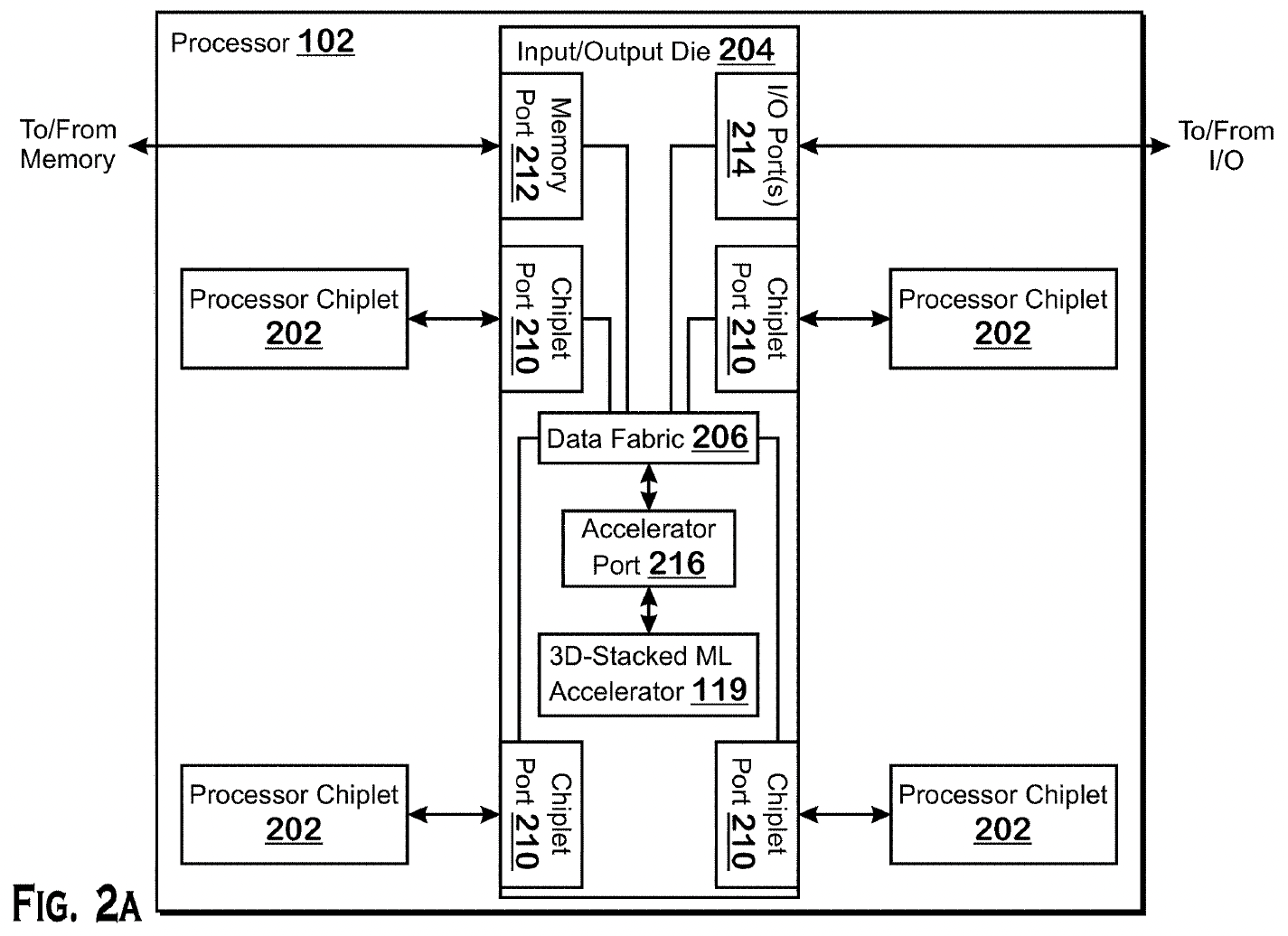
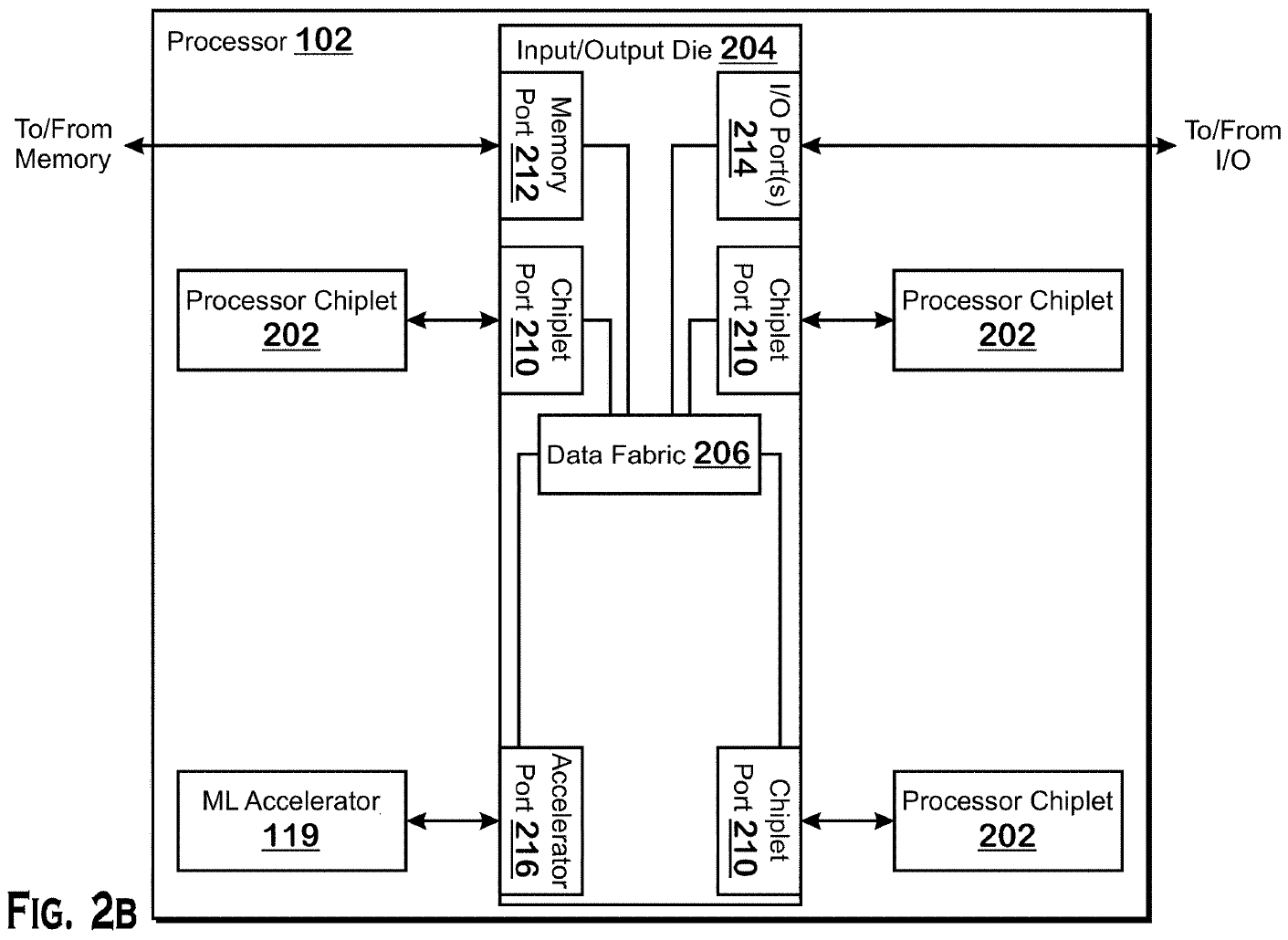
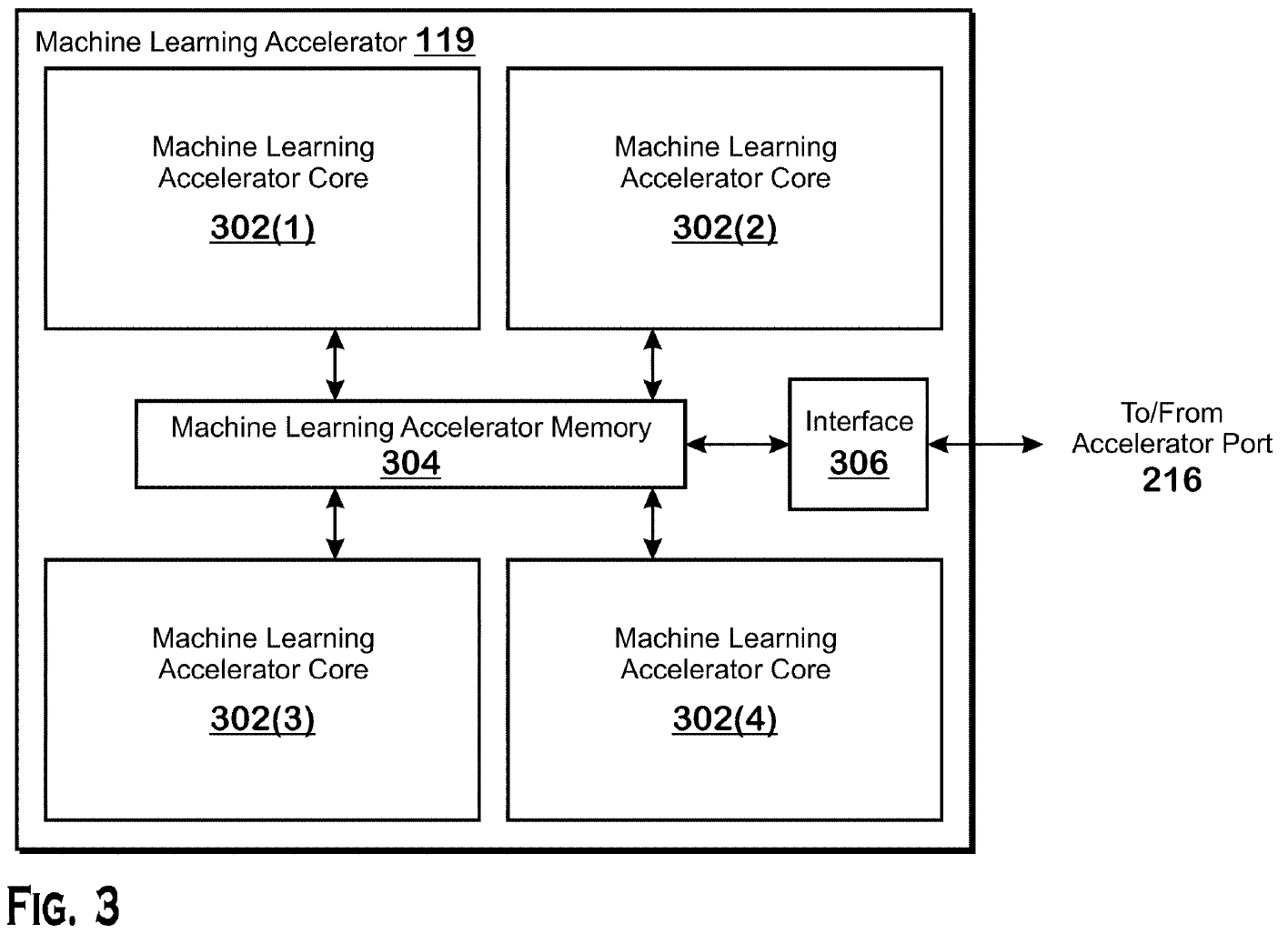
Machine learning techniques will be used extensively by future data centers. However, to be more competitive, AMD needs to accelerate ML workloads using its chips. Stacking a machine-learning accelerator on top of a CPU I/O die allows it to significantly speed up ML workloads without integrating expensive custom ML-optimized silicon into CPU chiplets. It also affords density, power, and data throughput advantages.
