MIT's Protonic Resistors Enable Deep Learning to Soar, In Analog
Bringing analog "tunes" to the world of digital chips - with increased performance.
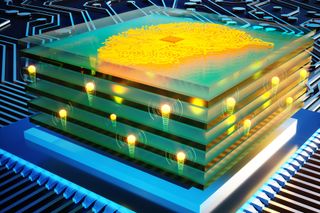
A team of researchers with the Massachusetts Institute of Technology (MIT) have been working on a new hardware resistor design for the next era of electronics scaling - particularly in AI processing tasks such as machine learning and neural networks.
Yet in what may seem like a throwback (if a throwback to the future can exist), their work focuses on a design that's more analog than digital in nature. Enter protonic programmable resistors - built to accelerate AI networks by mimicking our own neurons (and their interconnecting synapses) while accelerating their operation a million times -- and that's the actual figure, not just hyperbole.
All of this is done while cutting down energy consumption to a fraction of what's required by transistor-based designs currently used for machine-learning workloads, such as Cerebras' record-breaking Wafer Scale Engine 2.
While our synapses and neurons are extremely impressive from a computational standpoint, they're limited by their "wetware" medium: water.
While water's electrical conduction is enough for our brains to operate, these electrical signals work through weak potentials: signals of about 100 millivolts propagating over milliseconds, through trees of interconnected neurons (synapses correspond to the junctions through which neurons communicate via electrical signals). One issue is that liquid water decomposes with voltages of 1.23 V - more or less the same operating voltage used by the current best CPUs. So there's a difficulty in simply "repurposing" biological designs for computing.
“The working mechanism of the device is electrochemical insertion of the smallest ion, the proton, into an insulating oxide to modulate its electronic conductivity. Because we are working with very thin devices, we could accelerate the motion of this ion by using a strong electric field, and push these ionic devices to the nanosecond operation regime,” explains senior author Bilge Yildiz, the Breene M. Kerr Professor in the departments of Nuclear Science and Engineering and Materials Science and Engineering.
Another issue is that biological neurons aren't built on the same scale as modern transistors are. They're much bigger - ranging in sizes from 4 microns (.004 mm) to 100 microns (.1 mm) in diameter. When the latest available GPUs already carry transistors at the 6 nm range (with a nanometre being 1,000 times smaller than a micron), you can almost imagine the difference in scale, and how much more of these artificial neurons you can fit into the same space.
Stay On the Cutting Edge: Get the Tom's Hardware Newsletter
Get Tom's Hardware's best news and in-depth reviews, straight to your inbox.
The research focused on creating solid-state resistors which, as the name implies, create resistance to electricity's passage. Namely, they resist the ordered movement of electrons (negatively-charged particles). If using material that resists electricity's movement (and that thus should in turn generate heat) sounds counterintuitive, well, it is. But there are two distinct advantages to analog deep-learning compared to its digital counterpart.
First, in programming resistors, you are including the required data for training in the resistors themselves. When you program their resistance (in this case, by increasing or reducing the number of protons in certain areas of the chip), you're adding values to certain chip structures. This means that information is already present in the analog chips: There's no need to ferry more of it in and out towards external memory banks, which is exactly what happens in most current chip designs (and RAM or VRAM). All of this saves latency and energy.
Second, MIT's analog processors are architected in a matrix (remember Nvidia's Tensor cores?). This means they're more like your GPUs than your CPUs, in that they conduct operations in parallel. All computation happens simultaneously.
MIT's protonic resistor design operates at room temperature, which is easier to achieve than our brain's 38.5 ºC through 40 ºC. Yet it also allows for voltage modulation, a required feature in any modern chip, allowing the input voltage to be increased or decreased according to the requirements of the workload - with consequences on power consumption and temperature output.
According to the researchers, their resistors are a million times faster (again, an actual figure) than previous-generation designs, due to them being built with phosphosilicate glass (PSG), an inorganic material that is (surprise) compatible with silicon manufacturing techniques, because it's mainly silicon dioxide.
You've seen it yourself already: PSG is the powdery desiccant material found in those tiny bags that come in the box with new hardware pieces to remove moisture.
“With that key insight, and the very powerful nanofabrication techniques we have at MIT.nano, we have been able to put these pieces together and demonstrate that these devices are intrinsically very fast and operate with reasonable voltages,” says senior author Jesús A. del Alamo, the Donner Professor in MIT’s Department of Electrical Engineering and Computer Science (EECS). “This work has really put these devices at a point where they now look really promising for future applications.”
Just like with transistors, the more resistors in a smaller area, the higher the compute density. When you reach a certain point, this network can be trained to achieve complex AI tasks like image recognition and natural language processing. And all this is done with reduced power requirements and extremely increased performance.
Perhaps materials research will save Moore's Law from its untimely death.
Francisco Pires is a freelance news writer for Tom's Hardware with a soft side for quantum computing.
-
blargh4 All this research towards human obsolescence, definitely not something we might regret in 50 yearsReply -
bit_user I wonder if the 10^6 improvement is also accounting for the overhead of digital multiplication. If so, I wonder which datatype they used as the basis for comparison (my guess is fp32, but there are far more efficient options supported in recent hardware: bf16, int8, and even fp8 and int4).Reply
I also wonder how well analog neural nets will scale. It seems to me that the more signal propagation you have, the more noise can get amplified. To counteract that, you might need more redundancy, which in turn could impose a practical limit on scalability. And scale is what limits the complexity of "reasoning" it can perform, as well as how much it can "remember".
Analog computing is nothing new, nor are its intrinsic efficiency advantages. Yet, there are reasons why computing went decidedly digital. This makes me a little skeptical of just how far we can get by turning back to analog.
One more thought: I'm no expert, but I thought our neurons are actually sort of digital in amplitude (i.e. being driven by voltage spikes), but operate in the continuous time domain. There's a whole class of digital neural networks which aims to emulate these characteristics, called "spiking neural networks".