AMD posts first Instinct MI300X MLPerf benchmark results — roughly in line with Nvidia H100 performance
But only in Llama 2 70B.
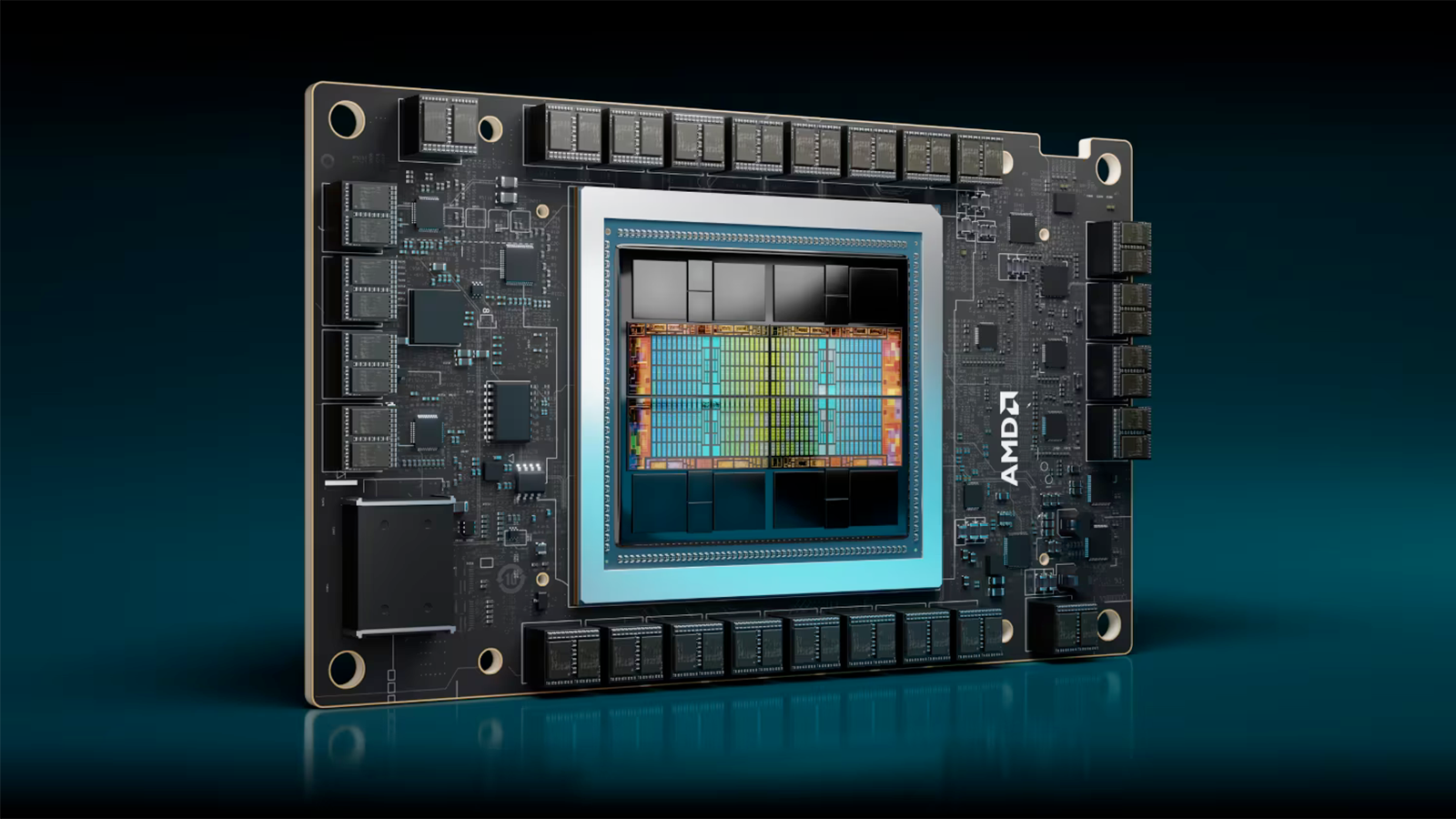
AMD has finally published its first official MLPerf results for the Instinct MI300X accelerator for AI and HPC. The MI300X processor apparently performs in line with Nvidia's previous-generation H100 GPU in the Llama 2 70B model for generative AI, but it falls well behind the revamped H200 version — never mind the upcoming Nvidia B200, which also got its first MLPerf results yesterday.
It's noteworthy that AMD only shared MI300X performance numbers in the MLPerf 4.1 generative AI benchmark on the Llama 2 70B model. Based on the data that AMD shared, a system with eight MI300X processors was only slightly slower (23,512 tokens/second offline) than a system with eight Nvidia H100 SXM3 processors (24,323 tokens/second offline), which can probably be called 'competitive' given how well is Nvidia's software stack is optimized for popular large language models like Llama 2 70B. The AMD MI300X system is also slightly faster than the Nvidia H100 machine in more or less real-world server benchmark: 21,028 tokens/second vs 20,605 tokens/second.