The patent was filed on September 25, 2020, a little more than a month before AMD and Xilinx announced that their management teams had reached a definitive agreement under which AMD would acquire Xilinx. The patent was published on March 31, 2022, with AMD fellow Maxim V. Kazakov listed as the inventor. AMD's first products with Xilinx IP are expected in 2023.
We do not know whether AMD will use its patent for real products, but the elegance of adding ML capabilities to almost any CPU makes the idea seem plausible. Assuming that AMD's codenamed EPYC 'Genoa' and 'Bergamo' processors use an I/O die with an accelerator port, there could well be Genoa-AI and Bergamo-AI CPUs with an ML accelerator.
It is also noteworthy that AMD is rumored to be looking at a 600W configurable thermal design power (cTDP) for its 5th Generation EPYC 'Turin' processors, which is more than two times higher than the cTDP of the current-generation EPYC 7003-series 'Milan' processors. Furthermore, AMD's AMD's SP5 platform for 4th Generation and 5th Generation EPYC CPUs provides up to 700W of power for very short periods to processors.
Stay On the Cutting Edge: Get the Tom's Hardware Newsletter
Get Tom's Hardware's best news and in-depth reviews, straight to your inbox.
We do not know how much power AMD's future 96 – 128 (Genoa and Bergamo) CPUs will need, but adding an ML accelerator into the processor package will certainly increase consumption. To that end, it makes great sense to ensure that next-generation server platforms will indeed be capable of supporting CPUs with stacked accelerators.
Building Ultimate Datacenter SoCs
AMD has talked about data center accelerated processing units (APUs) since it acquired ATI Technologies in 2006. Over the last 15 years, we have heard of multiple data center APU projects integrating general-purpose x86 cores for typical workloads and Radeon GPUs for highly parallel workloads.
Neither of these projects has ever materialized, and there are many reasons why. To some degree, because AMD's Bulldozer cores were not competitive, it didn't make a lot of sense to build a large and expensive chip that could be in very limited demand. Another reason is that conventional Radeon GPUs did not support all data formats and instructions required for data center/AI/ML/HPC workloads, and AMD's first compute-centered CDNA-based GPU only emerged in 2020.
But now that AMD has a competitive x86 microarchitecture, a compute-oriented GPU architecture, a portfolio of FPGAs from Xilinx, and a family of programmable processors from Pensando, it may not make much sense to put these diverse IP blocks into a single large chip. Quite the contrary, with today's packaging technologies offered by TSMC and AMD's own Infinity Fabric interconnection technology, it makes much more sense to build multi-tile (or multi-chiplet) modules featuring general-purpose x86 processor chiplets, an I/O die as well as GPU or FPGA-based accelerators.
In fact, it makes more sense to build a multi-chiplet data center processor rather than a large monolithic CPU with built-in diverse IP. For example, a multi-tile datacenter APU could benefit from a CPU tile made using TSMC's N4X performance-optimized node as well as a GPU or FPGA accelerator tile produced using a density-optimized N3E process technology.
Universal Accelerator Port
Another important part of the patent is not a particular implementation designed to accelerate machine learning workloads using an FPGA or a compute GPU, but the principle of adding a special-purpose accelerator to any CPU. The accelerator port will be a universal interface presented on AMD's I/O dies, so eventually, AMD could add other types of accelerators to its processors aimed at client or data center applications.
"It should be understood that many variations are possible based on the disclosure herein," a description of the patent reads. "Suitable processors include, by way of example, a general-purpose processor, a special purpose processor, a conventional processor, a graphics processor, a machine learning processor, [a DSP, an ASIC, an FPGA], and other types of integrated circuit (IC). […] Such processors can be manufactured by configuring a manufacturing process using the results of processed hardware description language (HDL) instructions and other intermediary data including netlists (such instructions capable of being stored on a computer readable media)."
While FPGAs, GPUs, and DSPs could be used for a variety of applications even today, things like data processing units (DPUs) for data centers will only gain importance in the coming years. DPUs are essentially an emerging application that AMD now happens to have. But as the data center transforms to process even more types of data and faster (and so do client PCs, like how Apple integrates application-specific acceleration, like for ProRes RAW, into its client SoCs), accelerators are becoming more common. That means there must be a way to add them to any, or almost any, server processor. Indeed, AMD's accelerator port is a relatively simple way of doing so.
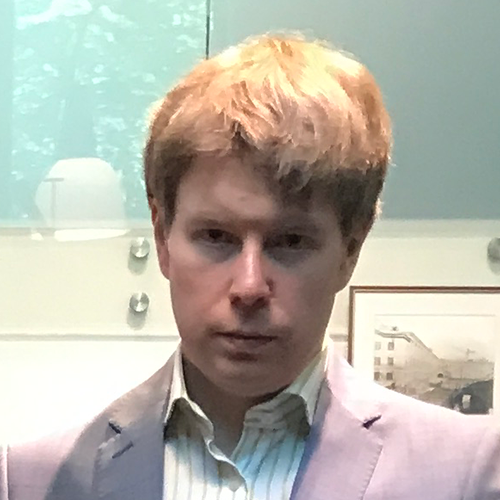
Anton Shilov is a contributing writer at Tom’s Hardware. Over the past couple of decades, he has covered everything from CPUs and GPUs to supercomputers and from modern process technologies and latest fab tools to high-tech industry trends.
-
ezst036 Is there any impact that this could have on long-standing GPU shortages?Reply
Is there a known percentage of users who buy GPUs for ML or neural workloads? (I mean, buy GPUs not for gaming and not for mining) -
TerryLaze
There is but they buy special cards just for that and not gaming GPUs.ezst036 said:Is there any impact that this could have on long-standing GPU shortages?
Is there a known percentage of users who buy GPUs for ML or neural workloads? (I mean, buy GPUs not for gaming and not for mining) -
hotaru.hino
Not really. Anyone who's serious about this sort of work will just get a GPU or some other specialized hardware. It's like how AVX didn't really impact the GPGPU market, despite AVX targeting the same sorts of workloads.ezst036 said:Is there any impact that this could have on long-standing GPU shortages?
Is there a known percentage of users who buy GPUs for ML or neural workloads? (I mean, buy GPUs not for gaming and not for mining) -
ddcservices ezst036 said:Is there any impact that this could have on long-standing GPU shortages?
Is there a known percentage of users who buy GPUs for ML or neural workloads? (I mean, buy GPUs not for gaming and not for mining)
There is a clear misunderstanding here that is very very common. A video card shortage is not necessarily caused by a GPU shortage. There isn't a lot of transparency in the video card market, but consider:
A video card takes a PCB(printed circuit board), the GPU chip itself, VRAM(video RAM), VRMs(Voltage Regulator Modules), then you have the connectors for both monitors, power, and cooling, and then you have the cooling system itself.
If there is a shortage of VRAM, or VRMs, there will be a shortage of video cards, even if there is a large supply of the actual GPUs from AMD or NVIDIA.
There is a huge amount of talk on web sites about the video card shortages, but people assume the shortages are caused by a lack of GPUs, rather than other factors. If a video card maker has limited supplies, they will prioritize select products, but it could also cause things like these video card makers putting the priority into NVIDIA cards, and short-changing AMD in the process by not making a fair number of AMD based cards.
Going back to what you are thinking, AMD has two primarily lines of GPUs, consumer based(RDNA), and workstation/server(CDNA). The CDNA based products(Radeon Instinct) aren't just using the same design as the consumer products, they are compute focused, while RDNA is gaming focused. I haven't heard about a shortage of Radeon Instinct products, but then again, there isn't much discussion about if there are ANY shortages for those professional tier products.