As with Nvidia's B200 results, we need to dig a little deeper to fully dissect these results.
Row 0 - Cell 0 | # of GPUs | Offline | Server | per GPU Offline | per GPU Server |
AMD MI300X 192GB HBM3 | 1 | 3,062 | 2,520 | - | - |
AMD MI300X 192GB HBM3 | 8 | 23,514 | 21,028 | 2,939 | 2,629 |
Nvidia H100 80GB HBM3 | 4 | 10,699 | 9,522 | 2,675 | 2,381 |
Nvidia H100 80GB HBM3 | 8 | 24,323 | 20,605 | 3,040 | 2,576 |
Nvidia H200 141GB HBM3E | 8 | 32,124 | 29,739 | 4,016 | 3,717 |
Nvidia B200 180GB HBM3E | 1 | 11,264 | 10,755 | - | - |
There are two big catches here though. Peak performance of AMD's MI300X for AI is 2.6 POPs (or 5.22 POPs with structured sparsity), while peak performance of Nvidia's H100 is 1.98 FP8/INT8 TFLOPS/TOPS (3.96 TFLOPS/TOPS with sparsity). Also, Nvidia's H100 SXM3 module carries 80GB of HBM3 memory with a peak bandwidth of 3.35 TB/s, while AMD's Instinct MI300X is equipped with 192GB of HBM3 memory with a peak bandwidth of 5.3 TB/s.
That should give AMD's MI300X processor a huge advantage over Nvidia's H100 in terms of performance. Memory capacity and bandwidth play a huge role in generative AI inference workloads and AMD's Instinct MI300X has over twice the capacity and 58% more bandwidth than Nvidia's H100. Yet, the Instinct MI300X can barely win in the server inference benchmark and falls behind H100 in the offline inference benchmark.
It looks as though the MI300X cannot take full advantage of its hardware capabilities, probably because of the software stack. That's probably also why AMD has, until now, avoided showing any MLPerf results. Nvidia is heavily involved with MLPerf and has worked with the benchmark consortium since the early days (MLPerf 0.7 came out in 2020). It's supposed to be an open and agnostic consortium of hardware and software providers, but it can still take time to get decent tuning for any specific AI workload.
That AMD has finally submitted single and 8-way GPU results is promising, and being competitive with Nvidia's H100 is a rather big deal. The MI300X also shows good scaling results, at least up to eight GPUs — though that doesn't necessarily say much about how things scale when looking at potentially tens of thousands of GPUs working in concert for LLM training workloads.
Of course, when it comes to performance comparisons between AMD's MI300X and Nvidia's newer H200, the latter is significantly faster. That's largely thanks to the increase in memory capacity and bandwidth, as the raw compute for H200 hasn't changed from H100. Also, Nvidia's next-generation B200 processor further ups the ante in the MLPerf 4.1 generative AI benchmark on the Llama 2 70B model, but that's a different conversation as the B200 yet has to come to market.
What remains to be seen from AMD's MI300X is a full MLPerf 4.1 submission, for all nine standardized benchmarks. Llama 2 70B is only one of those, with 3D Unet, BERT (Bidirectional Encoder Representations from Transformers), DLRM (Deep Learning Recommendation Model), GPT-J (Generative Pre-trained Transformer-Jumbo), Mixtral, Resnet, Retinanet, and Stable Diffusion XL all being part of the current version. It's not uncommon for a company to only submit results for a subset of these tests (see Nvidia's B200 submission), so we'll have to wait and see what happens in the rest of these inference workload tests.
Get Tom's Hardware's best news and in-depth reviews, straight to your inbox.
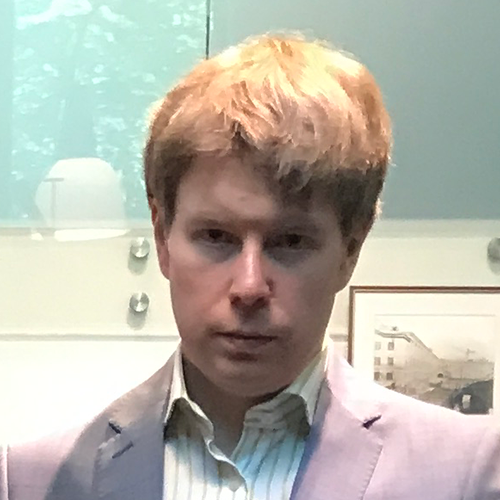
Anton Shilov is a contributing writer at Tom’s Hardware. Over the past couple of decades, he has covered everything from CPUs and GPUs to supercomputers and from modern process technologies and latest fab tools to high-tech industry trends.
-
Bikki AMD clearly has a lot of work to be done for their software stacks if they want to compete. Good article.Reply -
Pierce2623
I mean, realistically, anybody that’s ordered AMD parts already knows they’re significantly behind in the software stack. I’d say the main thing people are looking at if they realistically considering AMD is that the Mi300x is going to be available at like 1/3 of the price of the H100 while including a CPU in the deal, too.Bikki said:AMD clearly has a lot of work to be done for their software stacks if they want to compete. Good article.